Probabilistic Adaptive Control For Robust Behavior Imitation
IEEE ROBOTICS AND AUTOMATION LETTERS(2021)
摘要
In the context of learning from demonstration (LfD), trajectory policy representations such as probabilistic movement primitives (ProMPs) allow for rich modeling of demonstrated skills. To reproduce a learned skill with a real robot, a feedback controller is required to cope with perturbations and to react to dynamic changes in the environment. In this letter, we propose a generalized probabilistic control approach that merges the probabilistic modeling of the demonstrated movements and the feedback control action for reproducing the demonstrated behavior. We show that our controller can be easily employed, outperforming both original controller and a controller with constant feedback gains. Furthermore, we show that the proposed approach is able to solve dynamically changing tasks by modeling the demonstrated behavior as Gaussian mixtures and by introducing context variables. We demonstrate the capability of the approach with experiments in simulation and by teaching a 7-axis Franka Emika Panda robot to drop a ball into a moving box with only few demonstrations.
更多查看译文
关键词
Imitation learning, machine learning for robot control, robust/adaptive control
AI 理解论文
溯源树
样例
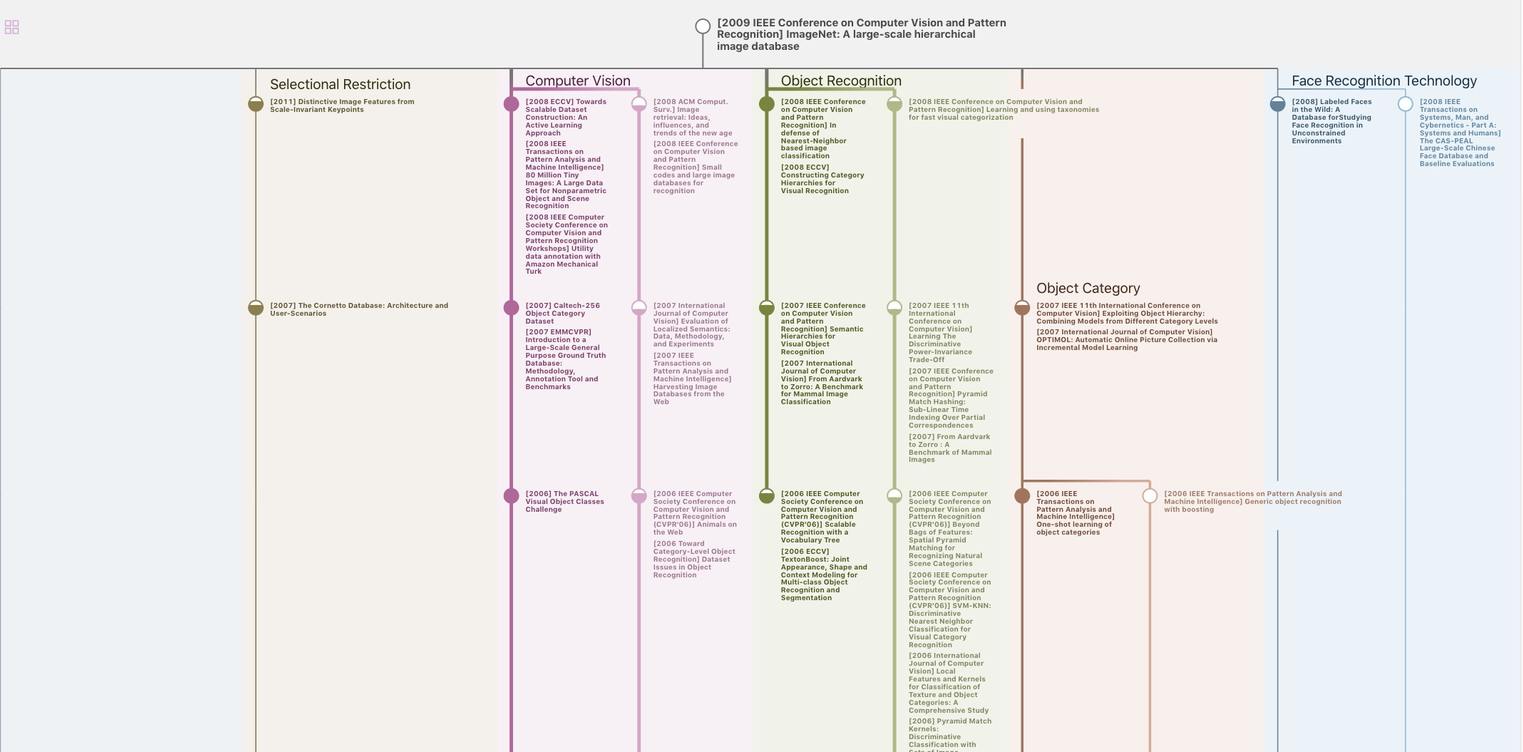
生成溯源树,研究论文发展脉络
Chat Paper
正在生成论文摘要