Analysis Based On Recent Deep Learning Approaches Applied In Real-Time Multi-Object Tracking: A Review
IEEE ACCESS(2021)
摘要
The deep learning technique has proven to be effective in the classification and localization of objects on the image or ground plane over time. The strength of the technique's features has enabled researchers to analyze object trajectories across multiple cameras for online multi-object tracking (MOT) systems. In the past five years, these technical features have gained a reputation in handling several real-time multiple object tracking challenges. This contributed to the increasing number of proposed deep learning methods (DLMs) and networks seen by the computer vision community. The technique efficiently handled various challenges in real-time MOT systems and improved overall tracking performance. However, it experienced difficulties in the detection and tracking of objects in overcrowded scenes and motion variations and confused appearance variations. Therefore, in this paper, we summarize and analyze the 95 contributions made in the past five years on deep learning-based online MOT methods and networks that rank highest in the public benchmark. We review their expedition, performance, advantages, and challenges under different experimental setups and tracking conditions. We also further categorize these methods and networks into four main themes: Online MOT Based Detection Quality and Associations, Real-Time MOT with High-Speed Tracking and Low Computational Costs, Modeling Target Uncertainty in Online MOT, and Deep Convolutional Neural Network (DCNN), Affinity and Data Association. Finally, we discuss the ongoing challenges and directions for future research.
更多查看译文
关键词
Target tracking, Real-time systems, Cameras, Deep learning, Object tracking, Feature extraction, Trajectory, Deep learning, detection quality, high-speed tracking, multi-camera object tracking, real-time tracking
AI 理解论文
溯源树
样例
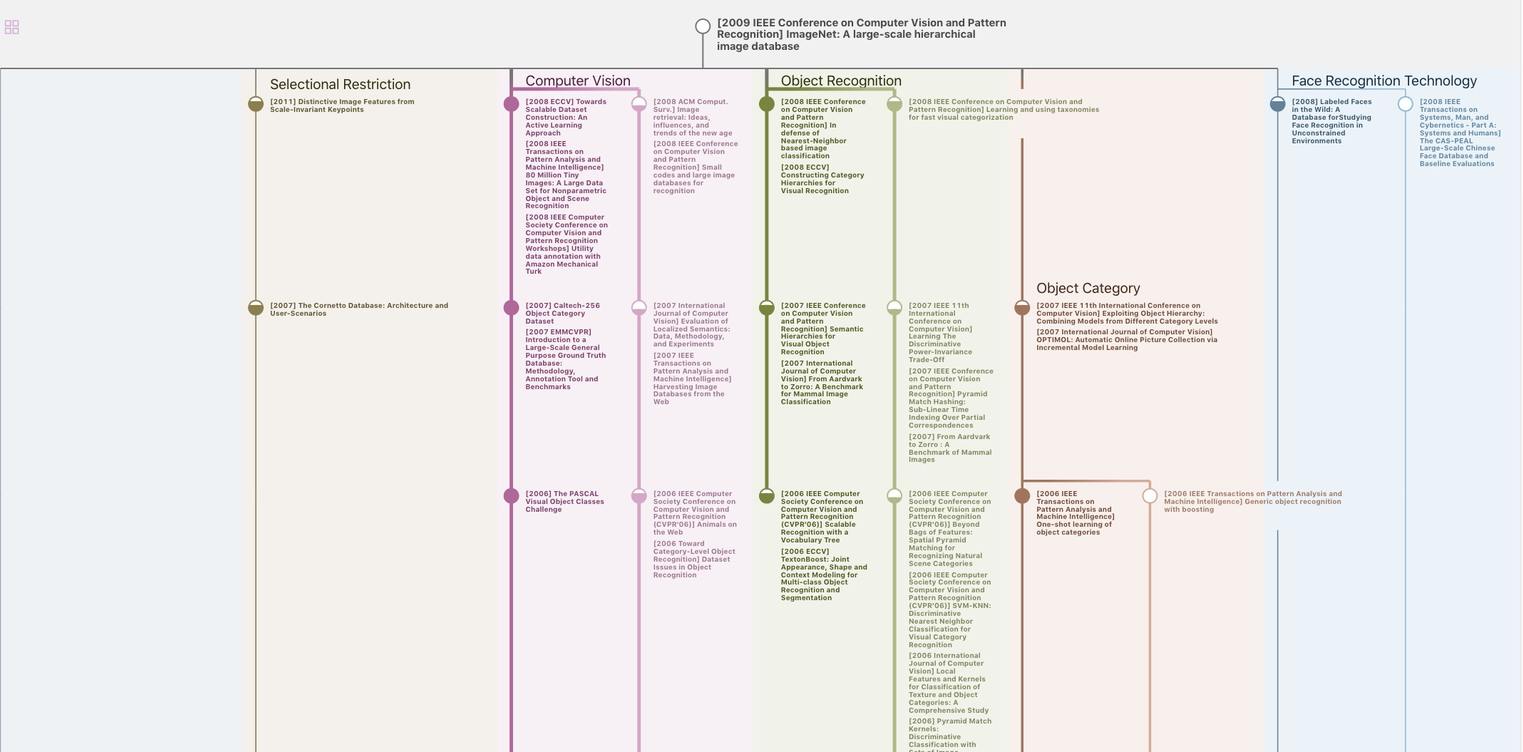
生成溯源树,研究论文发展脉络
Chat Paper
正在生成论文摘要