NuPS: A Parameter Server for Machine Learning with Non-Uniform Parameter Access
PROCEEDINGS OF THE 2022 INTERNATIONAL CONFERENCE ON MANAGEMENT OF DATA (SIGMOD '22)(2022)
摘要
Parameter servers (PSs) facilitate the implementation of distributed training for large machine learning tasks. In this paper, we argue that existing PSs are inefficient for tasks that exhibit non-uniform parameter access; their performance may even fall behind that of single node baselines. We identify two major sources of such nonuniform access: skew and sampling. Existing PSs are ill-suited for managing skew because they uniformly apply the same parameter management technique to all parameters. They are inefficient for sampling because the PS is oblivious to the associated randomized accesses and cannot exploit locality. To overcome these performance limitations, we introduce NuPS, a novel PS architecture that (i) integrates multiple management techniques and employs a suitable technique for each parameter and (ii) supports sampling directly via suitable sampling primitives and sampling schemes that allow for a controlled quality-efficiency trade-off. In our experimental study, NuPS outperformed existing PSs by up to one order of magnitude and provided up to linear scalability across multiple machine learning tasks.
更多查看译文
关键词
parameter servers, distributed machine learning, large-scale machine learning, skew, sampling
AI 理解论文
溯源树
样例
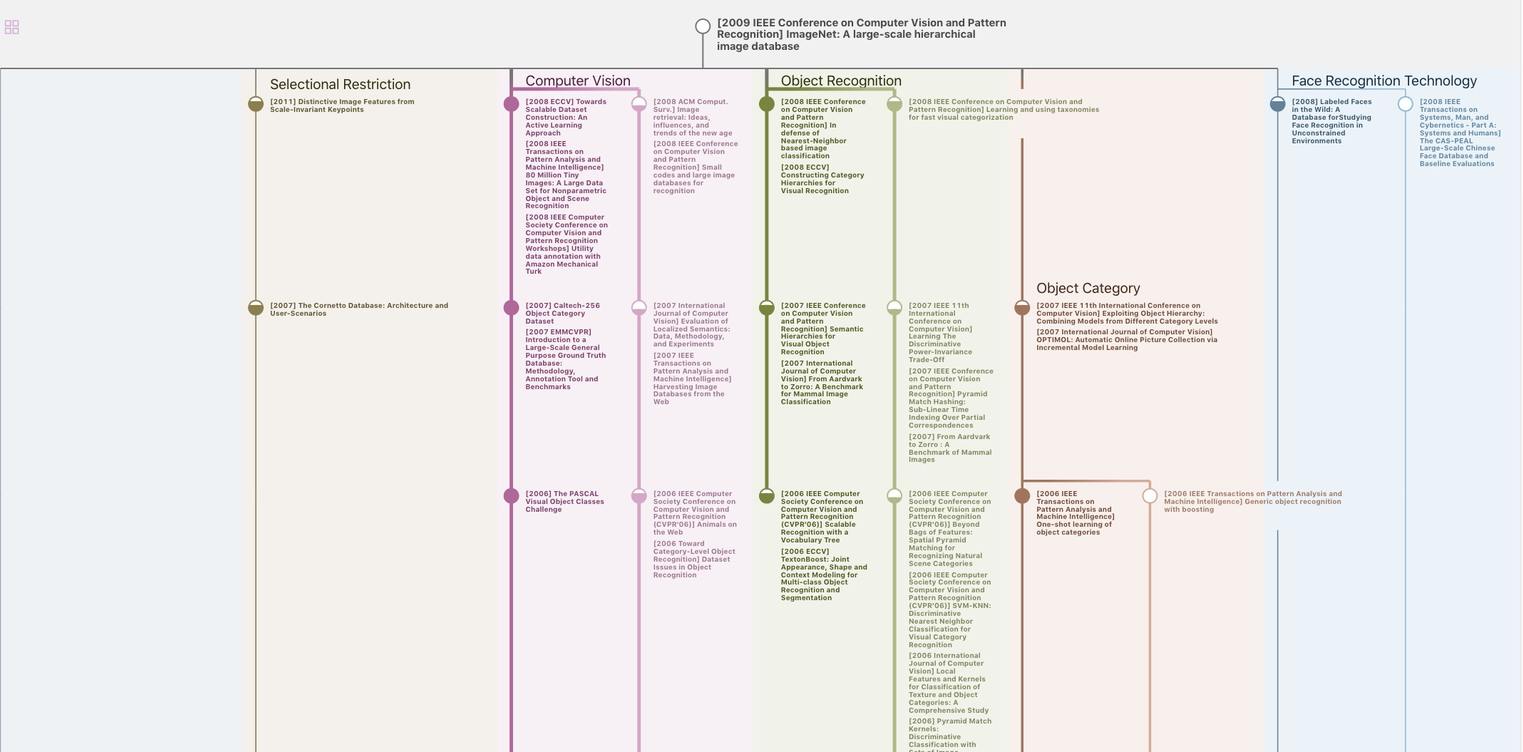
生成溯源树,研究论文发展脉络
Chat Paper
正在生成论文摘要