Improving Neural Question Generation using Deep Linguistic Representation
International World Wide Web Conference(2021)
摘要
ABSTRACT Question Generation (QG) is a challenging Natural Language Processing (NLP) task which aims at generating questions with given answers and context. There are many works incorporating linguistic features to improve the performance of QG. However, similar to traditional word embedding, these works normally embed such features with a set of trainable parameters, which results in the linguistic features not fully exploited. In this work, inspired by the recent achievements of text representation, we propose to utilize linguistic information via large pre-trained neural models. First, these models are trained in several specific NLP tasks in order to better represent linguistic features. Then, such feature representation is fused into a seq2seq based QG model to guide question generation. Extensive experiments were conducted on two benchmark Question Generation datasets to evaluate the effectiveness of our approach. The experimental results demonstrate that our approach outperforms the state-of-the-art QG systems, as a result, it significantly improves the baseline by 17.2% and 6.2% under the BLEU-4 metric on these two datasets, respectively.
更多查看译文
关键词
Question Generation, Embedding, Pre-trained Model, Linguistic Features
AI 理解论文
溯源树
样例
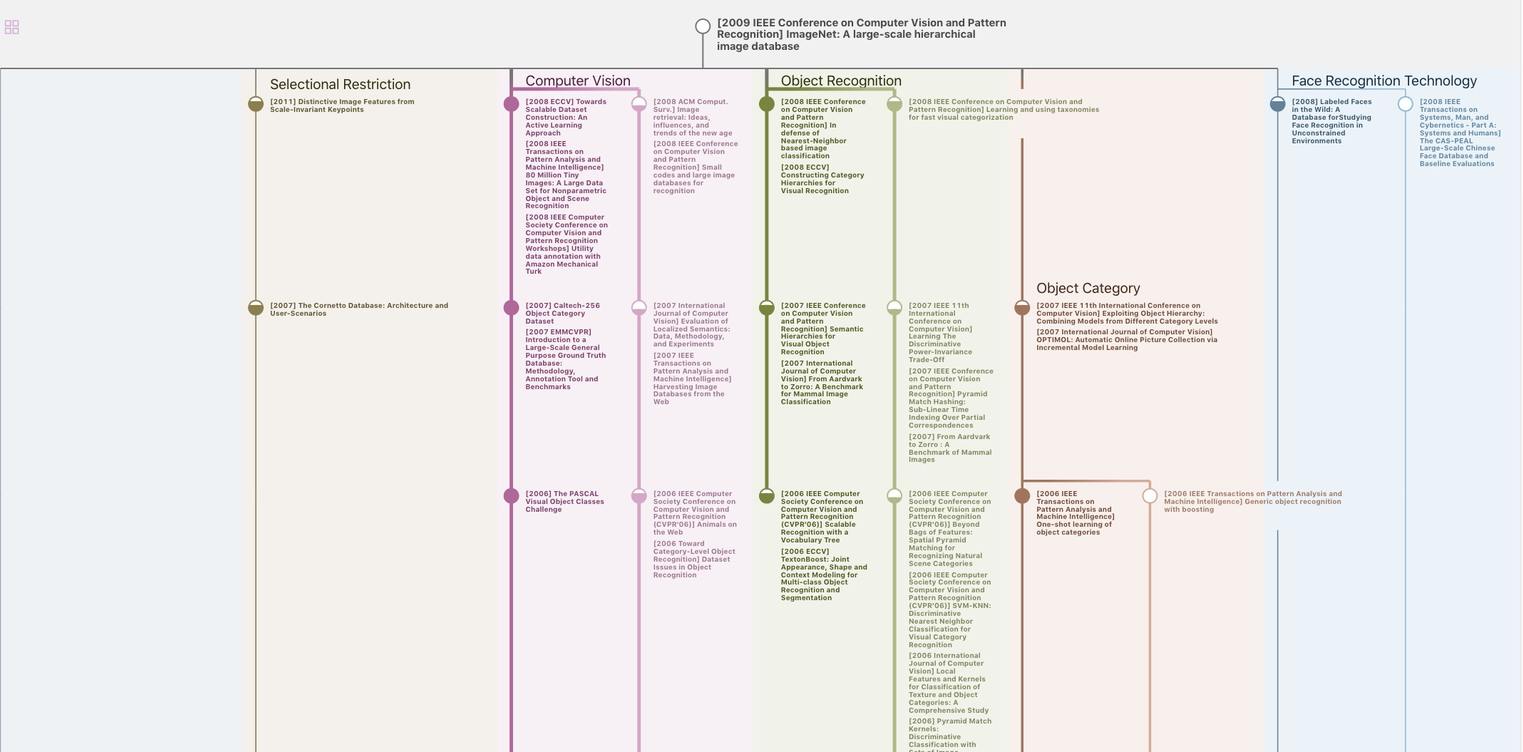
生成溯源树,研究论文发展脉络
Chat Paper
正在生成论文摘要