Approximating Pareto Fronts in Evolutionary Multiobjective Optimization with Large Population Size.
EMO(2021)
摘要
Approximating the Pareto fronts (PFs) of multiobjective optimization problems (MOPs) with a population of nondominated solutions is a common strategy in evolutionary multiobjective optimization (EMO). In the case of two or three objectives, the PFs of MOPs can be well approximated by the populations including several dozens or hundreds of nondominated solutions. However, this is not the case when approximating the PFs of many-objective optimization problems (MaOPs). Due to the high dimensionality in the objective space, almost all EMO algorithms with Pareto dominance encounter the difficulty in converging towards the PFs of MaOPs. In contrast, most of efficient EMO algorithms for many-objective optimization use the idea of decomposition in fitness assignment. It should be pointed out that small population size is often used in these many-objective optimization algorithms, which focus on the approximation of PFs along some specific search directions. In this paper, we studied the extensions of two well-known algorithms (i.e., NSGA-II and MOEA/D) with the ability to find a large population of nondominated solutions with good spread. A region-based archiving method is also suggested to reduce the computational complexity of updating external population. Our experimental results showed that these two extensions have good potential to find the PFs of MaOPs.
更多查看译文
关键词
Multiobjective optimization,NSGA-II/LP,MOEA/D-LP,Large population,Relaxed epsilon dominance
AI 理解论文
溯源树
样例
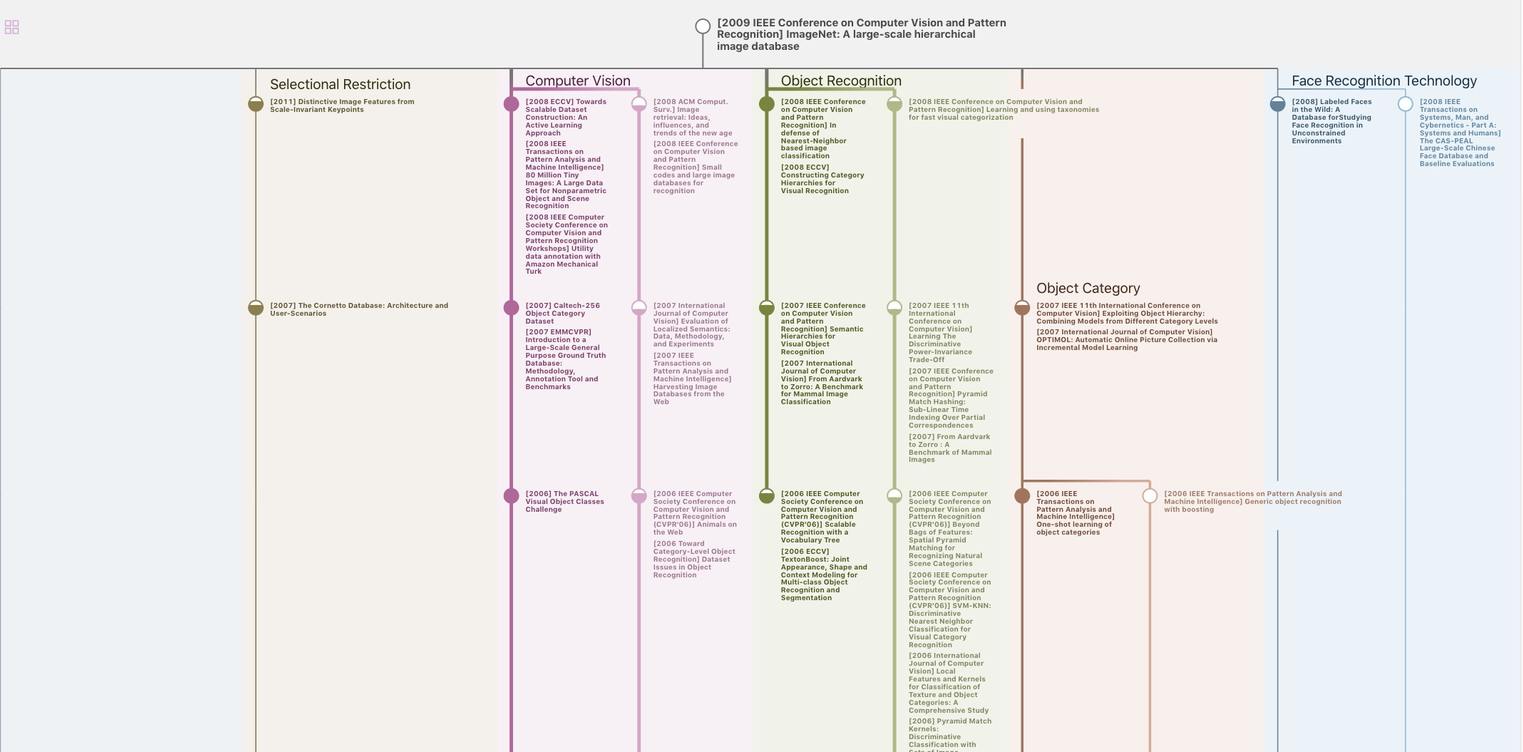
生成溯源树,研究论文发展脉络
Chat Paper
正在生成论文摘要