Short Term Load Forecasting Based on BFA-DRESN Algorithm
2021 IEEE 2nd International Conference on Big Data, Artificial Intelligence and Internet of Things Engineering (ICBAIE)(2021)
摘要
With the in-depth development of Internet of things technology, a large number of Internet of things sensing devices are deployed in various environments of power grid. Smart meters can continuously obtain users’ power consumption parameters according to a certain frequency, and transmit them to power companies through Internet of things communication technology. In the existing smart grid architecture, the power grid system produces a large amount of related data. Based on these data, more useful information can be mined to improve the accuracy of load forecasting. However, load forecasting is affected by many complex factors, weather, date type, user behavior mode and so on. Most of the existing time series forecasting methods have some limitations when applied to power load forecasting. Aiming at the problem that the structure of traditional ESN reserve pool affects the forecasting performance; this paper adopts the echo state network with double reserve pools. According to the demand of load forecasting, the depth neural network intelligent load forecasting model is constructed by using the historical load data and multi-dimensional information such as environment, and the parameters of the echo state network with double reserve pools are optimized by the bacterial foraging algorithm. The simulation results show that the proposed BFA-DRESN can effectively improve the prediction accuracy.
更多查看译文
关键词
Microorganisms,Load forecasting,Predictive models,Prediction algorithms,Internet of Things,Forecasting,Load modeling
AI 理解论文
溯源树
样例
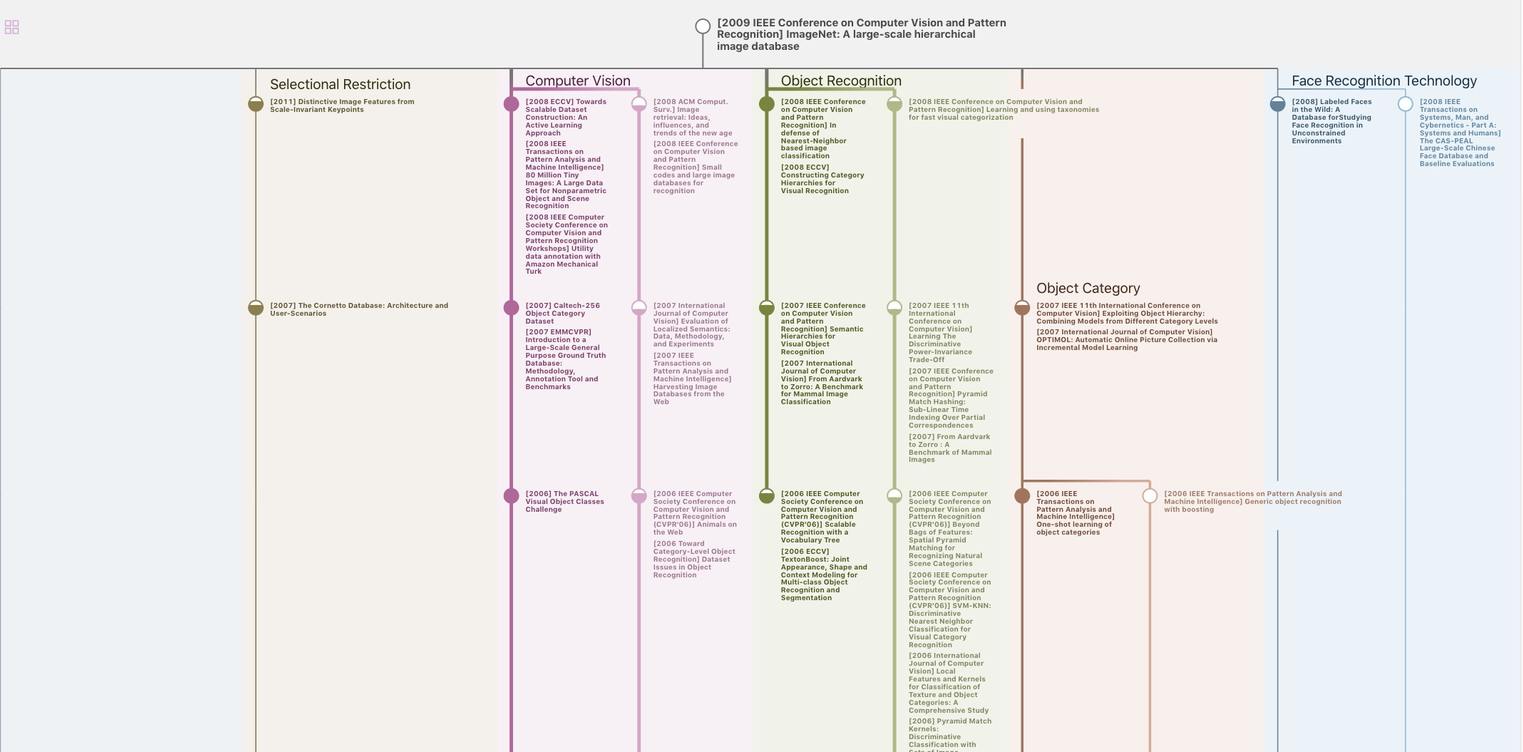
生成溯源树,研究论文发展脉络
Chat Paper
正在生成论文摘要