Experimental Quantum Principal Component Analysis Via Parametrized Quantum Circuits
PHYSICAL REVIEW LETTERS(2021)
摘要
Principal component analysis (PCA) is a widely applied but rather time-consuming tool in machine learning techniques. In 2014, Lloyd, Mohseni, and Rebentrost proposed a quantum PCA (qPCA) algorithm [Lloyd, Mohseni, and Rebentrost, Nat. Phys. 10, 631 (2014)] that still lacks experimental demonstration due to the experimental challenges in preparing multiple quantum state copies and implementing quantum phase estimations. Here, we propose a new qPCA algorithm using the hybrid classical-quantum control, where parameterized quantum circuits are optimized with simple measurement observables, which significantly reduces the experimental complexity. As one important PCA application, we implement a human face recognition process using the images from the Yale Face Dataset. By training our quantum processor, the eigenface information in the training dataset is encoded into the parameterized quantum circuit, and the quantum processor learns to recognize new face images from the test dataset with high fidelities. Our work paves a new avenue toward the study of qPCA applications in theory and experiment.
更多查看译文
关键词
quantum,circuits
AI 理解论文
溯源树
样例
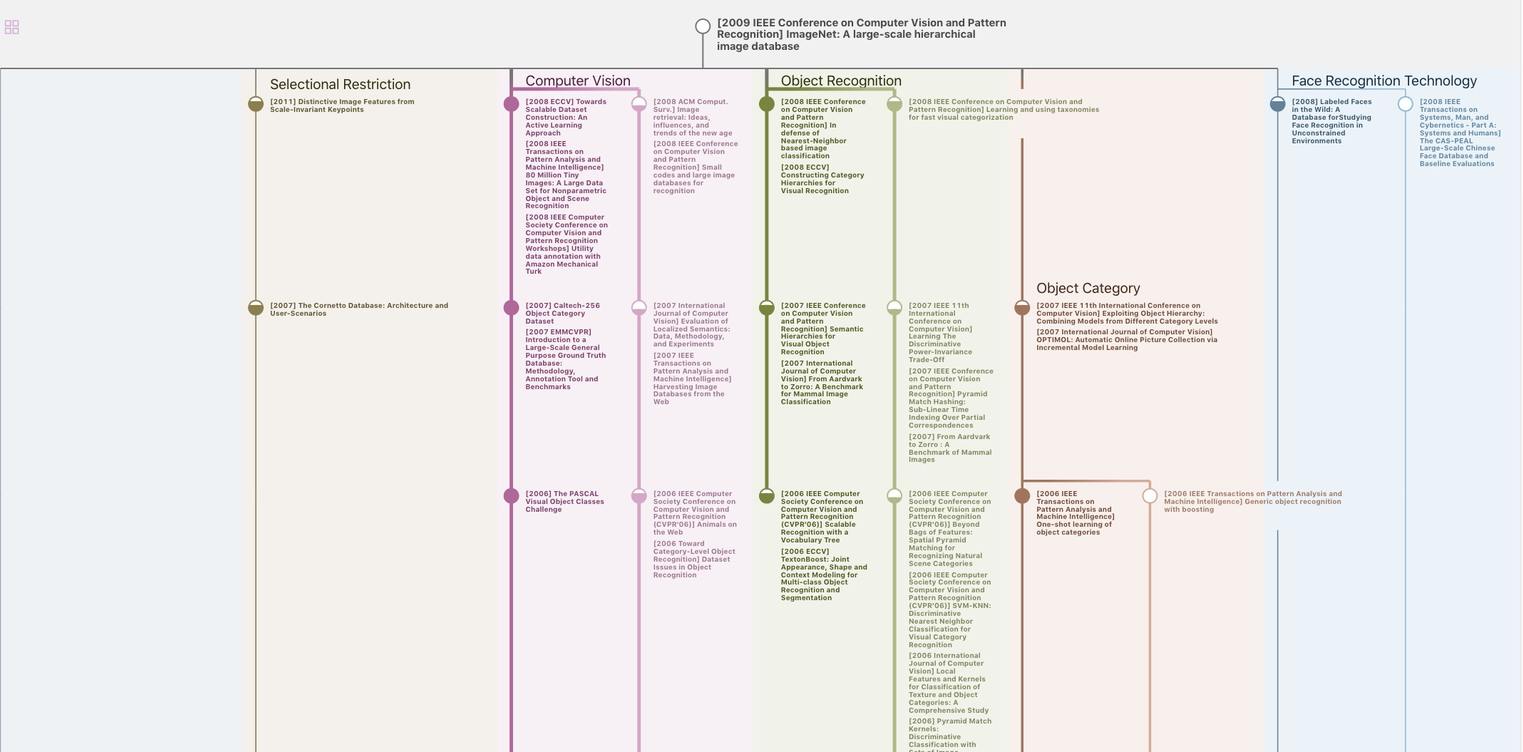
生成溯源树,研究论文发展脉络
Chat Paper
正在生成论文摘要