Privacy-Aware Personal Data Storage (P-PDS): Learning how to Protect User Privacy from External Applications
Periodicals(2021)
摘要
AbstractRecently, Personal Data Storage (PDS) has inaugurated a substantial change to the way people can store and control their personal data, by moving from a service-centric to a user-centric model. PDS offers individuals the capability to keep their data in a unique logical repository, that can be connected and exploited by proper analytical tools, or shared with third parties under the control of end users. Up to now, most of the research on PDS has focused on how to enforce user privacy preferences and how to secure data when stored into the PDS. In contrast, in this paper we aim at designing a Privacy-aware Personal Data Storage (P-PDS), that is, a PDS able to automatically take privacy-aware decisions on third parties access requests in accordance with user preferences. The proposed P-PDS is based on preliminary results presented in [1] , where it has been demonstrated that semi-supervised learning can be successfully exploited to make a PDS able to automatically decide whether an access request has to be authorized or not. In this paper, we have deeply revised the learning process in order to have a more usable P-PDS, in terms of reduced effort for the training phase, as well as a more conservative approach w.r.t. users privacy, when handling conflicting access requests. We run several experiments on a realistic dataset exploiting a group of 360 evaluators. The obtained results show the effectiveness of the proposed approach.
更多查看译文
关键词
Training, Data privacy, Privacy, Memory, Data models, Semisupervised learning, Handheld computers, Personal data storage (PDS), active learning, personalized privacy preferences
AI 理解论文
溯源树
样例
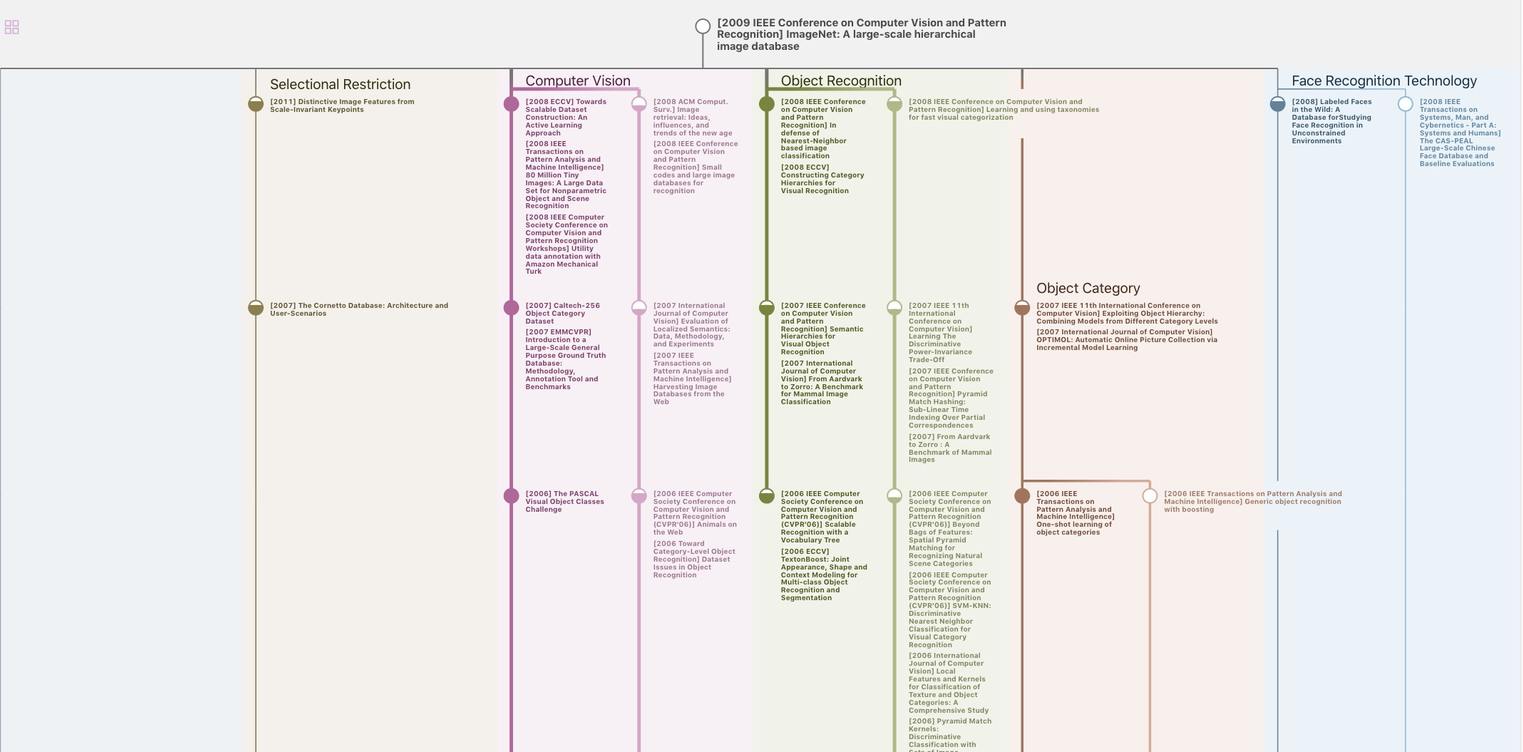
生成溯源树,研究论文发展脉络
Chat Paper
正在生成论文摘要