NDT-Transformer: Large-Scale 3D Point Cloud Localisation using the Normal Distribution Transform Representation
2021 IEEE INTERNATIONAL CONFERENCE ON ROBOTICS AND AUTOMATION (ICRA 2021)(2021)
摘要
3D point cloud-based place recognition is highly demanded by autonomous driving in GPS-challenged environments and serves as an essential component (i.e. loop-closure detection) in lidar-based SLAM systems. This paper proposes a novel approach, named NDT-Transformer, for real-time and large-scale place recognition using 3D point clouds. Specifically, a 3D Normal Distribution Transform (NDT) representation is employed to condense the raw, dense 3D point cloud as probabilistic distributions (NDT cells) to provide the geometrical shape description. Then a novel NDT-Transformer network learns a global descriptor from a set of 3D NDT cell representations. Benefiting from the NDT representation and NDT-Transformer network, the learned global descriptors are enriched with both geometrical and contextual information. Finally, descriptor retrieval is achieved using a query-database for place recognition. Compared to the state-of-the-art methods, the proposed approach achieves an improvement of 7.52% on average top 1 recall and 2.73% on average top 1% recall on the Oxford Robotcar benchmark.
更多查看译文
关键词
large-scale 3D point cloud localisation,3D point cloud-based place recognition,autonomous driving,GPS-challenged environments,loop-closure detection,lidar-based SLAM systems,large-scale place recognition,dense 3D point cloud,probabilistic distributions,NDT cells,geometrical shape description,novel NDT-Transformer network,global descriptor,3D NDT cell representations,NDT representation,learned global descriptors,3D normal distribution transform representation,descriptor retrieval
AI 理解论文
溯源树
样例
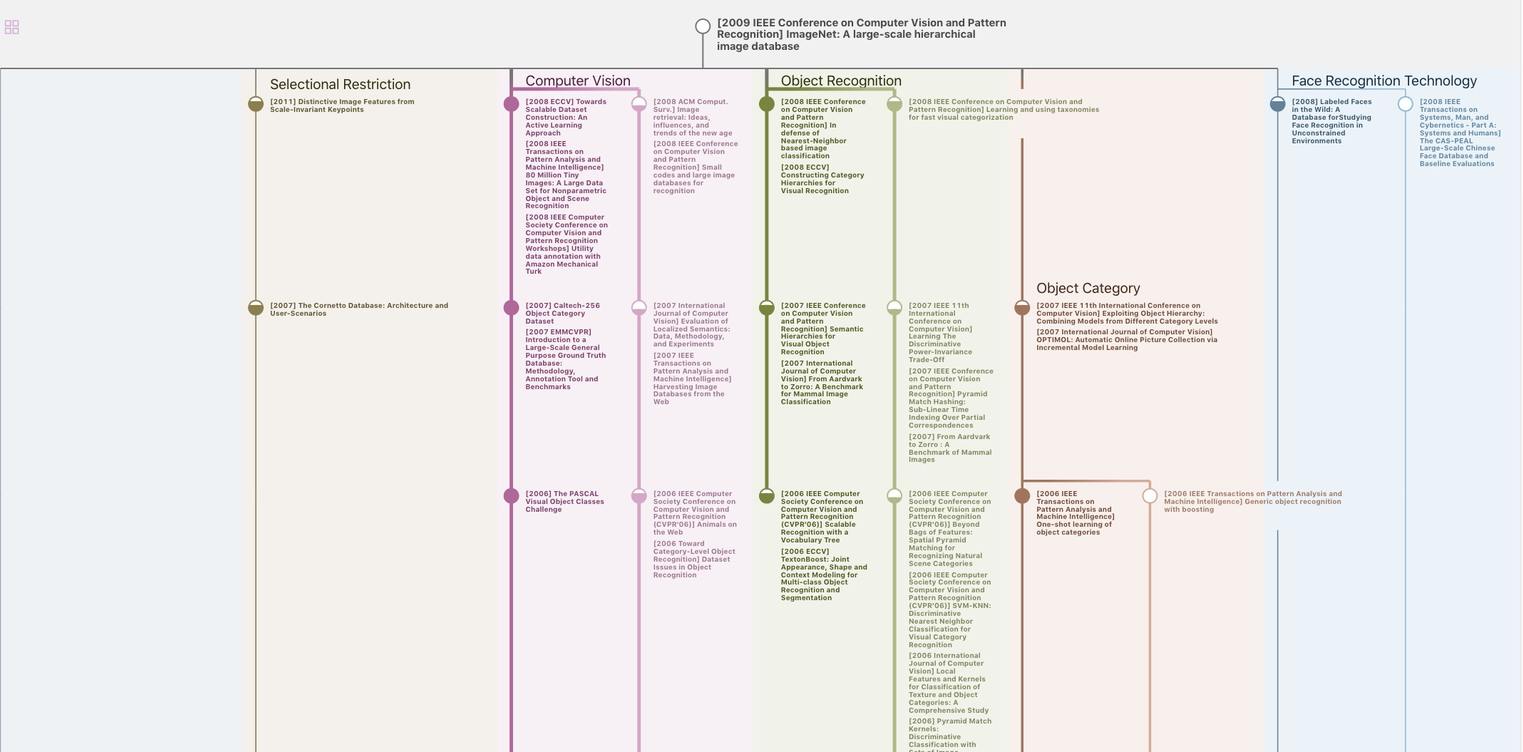
生成溯源树,研究论文发展脉络
Chat Paper
正在生成论文摘要