Dialogue Act Recognition using Branch Architecture with Attention Mechanism for Imbalanced Data
2021 12th International Symposium on Chinese Spoken Language Processing (ISCSLP)(2021)
摘要
Dialogue act recognition is a sequence labeling task that maps the dialogue act tag to each utterance in a conversation. Previous works on dialogue act recognition have investigated many methods, such as using Bi-LSTM-CRF model to improve accuracy. However, these methods ignore the problem caused by the imbalanced distribution of the data. In this paper, we target at dealing with the class imbalance problem on dialogue act recognition, and propose a branch architecture to predict different level data. The whole framework reflects a hierarchical pattern. The branches can induce global regularization, which is conducive to the utterance layer, help LSTM model to capture the features for minority classes. We also exploit self-attention mechanism after utterance layer to capture dependencies among words. Experimental results on a mandarin dialogue corpus, called CASIA-CASSIL corpus, show that our framework significantly outperforms other methods. And our experimental results also indicate the effectiveness of punctuation on the branch model and the interaction between two branches.
更多查看译文
关键词
Spoken language understanding,Adversarial training,Self-attention mechanism
AI 理解论文
溯源树
样例
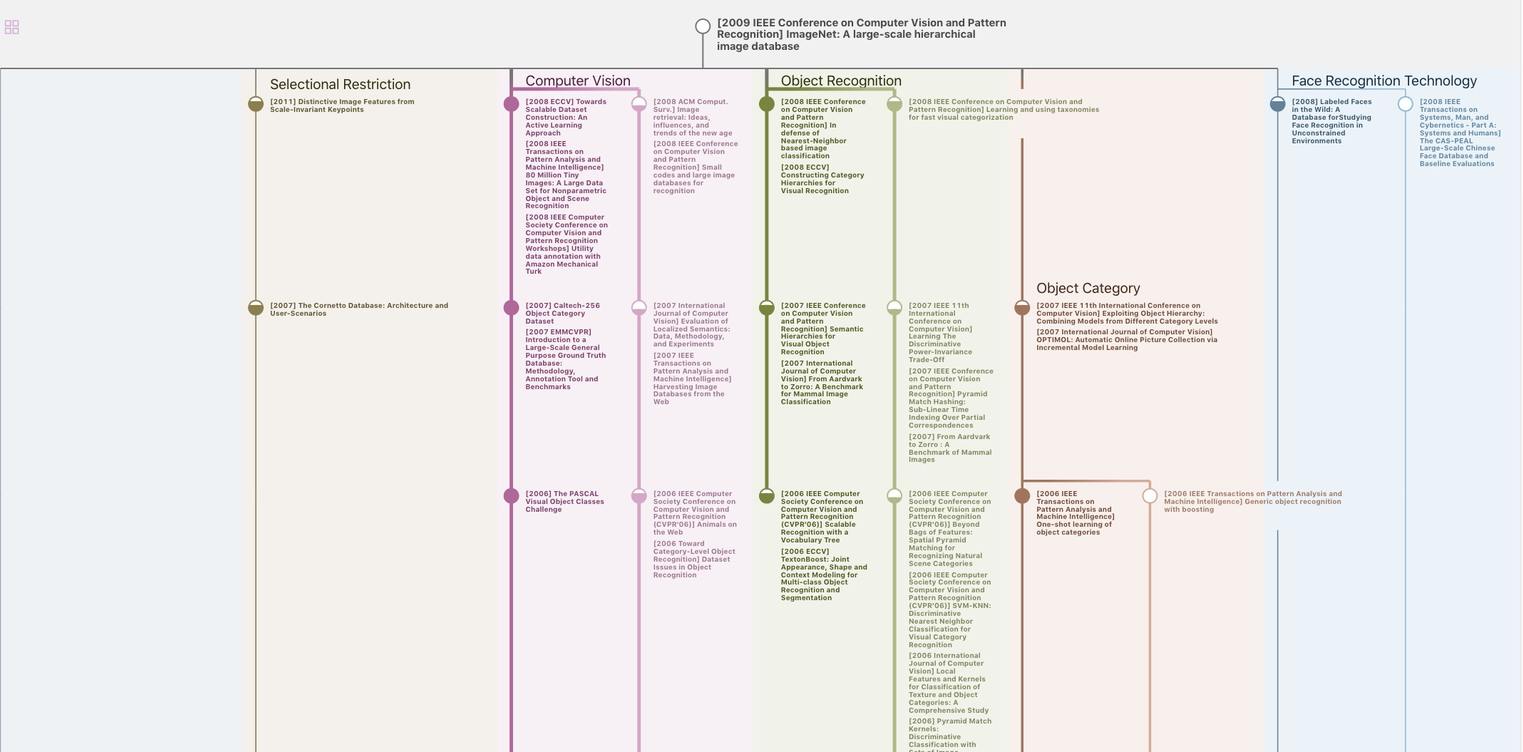
生成溯源树,研究论文发展脉络
Chat Paper
正在生成论文摘要