The Sample Complexity of Distribution-Free Parity Learning in the Robust Shuffle Model.
J. Priv. Confidentiality(2022)
摘要
We provide a lowerbound on the sample complexity of distribution-free parity learning in the realizable case in the shuffle model of differential privacy. Namely, we show that the sample complexity of learning $d$-bit parity functions is $\Omega(2^{d/2})$. Our result extends a recent similar lowerbound on the sample complexity of private agnostic learning of parity functions in the shuffle model by Cheu and Ullman. We also sketch a simple shuffle model protocol demonstrating that our results are tight up to $poly(d)$ factors.
更多查看译文
关键词
shuffle model,sample complexity,distribution-free
AI 理解论文
溯源树
样例
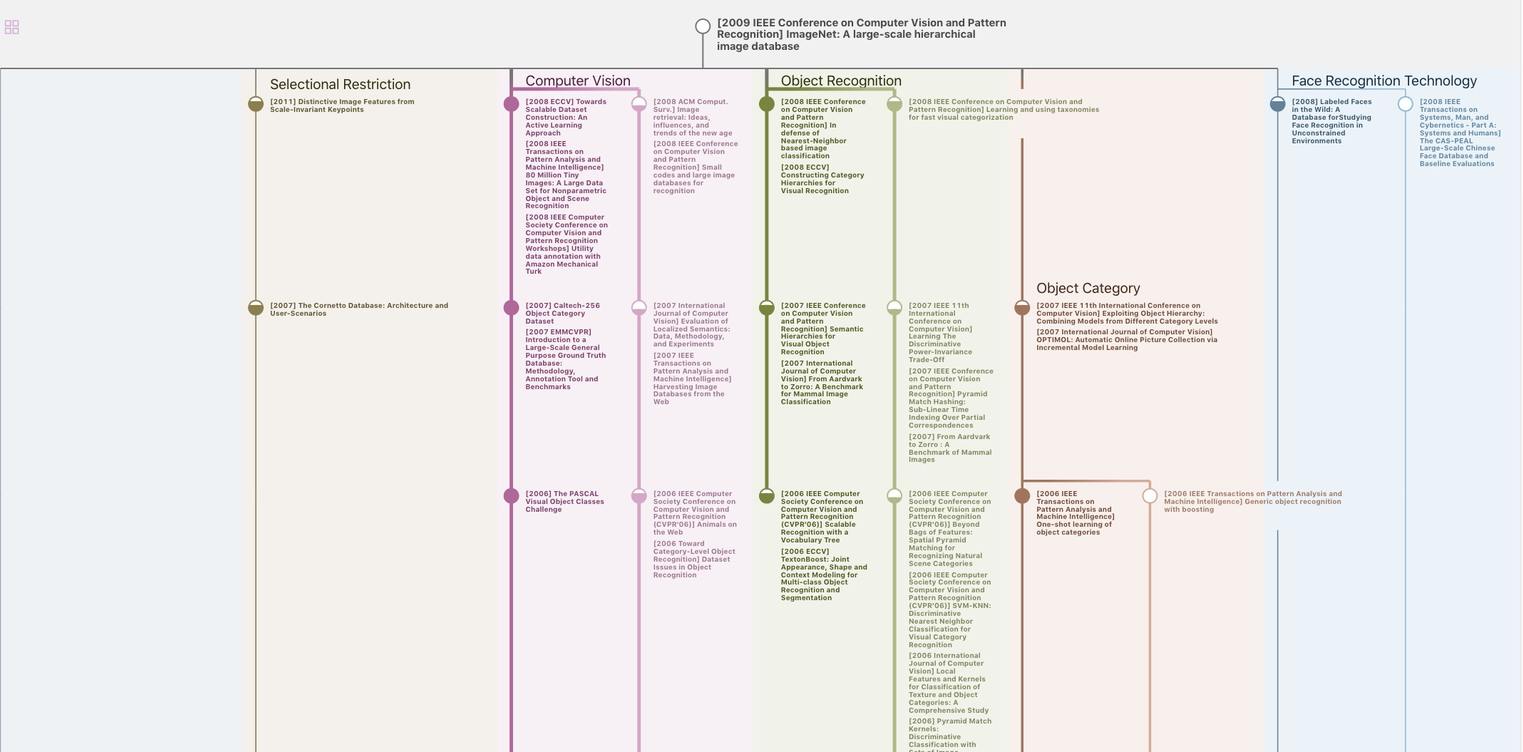
生成溯源树,研究论文发展脉络
Chat Paper
正在生成论文摘要