Federated Mixture of Experts
CoRR(2021)
Abstract
Federated learning (FL) has emerged as the predominant approach for collaborative training of neural network models across multiple users, without the need to gather the data at a central location. One of the important challenges in this setting is data heterogeneity; different users have different data characteristics. For this reason, training and using a single global model might be suboptimal when considering the performance of each of the individual user’s data. In this work, we tackle this problem via Federated Mixture of Experts, FedMix, a framework that allows us to train an ensemble of specialized models. FedMix adaptively selects and trains a user-specific selection of the ensemble members. We show that users with similar data characteristics select the same members and therefore share statistical strength while mitigating the effect of non-i.i.d data. Empirically, we show through an extensive experimental evaluation that FedMix improves performance compared to using a single global model while requiring similar or less communication costs.
MoreTranslated text
Key words
Artificial neural network,Machine learning,Computer science,Train,Artificial intelligence,Data heterogeneity,Federated learning,Global model,Mixture of experts,Statistical strength
AI Read Science
Must-Reading Tree
Example
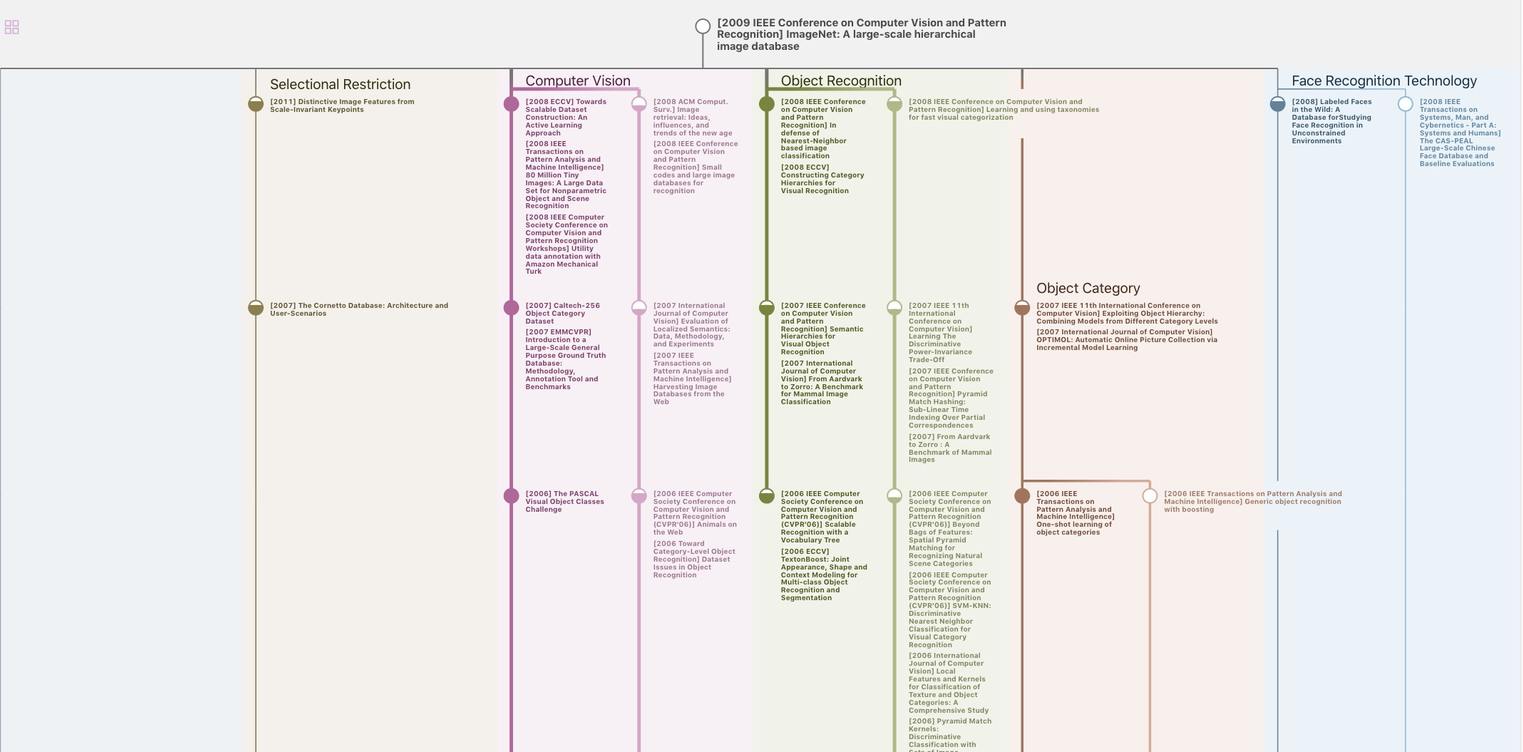
Generate MRT to find the research sequence of this paper
Chat Paper
Summary is being generated by the instructions you defined