Learning algorithm reflecting universal scaling behavior near phase transitions
PHYSICAL REVIEW RESEARCH(2022)
摘要
Machine-learning-inspired techniques have emerged as a new paradigm for analysis of phase transitions in quantum matter. In this work, we introduce a supervised learning algorithm for studying critical phenomena from measurement data, "neural network scaling," which is based on iteratively training convolutional networks of increasing (spatial) complexity and test it on the transverse field Ising chain and q = 6 Potts model. At the continuous Ising transition, our scaling procedure directly reflects the hallmark of a continuous (second-order) phase transition, divergence of a characteristic length scale. Specifically, we extract a classification length scale by measuring the response of the classification accuracy while varying the largest convolution size (architecture of the network). We observe empirically the scaling exponent of the classification length is consistent with a power law with the correlation length exponent v = 1. Furthermore, we demonstrate the versatility of our algorithm by showing the universal scaling behaviors persist across a variety of measurement bases, including when the order parameter is nonlocal. Finally, we show that the classification length scale remains finite for the q = 6 Potts model, which has a first-order transition and lacks a divergent correlation length.
更多查看译文
关键词
learning algorithm,phase
AI 理解论文
溯源树
样例
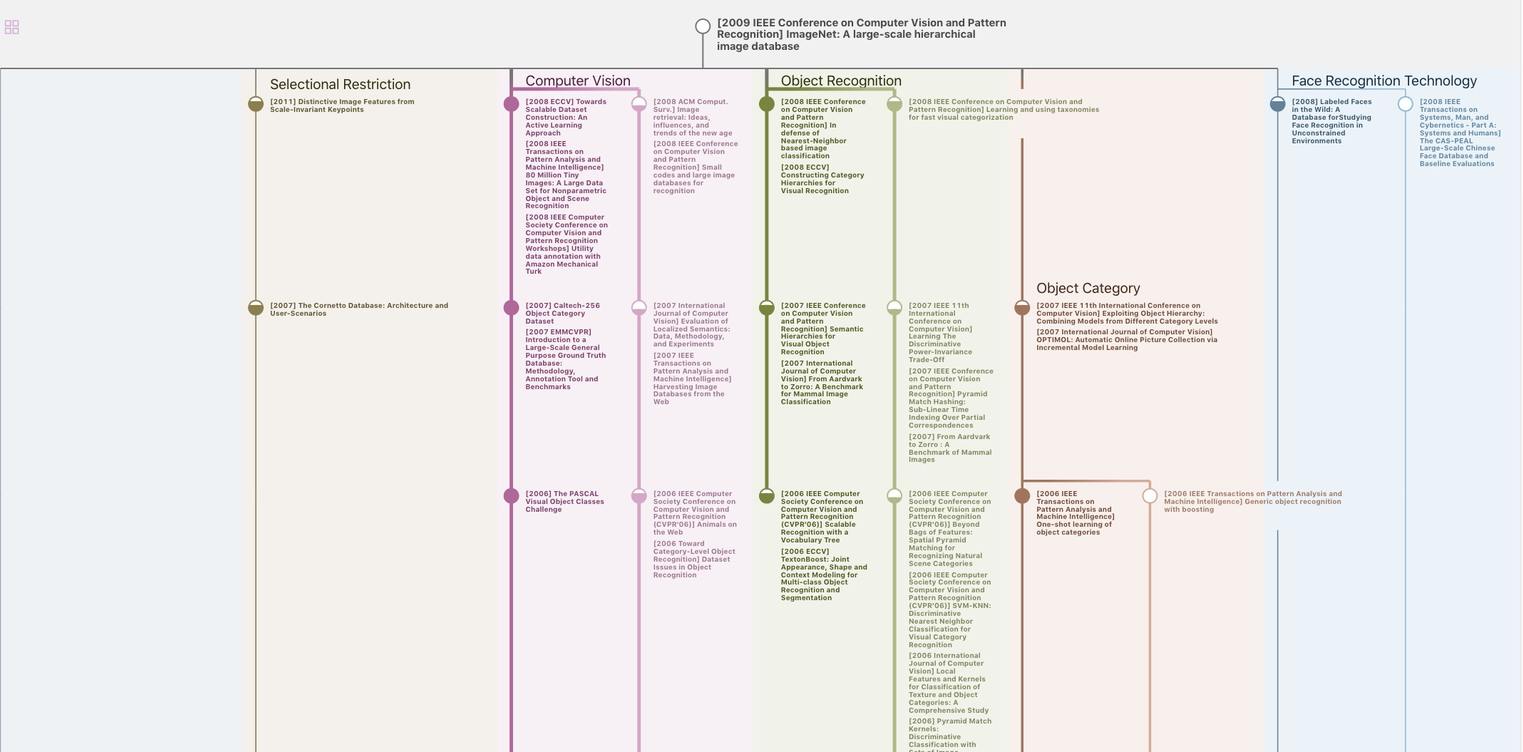
生成溯源树,研究论文发展脉络
Chat Paper
正在生成论文摘要