Adaptive Methods for Real-World Domain Generalization
2021 IEEE/CVF CONFERENCE ON COMPUTER VISION AND PATTERN RECOGNITION, CVPR 2021(2021)
摘要
Invariant approaches have been remarkably successful in tackling the problem of domain generalization, where the objective is to perform inference on data distributions different from those used in training. In our work, we investigate whether it is possible to leverage domain information from the unseen test samples themselves. We propose a domain-adaptive approach consisting of two steps: a) we first learn a discriminative domain embedding from unsupervised training examples, and b) use this domain embedding as supplementary information to build a domainadaptive model, that takes both the input as well as its domain into account while making predictions. For unseen domains, our method simply uses few unlabelled test examples to construct the domain embedding. This enables adaptive classification on any unseen domain. Our approach achieves state-of-the-art performance on various domain generalization benchmarks. In addition, we introduce the first real-world, large-scale domain generalization benchmark, Geo-YFCC, containing 1.1M samples over 40 training, 7 validation and 15 test domains, orders of magnitude larger than prior work. We show that the existing approaches either do not scale to this dataset or underperform compared to the simple baseline of training a model on the union of data from all training domains. In contrast, our approach achieves a significant 1% improvement.
更多查看译文
关键词
adaptive methods,real-world domain generalization,domain-adaptive approach,discriminative domain,unsupervised training examples,domain embedding,unseen domain,unlabelled test examples,adaptive classification,large-scale domain generalization benchmark
AI 理解论文
溯源树
样例
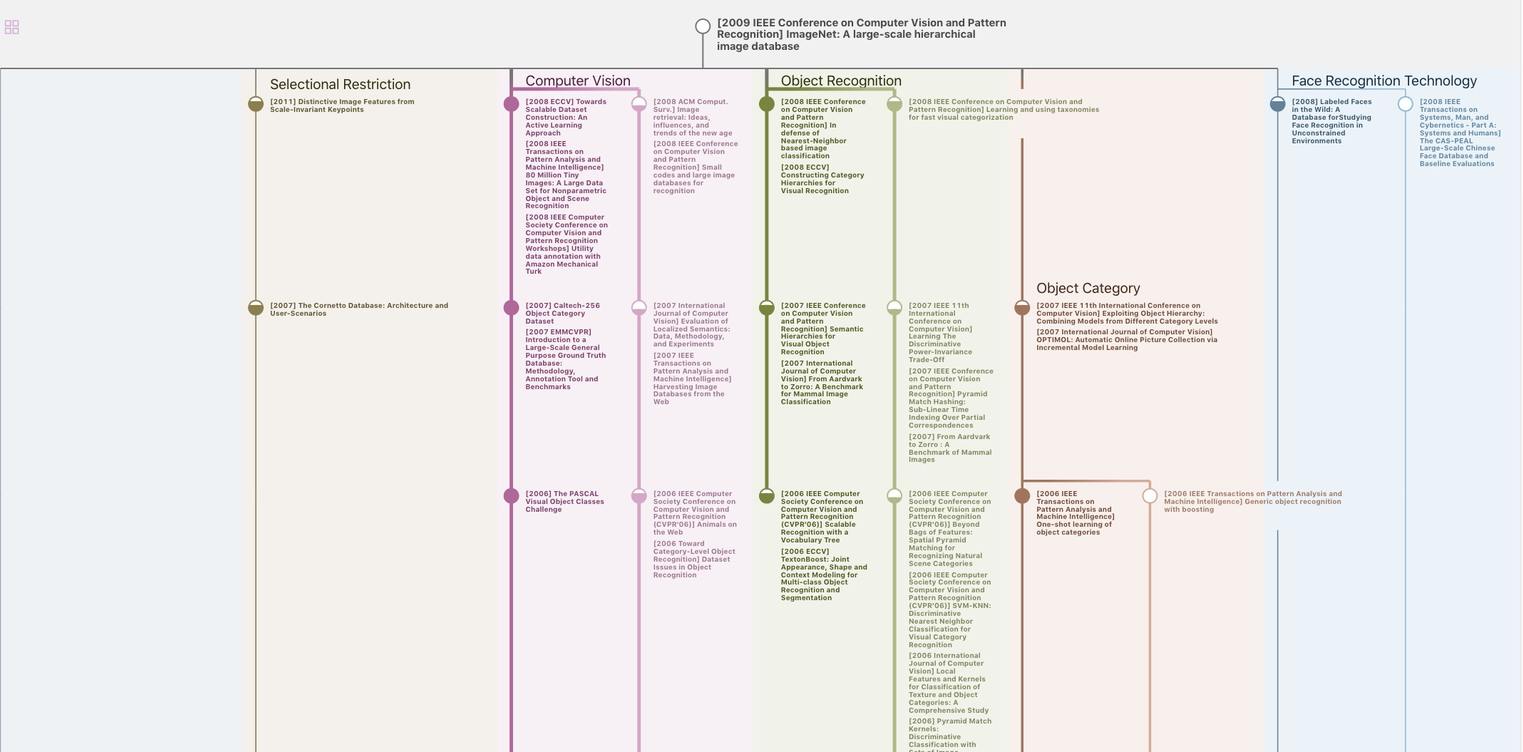
生成溯源树,研究论文发展脉络
Chat Paper
正在生成论文摘要