A Physics-Informed Neural Network for Quantifying the Microstructural Properties of Polycrystalline Nickel Using Ultrasound Data: A promising approach for solving inverse problems
IEEE SIGNAL PROCESSING MAGAZINE(2022)
摘要
We employ physics-informed neural networks (PINNs) to quantify the microstructure of polycrystalline nickel by computing the spatial variation of compliance coefficients (compressibility, stiffness, and rigidity) of the material. The PINNs are supervised with realistic ultrasonic surface acoustic wavefield data acquired at an ultrasonic frequency of 5 MHz for the polycrystalline material. The ultrasonic wavefield data are represented as a deformation on the top surface of the material with the deformation measured using the method of laser vibrometry. The ultrasonic data are further complemented with wavefield data generated using a finite-element-based solver. The neural network is physically informed by the in-plane and out-of-plane elastic wave equations, and its convergence is accelerated using adaptive activation functions. The overarching goal of this work is to infer the spatial variation of compliance coefficients of materials using PINNs, which for ultrasound involves the spatially varying speed of the elastic waves. More broadly, the resulting PINN-based surrogate model shows a promising approach for solving ill-posed inverse problems, often encountered in the nondestructive evaluation of materials.
更多查看译文
关键词
Ultrasonic imaging, Inverse problems, Ultrasonic variables measurement, Neural networks, Surface acoustic waves, Surface emitting lasers, Acoustics
AI 理解论文
溯源树
样例
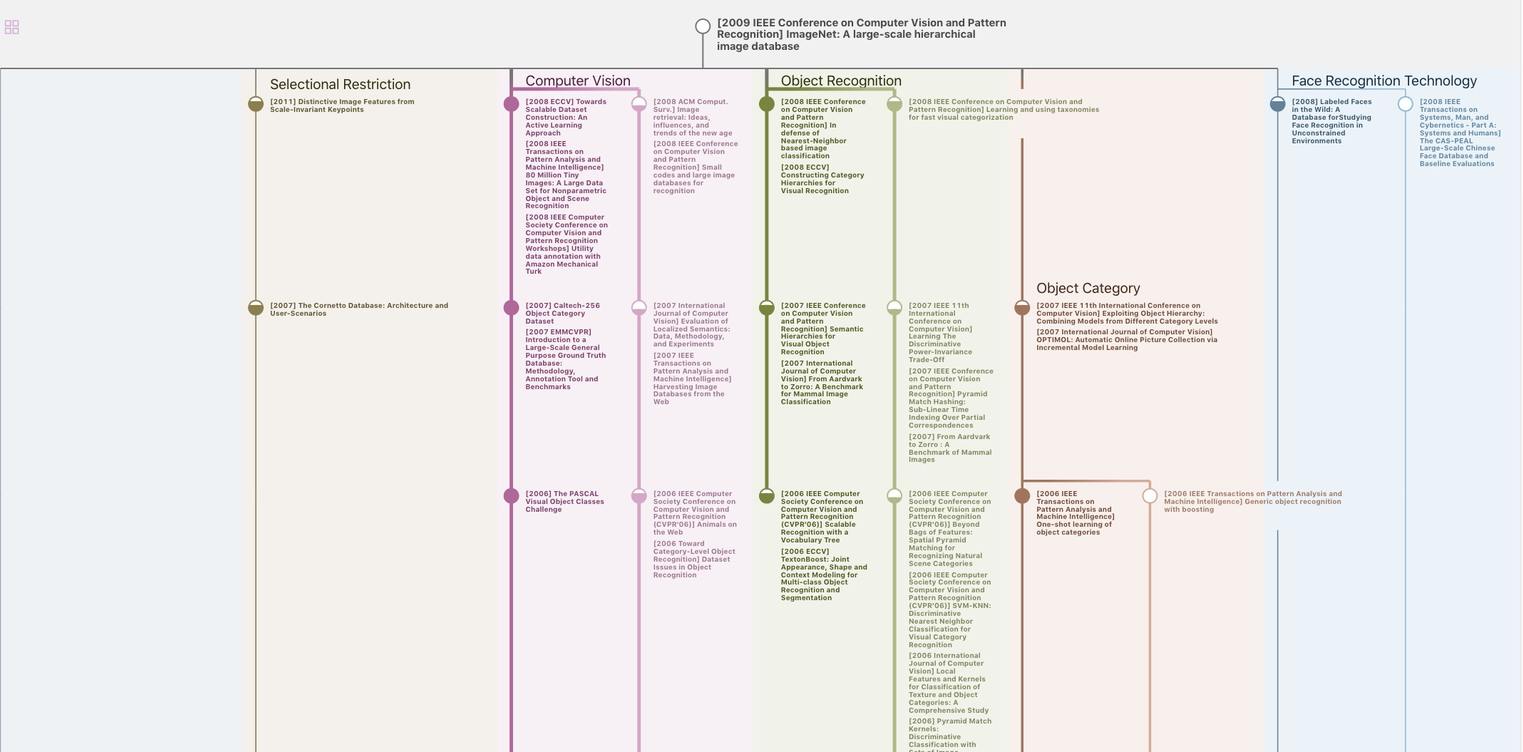
生成溯源树,研究论文发展脉络
Chat Paper
正在生成论文摘要