Low-rank tensor completion based on non-convex logDet function and Tucker decomposition
SIGNAL IMAGE AND VIDEO PROCESSING(2021)
摘要
For the problem of low-rank tensor completion, rank estimation plays an extremely important role. And among some outstanding researches, nuclear norm is often used as a substitute of rank in the optimization due to its convex property. However, recent advances show that some non-convex functions could approximate the rank better, which can significantly improve the precision of the algorithm. While, the complexity of non-convex functions also leads to much higher computation cost, especially when the data are on a large scale. This paper proposes a mixture model for tensor completion by combining logDet function with Tucker decomposition, in which the logDet function is utilized as a much tighter rank approximation than the nuclear norm and the Tucker decomposition can significantly reduce the size of tensor that needs to be evaluated. In the implementation of the method, alternating direction method of multipliers is employed to obtain the optimal tensor completion. Several experiments are carried out to validate the effectiveness and efficiency of the method.
更多查看译文
关键词
Low-rank tensor completion, LogDet function, Tucker decomposition, Image recovery
AI 理解论文
溯源树
样例
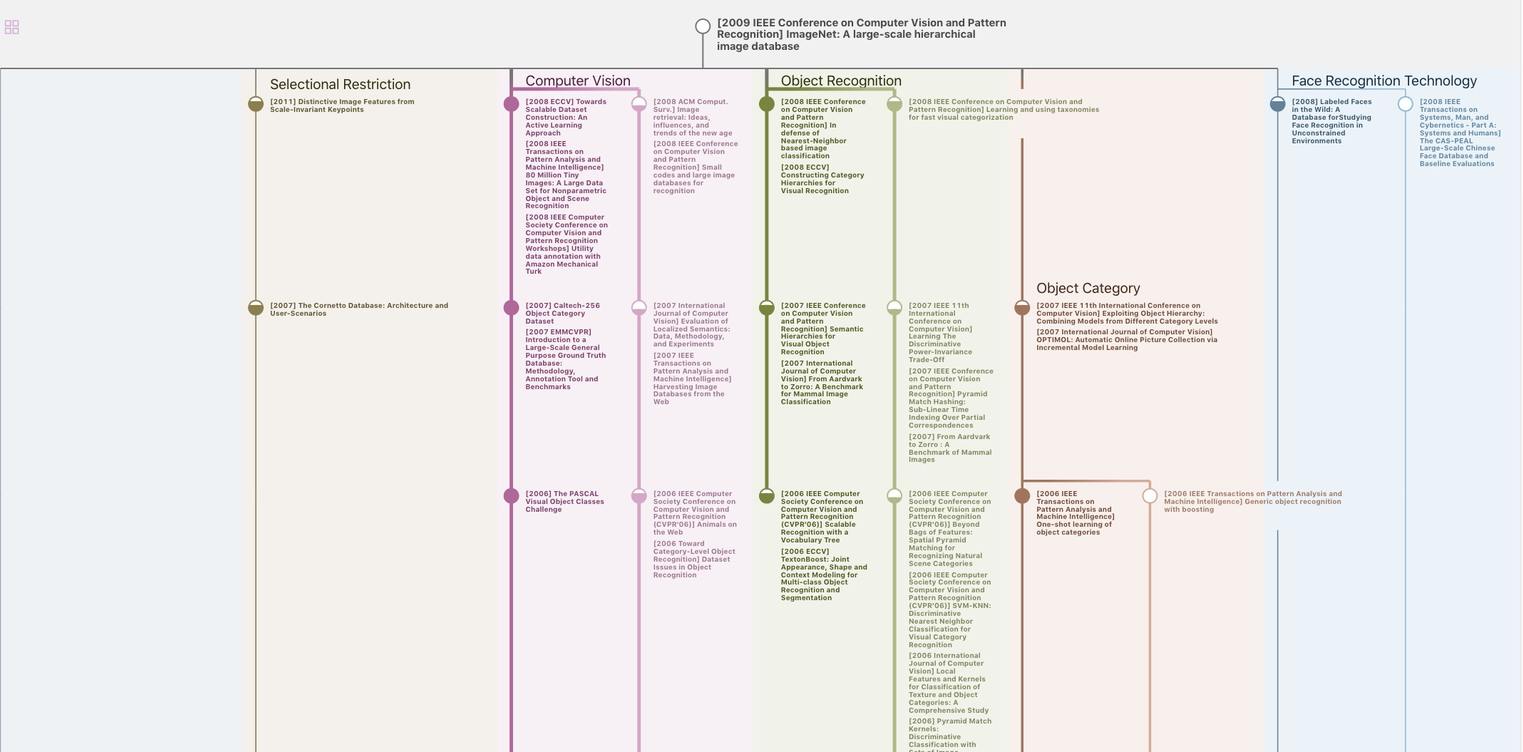
生成溯源树,研究论文发展脉络
Chat Paper
正在生成论文摘要