Graph Classification by Mixture of Diverse Experts
International Joint Conference on Artificial Intelligence(2021)
摘要
Graph classification is a challenging research problem in many applications across a broad range of domains. In these applications, it is very common that class distribution is imbalanced. Recently, Graph Neural Network (GNN) models have achieved superior performance on various real-world datasets. Despite their success, most of current GNN models largely overlook the important setting of imbalanced class distribution, which typically results in prediction bias towards majority classes. To alleviate the prediction bias, we propose to leverage semantic structure of dataset based on the distribution of node embedding. Specifically, we present GraphDIVE, a general framework leveraging mixture of diverse experts (i.e., graph classifiers) for imbalanced graph classification. With a divide-and-conquer principle, GraphDIVE employs a gating network to partition an imbalanced graph dataset into several subsets. Then each expert network is trained based on its corresponding subset. Experiments on real-world imbalanced graph datasets demonstrate the effectiveness of GraphDIVE.
更多查看译文
关键词
graphdive classification,diverse experts
AI 理解论文
溯源树
样例
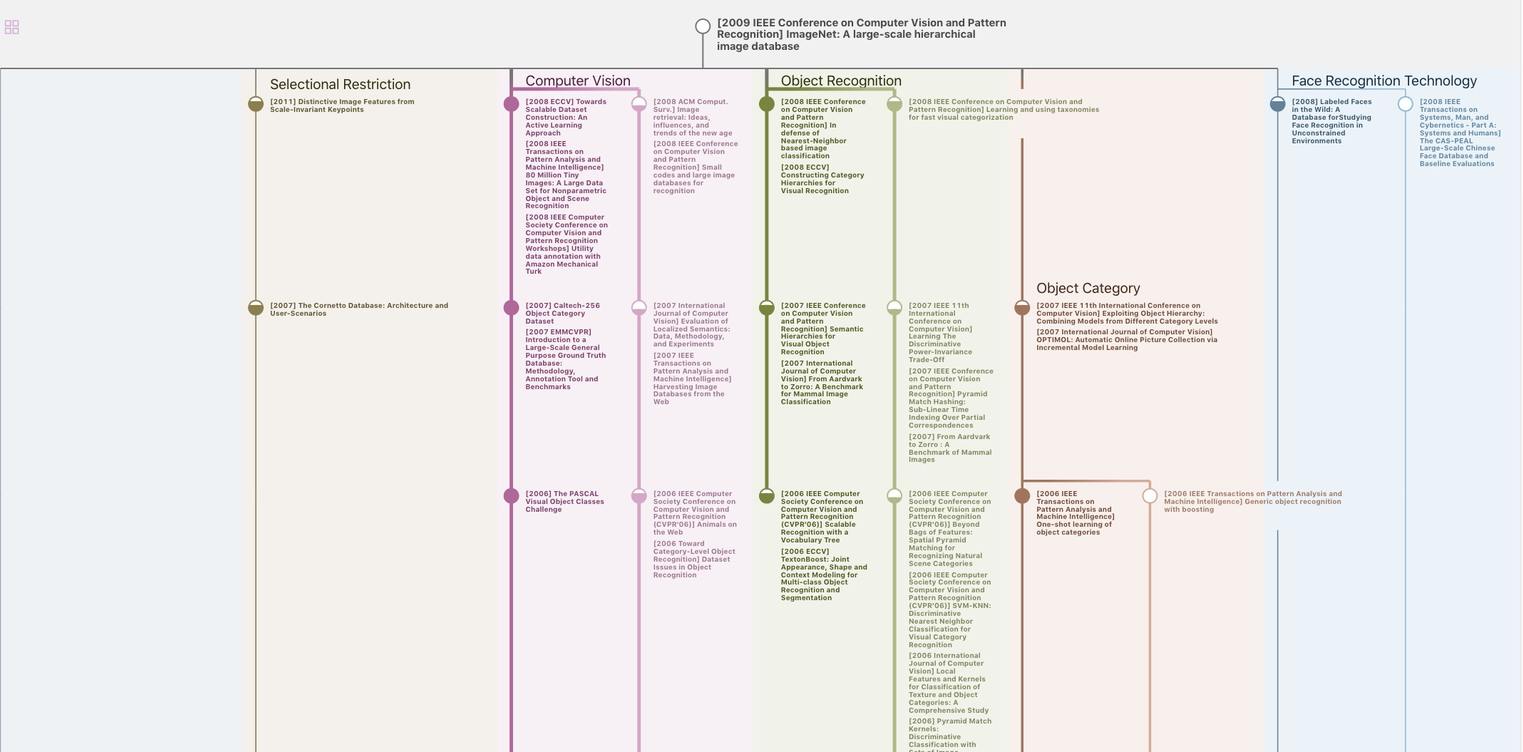
生成溯源树,研究论文发展脉络
Chat Paper
正在生成论文摘要