Dual-Channel Evidence Fusion for Fact Verification over Texts and Tables
North American Chapter of the Association for Computational Linguistics (NAACL)(2022)
摘要
Different from previous fact extraction and verification tasks that only consider evidence of a single format, FEVEROUS brings further challenges by extending the evidence format to both plain text and tables. Existing works convert all candidate evidence into either sentences or tables, thus often failing to fully capture the rich context in their original format from the converted evidence, let alone the context information lost during conversion. In this paper, we propose a Dual Channel Unified Format fact verification model (DCUF), which unifies various evidence into parallel streams, i.e., natural language sentences and a global evidence table, simultaneously. With carefully-designed evidence conversion and organization methods, DCUF makes the most of pre-trained table/language models to encourage each evidence piece to perform early and thorough interactions with other pieces in its original format. Experiments show that our model can make better use of existing pre-trained models to absorb evidence of two formats, thus outperforming previous works by a large margin. Our code and models are publicly available(1).
更多查看译文
关键词
fact verification,evidence,texts,fusion,dual-channel
AI 理解论文
溯源树
样例
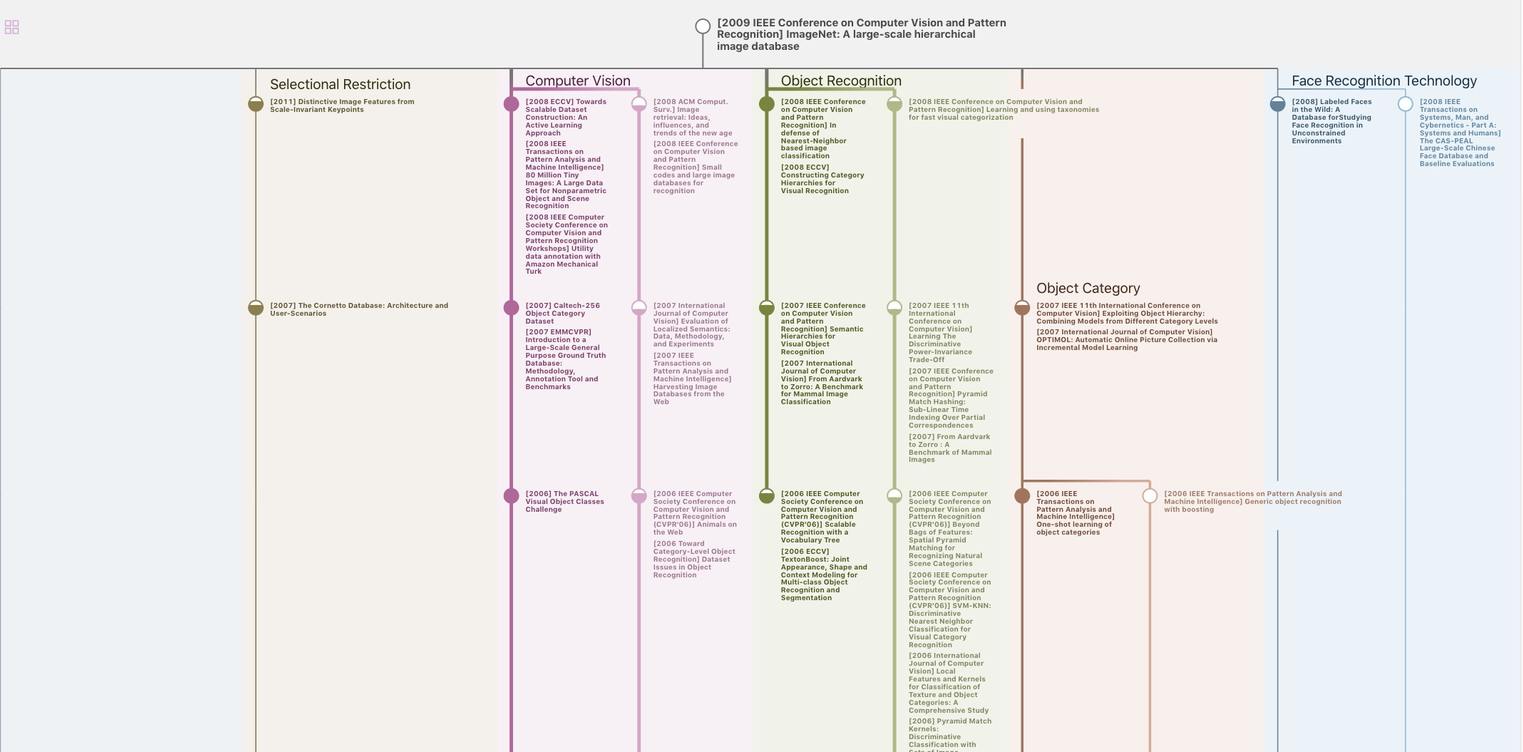
生成溯源树,研究论文发展脉络
Chat Paper
正在生成论文摘要