Intelliquench: An Adaptive Machine Learning System for Detection of Superconducting Magnet Quenches
IEEE TRANSACTIONS ON APPLIED SUPERCONDUCTIVITY(2021)
Abstract
In superconducting magnets, the irreversible transition of a portion of the conductor to resistive state is called a "quench." Having large stored energy, magnets can be damaged by quenches due to localized heating, high voltage, or large force transients. Unfortunately, current quench protection systems can only detect a quench after it happens, and mitigating risks in Low Temperature Superconducting (ITS) accelerator magnets often requires fast response (down to ms). Additionally, protection of High Temperature Superconducting (HTS) magnets is still suffering from prohibitively slow quench detection. In this study, we lay the groundwork for a quench prediction system using an auto-encoder fully-connected deep neural network. After dynamically trained with data features extracted from acoustic sensors around the magnet, the system detects anomalous events seconds before the quench in most of our data. While the exact nature of the events is under investigation, we show that the system can "forecast" a quench before it happens under magnet training conditions through a randomized experiment. This opens up the way of integrated data processing, potentially leading to faster and better diagnostics and detection of magnet quenches.
MoreTranslated text
Key words
Machine learning,online learning,quench detection,real-time system,superconducting magnets,quench
AI Read Science
Must-Reading Tree
Example
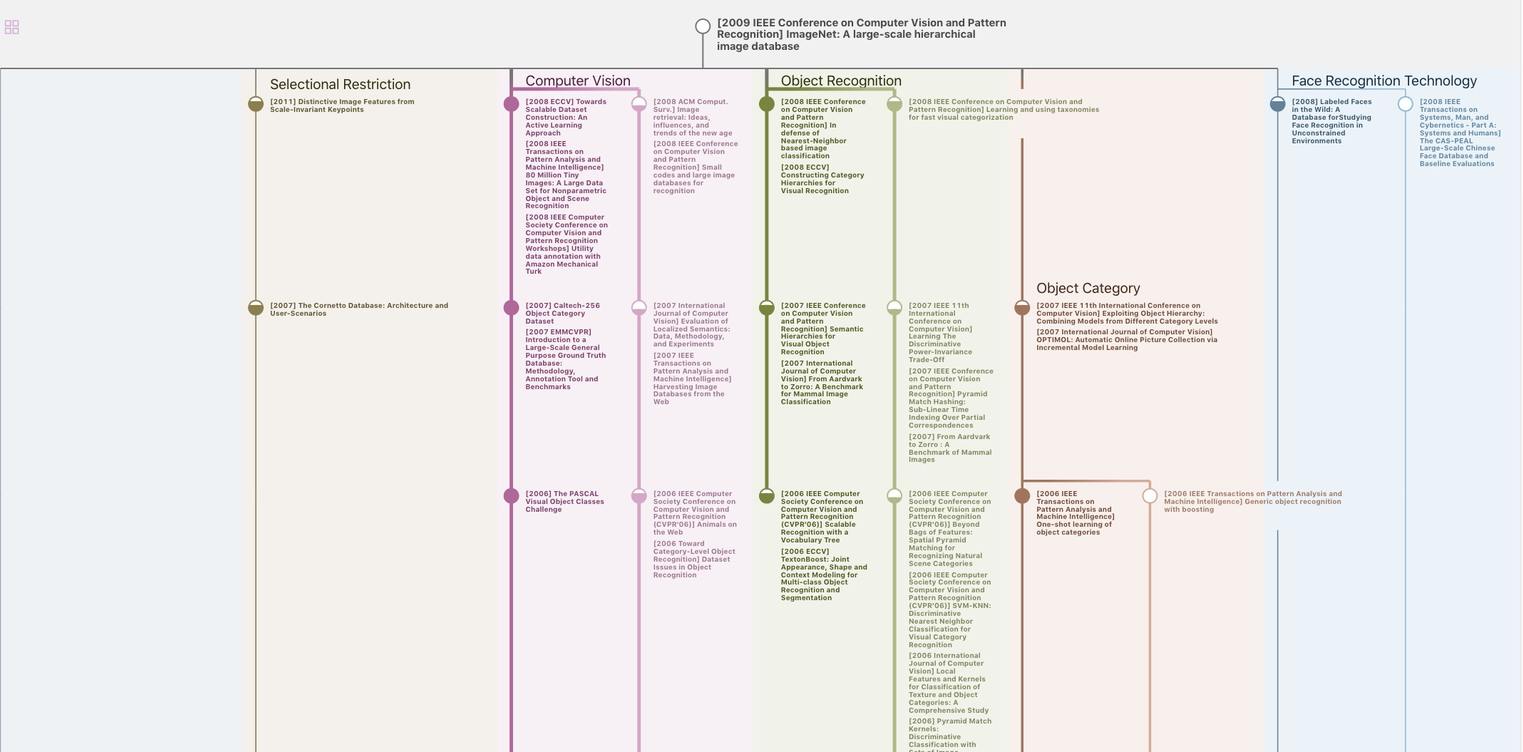
Generate MRT to find the research sequence of this paper
Chat Paper
Summary is being generated by the instructions you defined