MStream: Fast Anomaly Detection in Multi-Aspect Streams
WWW(2021)
摘要
ABSTRACT Given a stream of entries in a multi-aspect data setting i.e., entries having multiple dimensions, how can we detect anomalous activities in an unsupervised manner? For example, in the intrusion detection setting, existing work seeks to detect anomalous events or edges in dynamic graph streams, but this does not allow us to take into account additional attributes of each entry. Our work aims to define a streaming multi-aspect data anomaly detection framework, termed MStream which can detect unusual group anomalies as they occur, in a dynamic manner. MStream has the following properties: (a) it detects anomalies in multi-aspect data including both categorical and numeric attributes; (b) it is online, thus processing each record in constant time and constant memory; (c) it can capture the correlation between multiple aspects of the data. MStream is evaluated over the KDDCUP99, CICIDS-DoS, UNSW-NB 15 and CICIDS-DDoS datasets, and outperforms state-of-the-art baselines.
更多查看译文
关键词
Anomaly Detection, Multi-Aspect Data, Stream, Intrusion Detection
AI 理解论文
溯源树
样例
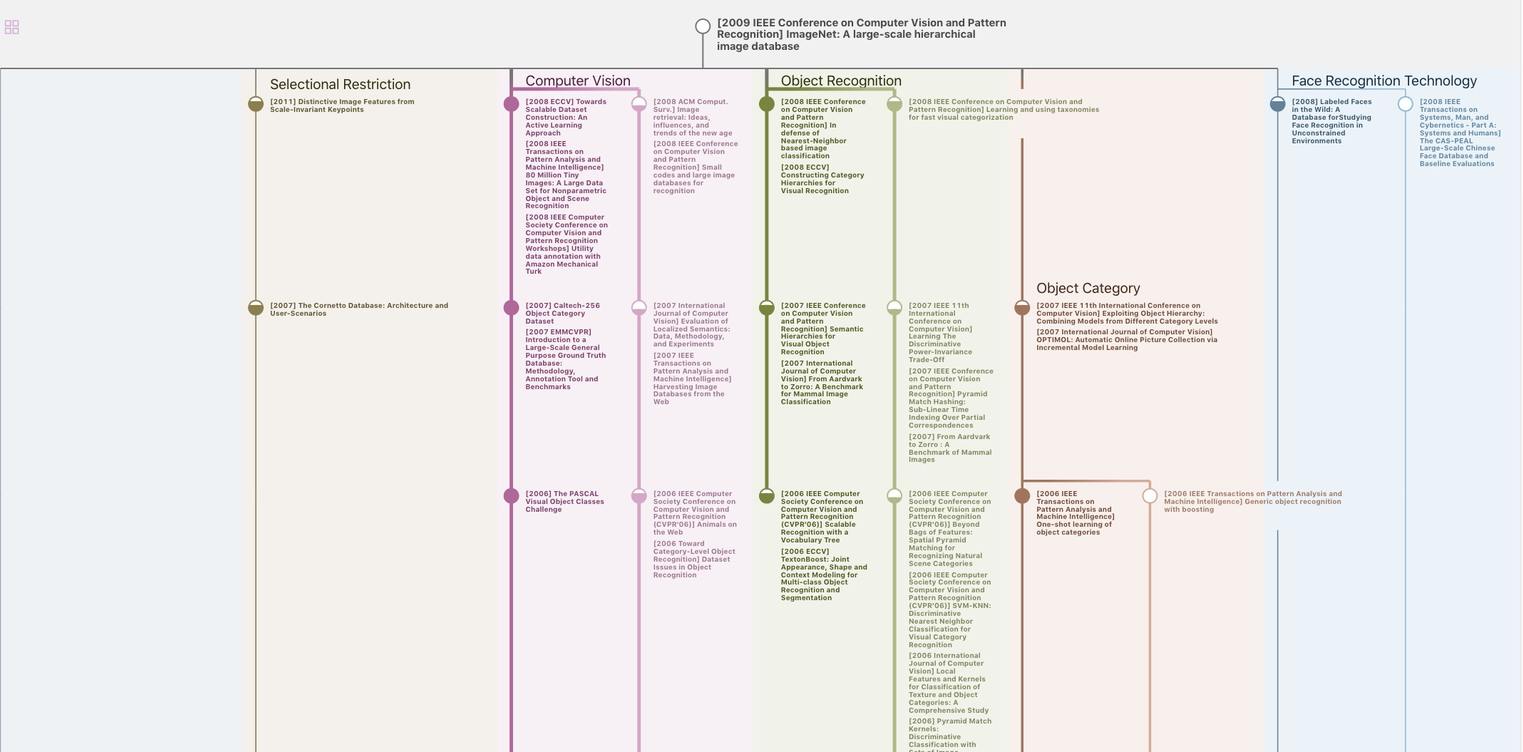
生成溯源树,研究论文发展脉络
Chat Paper
正在生成论文摘要