Iterative Learning for Model Reactive Control: Application to Autonomous Multi-agent Control
2021 7th International Conference on Automation, Robotics and Applications (ICARA)(2021)
摘要
In this paper, a decentralized autonomous controller aimed to control a fleet of quadrotors is designed, based on the iterative generation and exploitation of logged traces. The presented approach, inspired by model predictive control, aims to maintain the geometrical configuration for a set of quadrotors led by remotely controlled leaders. The novelty of this approach is to rely on inexpensive commercial off-the-shelf sensors (as opposed to positioning systems and/or cameras) that only measure the distance among quadrotors. In the first phase (trace generation) quadrotors are operated using randomized controllers based on domain knowledge, and their trajectories are registered. In the exploitation phase, a policy is learned from the traces generated in the previous phase, and the policy is iteratively refined, to achieve a robust reactive control of each quadrotor agent. Extensive experiments using RotorS, a Software In the Loop (SITL) framework in Gazebo simulator demonstrates the efficiency of the approach, and its ability to preserve the flocking structure of the quadrotors, following the (remotely and independently controlled) leaders.
更多查看译文
关键词
Quadrotors,leader-follower,machine learning,iterative learning,model predictive control,neural networks
AI 理解论文
溯源树
样例
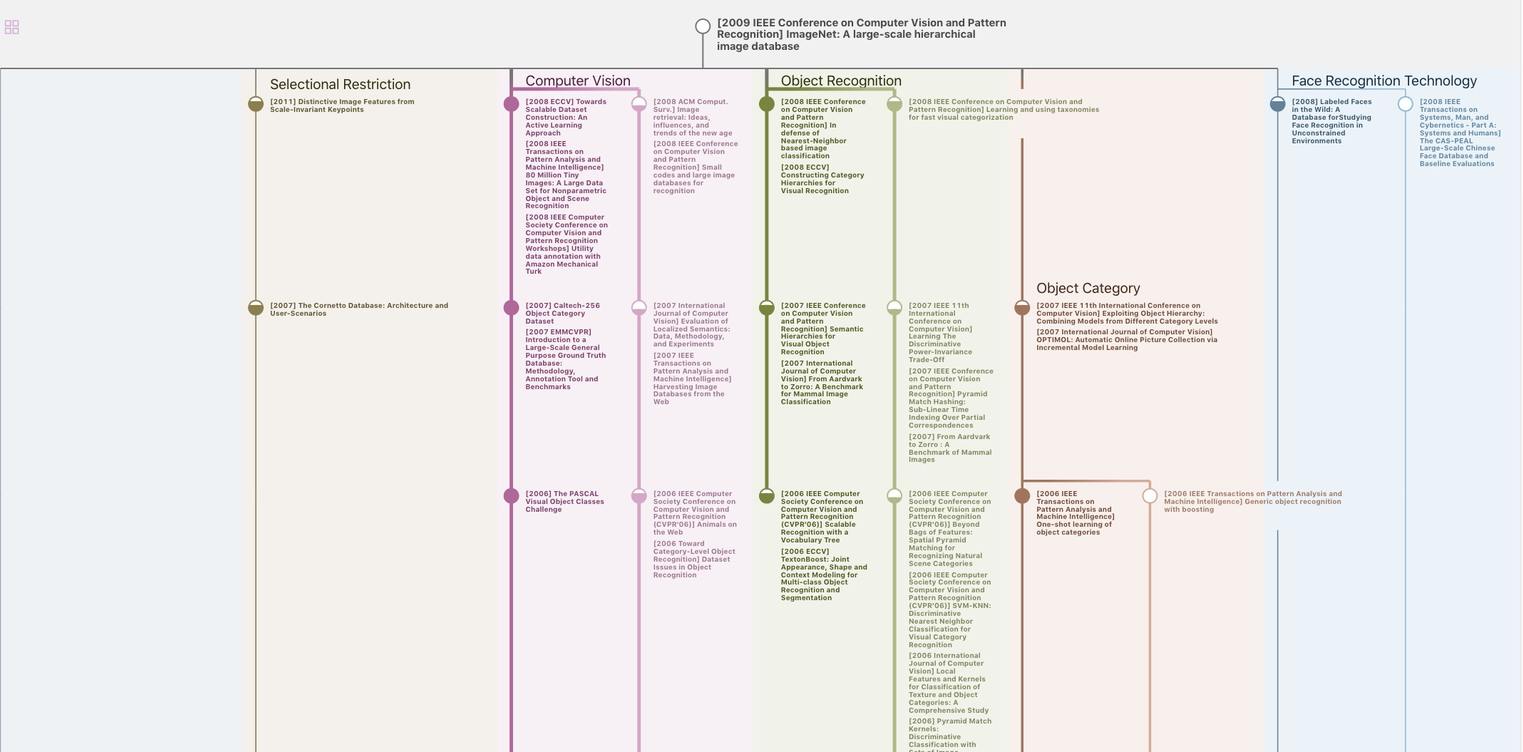
生成溯源树,研究论文发展脉络
Chat Paper
正在生成论文摘要