Contrast to Divide: Self-Supervised Pre-Training for Learning with Noisy Labels
2022 IEEE/CVF Winter Conference on Applications of Computer Vision (WACV)(2022)
摘要
The success of learning with noisy labels (LNL) methods relies heavily on the success of a warm-up stage where standard supervised training is performed using the full (noisy) training set. In this paper, we identify a "warm-up obstacle": the inability of standard warm-up stages to train high quality feature extractors and avert memorization of noisy labels. We propose "Contrast to Divide" (C2D), a simple framework that solves this problem by pre-training the feature extractor in a self-supervised fashion. Using self-supervised pre-training boosts the performance of existing LNL approaches by drastically reducing the warm-up stage's susceptibility to noise level, shortening its duration, and improving extracted feature quality. C2D works out of the box with existing methods and demonstrates markedly improved performance, especially in the high noise regime, where we get a boost of more than 27% for CIFAR-100 with 90% noise over the previous state of the art. In real-life noise settings, C2D trained on mini-WebVision outperforms previous works both in WebVision and ImageNet validation sets by 3% top-1 accuracy. We perform an in-depth analysis of the framework, including investigating the performance of different pre-training approaches and estimating the effective upper bound of the LNL performance with semi-supervised learning. Code for reproducing our experiments is available at https://github.com/ContrastToDivide/C2D.
更多查看译文
关键词
Transfer, Few-shot, Semi- and Un- supervised Learning Deep Learning, Large-scale Vision Applications
AI 理解论文
溯源树
样例
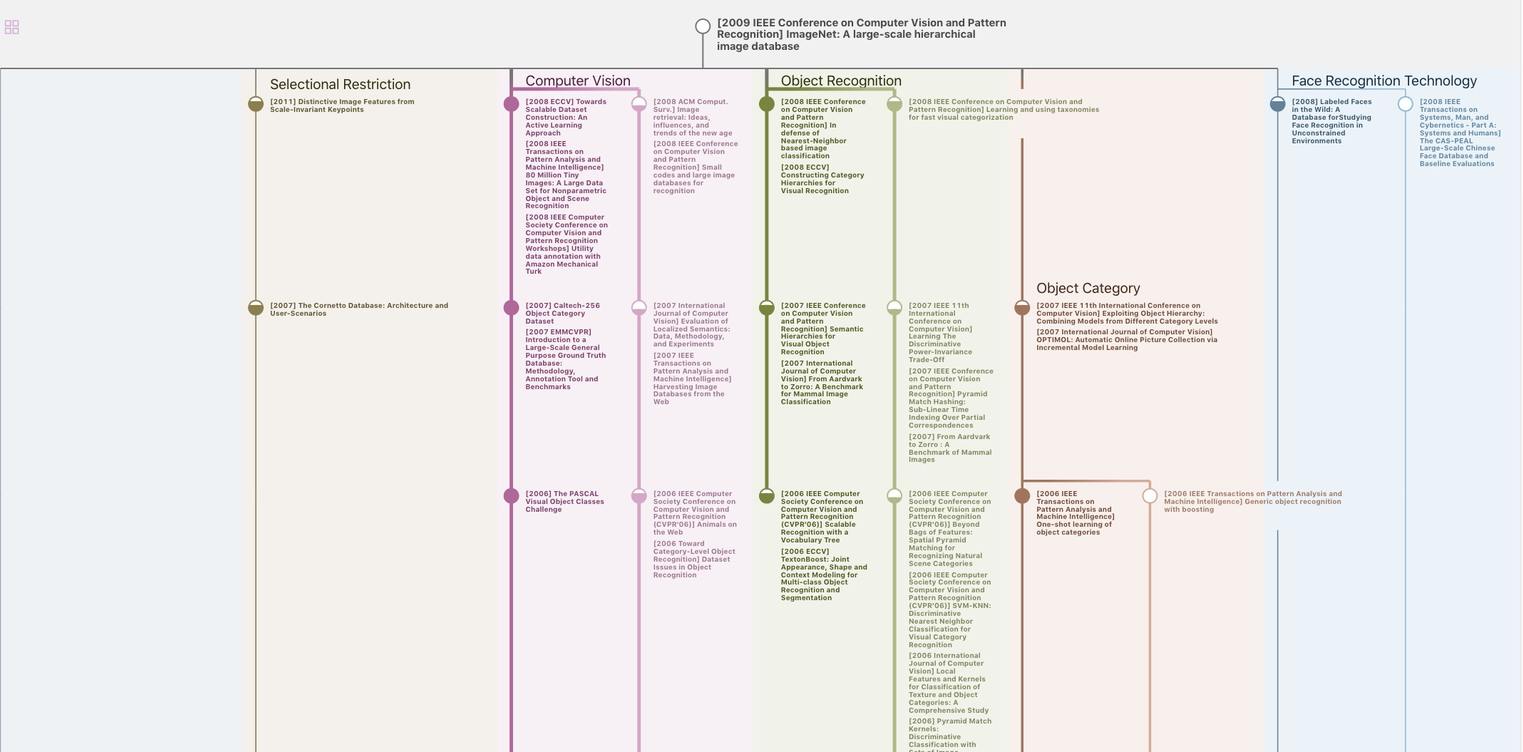
生成溯源树,研究论文发展脉络
Chat Paper
正在生成论文摘要