Multi-Objectivization Inspired Metaheuristics For The Sum-Of-The-Parts Combinatorial Optimization Problems
APPLIED SOFT COMPUTING(2021)
摘要
Multi-objectivization is a term used to describe strategies developed for optimizing single-objective problems by multi-objective algorithms. This paper focuses on multi-objectivizing the sum-of-the-parts combinatorial optimization problems, which include the traveling salesman problem, the unconstrained binary quadratic programming and other well-known combinatorial optimization problem. For a sum-of-the-parts combinatorial optimization problem, we propose to decompose its original objective into two sub-objectives with controllable correlation. Based on the decomposition method, two new multi-objectivization inspired single-objective optimization techniques called non-dominance search and non-dominance exploitation are developed, respectively. Non-dominance search is combined with two metaheuristics, namely iterated local search and iterated tabu search, while non-dominance exploitation is embedded within the iterated Lin-Kernighan metaheuristic. The resultant metaheuristics are called ILS+NDS, ITS+NDS and ILK+NDE, respectively. Empirical studies on some TSP and UBQP instances show that with appropriate correlation between the sub-objectives, there are more chances to escape from local optima when new starting solution is selected from the non-dominated solutions defined by the decomposed sub-objectives. Experimental results also show that ILS+NDS, ITS+NDS and ILK+NDE all significantly outperform their counterparts on most of the test instances. (C) 2021 Elsevier B.V. All rights reserved.
更多查看译文
关键词
Multi-objectivization, Local search, Combinatorial optimization, Traveling salesman problem
AI 理解论文
溯源树
样例
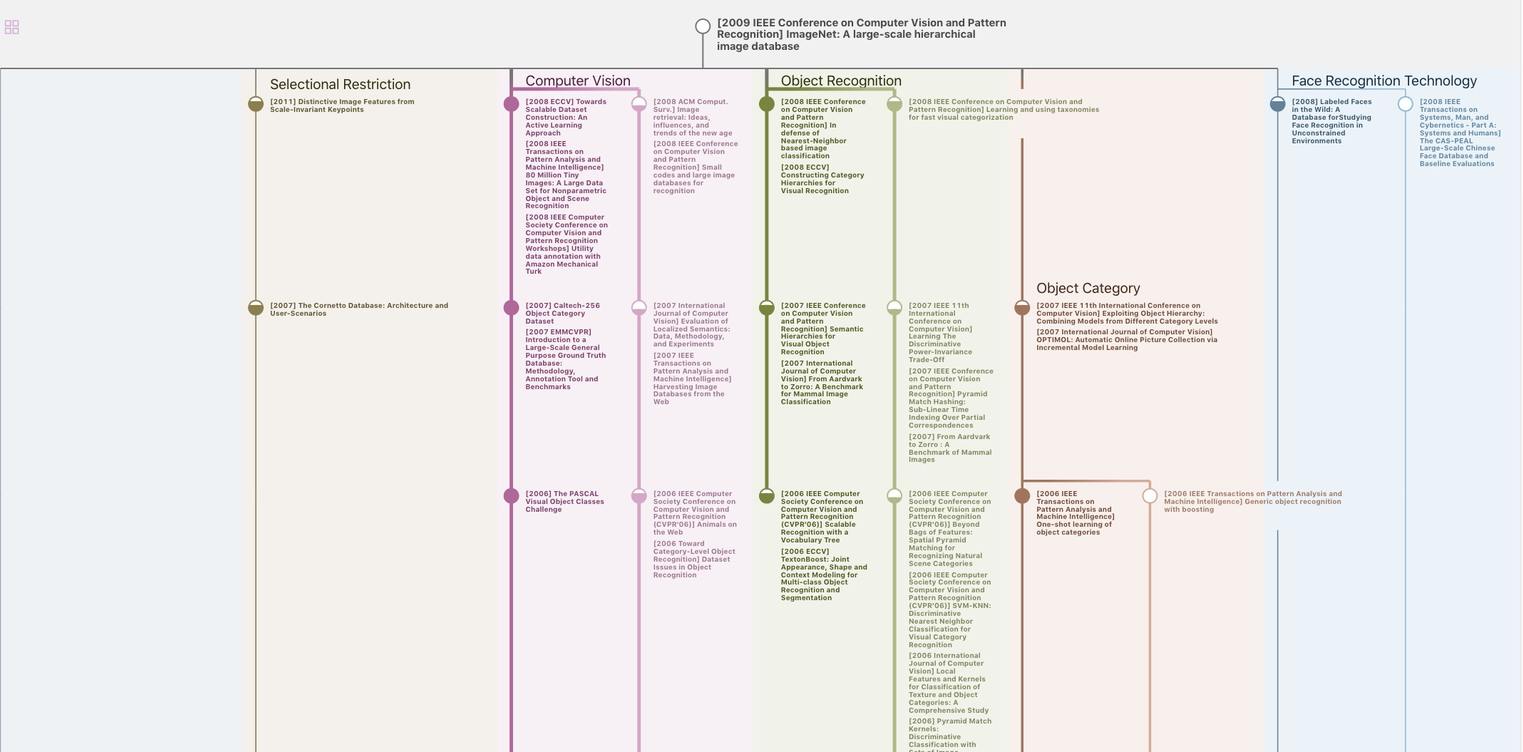
生成溯源树,研究论文发展脉络
Chat Paper
正在生成论文摘要