Exploiting Invariance in Training Deep Neural Networks.
AAAI Conference on Artificial Intelligence(2022)
摘要
Inspired by two basic mechanisms in animal visual systems, we introduce a feature transform technique that imposes invariance properties in the training of deep neural networks. The resulting algorithm requires less parameter tuning, trains well with an initial learning rate 1.0, and easily generalizes to different tasks. We enforce scale invariance with local statistics in the data to align similar samples at diverse scales. To accelerate convergence, we enforce a GL(n)-invariance property with global statistics extracted from a batch such that the gradient descent solution should remain invariant under basis change. Profiling analysis shows our proposed modifications takes 5% of the computations of the underlying convolution layer. Tested on convolutional networks and transformer networks, our proposed technique requires fewer iterations to train, surpasses all baselines by a large margin, seamlessly works on both small and large batch size training, and applies to different computer vision and language tasks.
更多查看译文
关键词
Machine Learning (ML),Computer Vision (CV),Search And Optimization (SO),Constraint Satisfaction And Optimization (CSO)
AI 理解论文
溯源树
样例
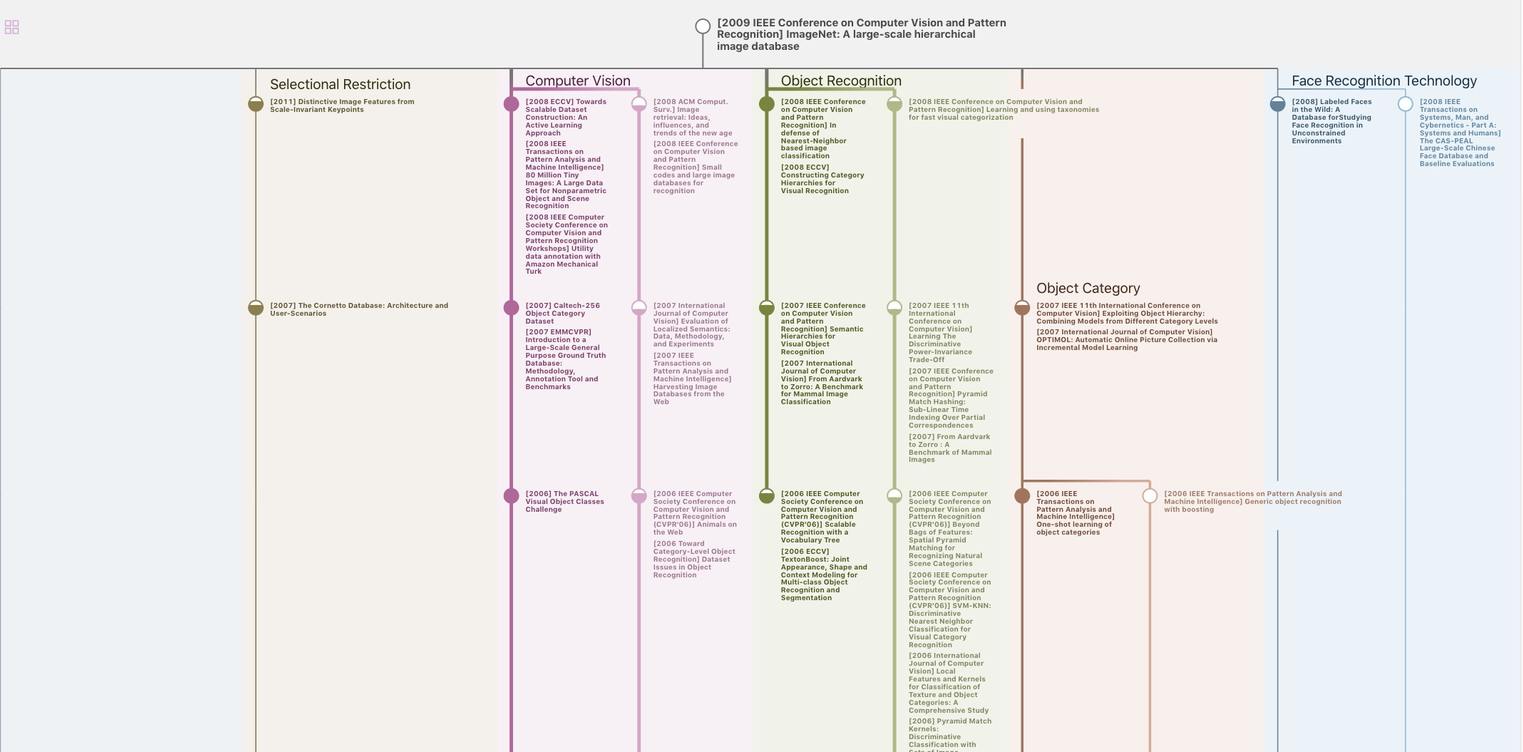
生成溯源树,研究论文发展脉络
Chat Paper
正在生成论文摘要