Variational Autoencoder-Based Vehicle Trajectory Prediction with an Interpretable Latent Space.
ITSC(2021)
摘要
This paper introduces the Descriptive Variational Autoencoder (DVAE), an unsupervised and end-to-end trainable neural network for predicting vehicle trajectories that provides partial interpretability. The novel approach is based on the architecture and objective of common variational autoencoders. By introducing expert knowledge within the decoder part of the autoencoder, the encoder learns to extract latent parameters that provide a graspable meaning in human terms. Such an interpretable latent space enables the validation by expert defined rule sets. The evaluation of the DVAE is performed using the publicly available highD dataset for highway traffic scenarios. In comparison to a conventional variational autoencoder with equivalent complexity, the proposed model provides a similar prediction accuracy but with the great advantage of having an interpretable latent space. For crucial decision making and assessing trustworthiness of a prediction this property is highly desirable.
更多查看译文
关键词
Variational Autoencoder-based vehicle trajectory prediction,interpretable latent space,Descriptive Variational Autoencoder,DVAE,unsupervised end-to-end trainable neural network,vehicle trajectories,partial interpretability,common variational autoencoders,latent parameters,expert defined rule sets,conventional variational autoencoder,similar prediction accuracy,prediction this property
AI 理解论文
溯源树
样例
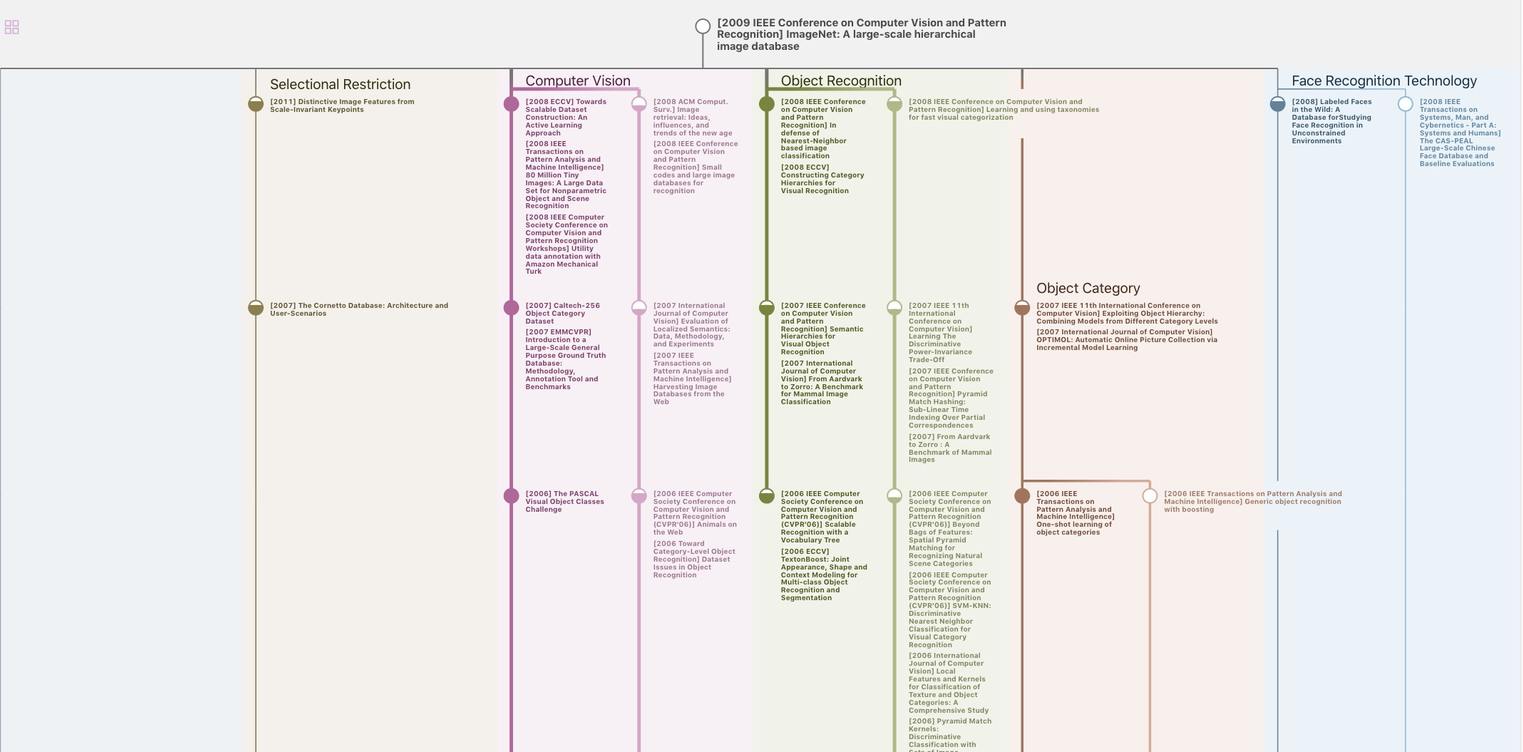
生成溯源树,研究论文发展脉络
Chat Paper
正在生成论文摘要