Deep Ladder-Suppression Network for Unsupervised Domain Adaptation
IEEE TRANSACTIONS ON CYBERNETICS(2022)
Abstract
Unsupervised domain adaptation (UDA) aims at learning a classifier for an unlabeled target domain by transferring knowledge from a labeled source domain with a related but different distribution. Most existing approaches learn domain-invariant features by adapting the entire information of the images. However, forcing adaptation of domain-specific variations undermines the effectiveness of the learned features. To address this problem, we propose a novel, yet elegant module, called the deep ladder-suppression network (DLSN), which is designed to better learn the cross-domain shared content by suppressing domain-specific variations. Our proposed DLSN is an autoencoder with lateral connections from the encoder to the decoder. By this design, the domain-specific details, which are only necessary for reconstructing the unlabeled target data, are directly fed to the decoder to complete the reconstruction task, relieving the pressure of learning domain-specific variations at the later layers of the shared encoder. As a result, DLSN allows the shared encoder to focus on learning cross-domain shared content and ignores the domain-specific variations. Notably, the proposed DLSN can be used as a standard module to be integrated with various existing UDA frameworks to further boost performance. Without whistles and bells, extensive experimental results on four gold-standard domain adaptation datasets, for example: 1) Digits; 2) Office31; 3) Office-Home; and 4) VisDA-C, demonstrate that the proposed DLSN can consistently and significantly improve the performance of various popular UDA frameworks.
MoreTranslated text
Key words
Measurement,Task analysis,Image reconstruction,Decoding,Feature extraction,Neural networks,Electronic mail,Autoencoder,deep convolutional neural network (DCNN),deep learning,domain adaptation (DA),transfer learning,unsupervised learning
AI Read Science
Must-Reading Tree
Example
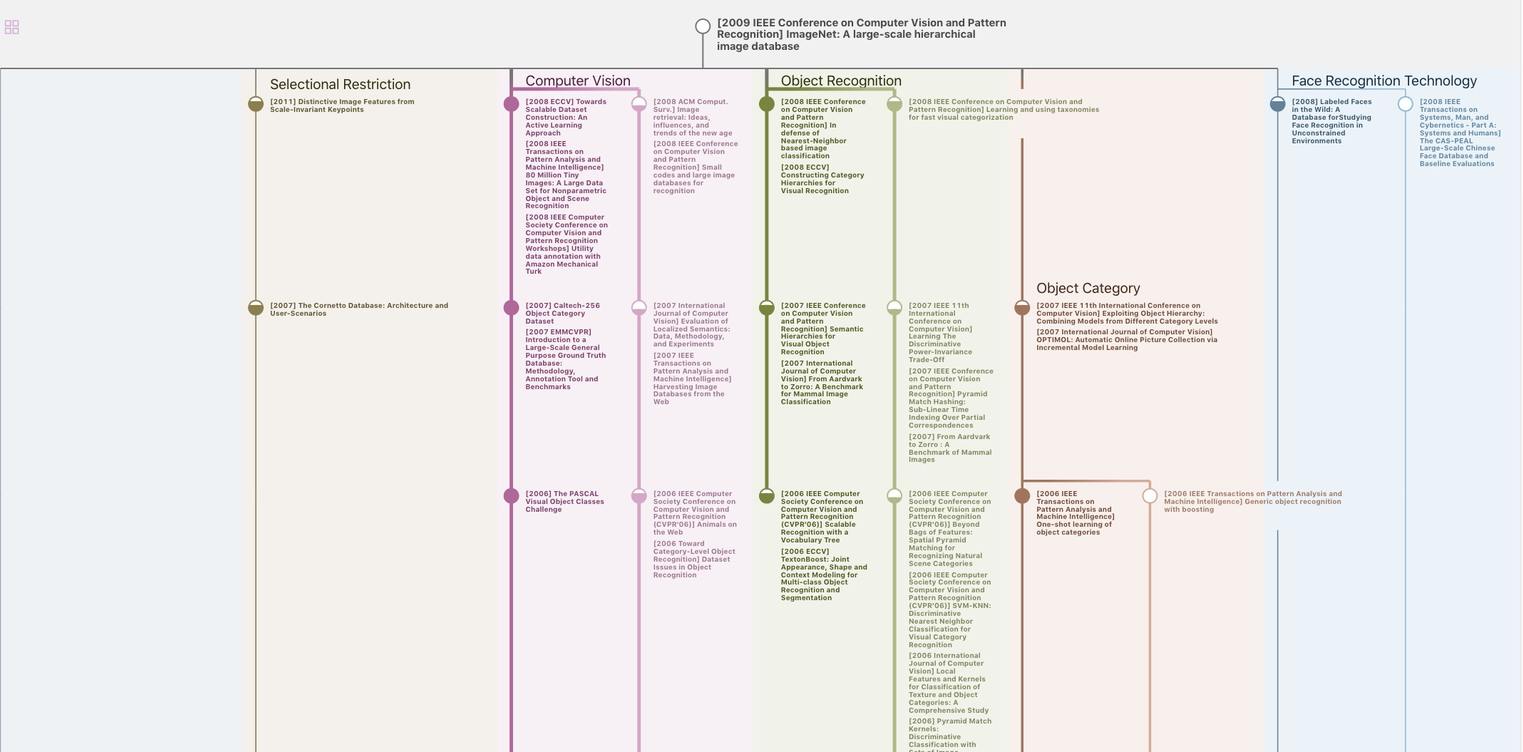
Generate MRT to find the research sequence of this paper
Chat Paper
Summary is being generated by the instructions you defined