SELFGAIT: A SPATIOTEMPORAL REPRESENTATION LEARNING METHOD FOR SELF-SUPERVISED GAIT RECOGNITION
2021 IEEE INTERNATIONAL CONFERENCE ON ACOUSTICS, SPEECH AND SIGNAL PROCESSING (ICASSP 2021)(2021)
摘要
Gait recognition plays a vital role in human identification since gait is a unique biometric feature that can be perceived at a distance. Although existing gait recognition methods can learn gait features from gait sequences in different ways, the performance of gait recognition suffers from insufficient labeled data, especially in some practical scenarios associated with short gait sequences or various clothing styles. It is unpractical to label the numerous gait data. In this work, we propose a self-supervised gait recognition method, termed SelfGait, which takes advantage of the massive, diverse, unlabeled gait data as a pre-training process to improve the representation abilities of spatiotemporal backbones. Specifically, we employ the horizontal pyramid mapping (HPM) and micro-motion template builder (MTB) as our spatiotemporal backbones to capture the multi-scale spatiotemporal representations. Experiments on CASIA-B and OU-MVLP benchmark gait datasets demonstrate the effectiveness of the proposed SelfGait compared with four state-of-the-art gait recognition methods. The source code has been released at https://github.com/EchoItLiu/SelfGait.
更多查看译文
关键词
Gait Recognition, Self-Supervised, Contrastive Learning, Multi-Scale Feature Pyramid, Spatiotemporal Representation
AI 理解论文
溯源树
样例
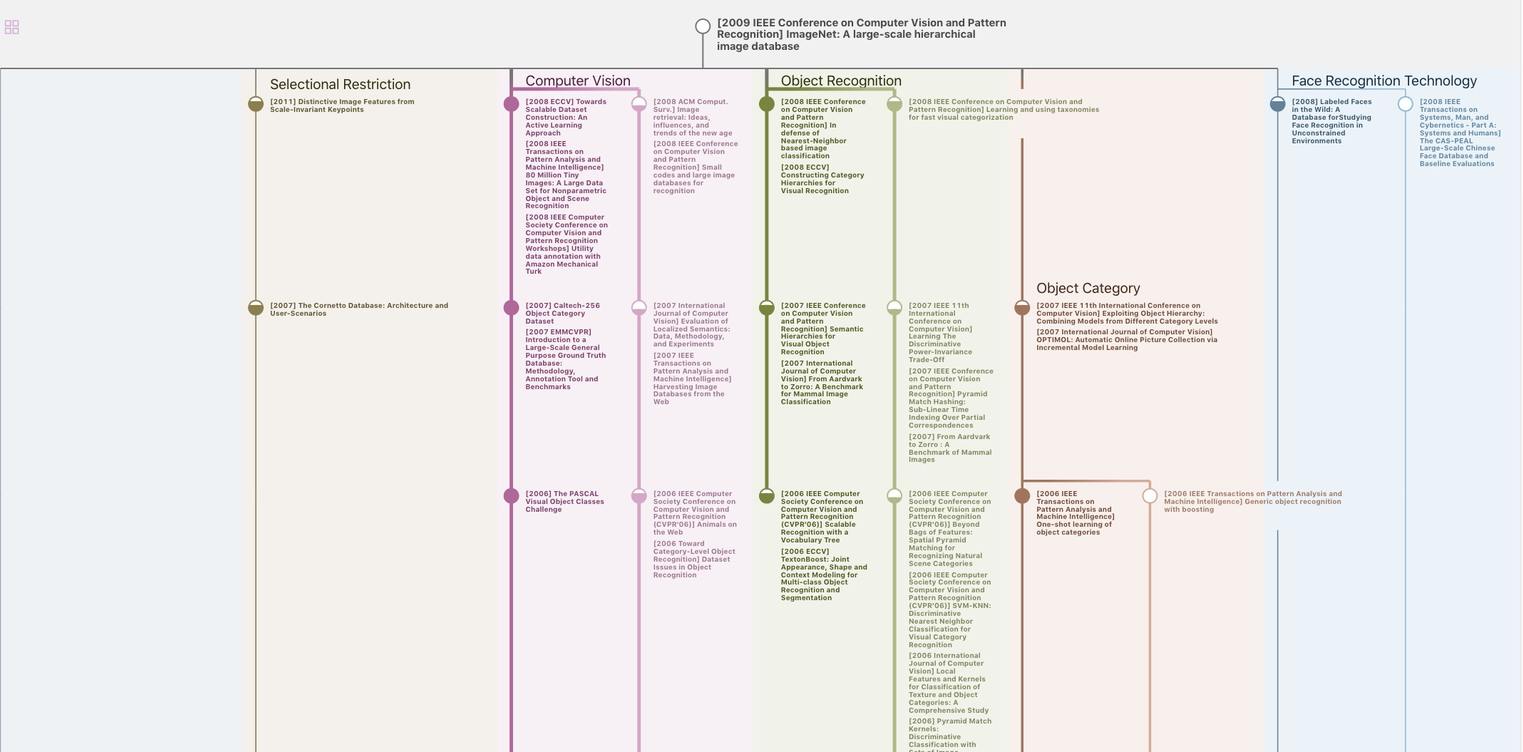
生成溯源树,研究论文发展脉络
Chat Paper
正在生成论文摘要