Deep Convolutional Neural Network Using Transfer Learning For Fault Diagnosis
IEEE ACCESS(2021)
摘要
Fault diagnosis is critical in industrial systems since early detection of problems can not only save valuable time but also reduce maintenance costs. The feature extraction process of traditional fault diagnosis is time-consuming and laborious work. Recently, with the rapid development of the deep learning (DL) method, it has shown its superiority with an end-to-end process and has been applied to classification and other fields. To a certain extent, it solves the disadvantages of manual feature extraction in the traditional fault diagnosis method. However, the available training data is often limited, and it will degrade the performance of DL methods. A new DL method that combines deep convolutional neural network (DCNN) and transfers learning (TL) for fault diagnosis is proposed in this paper to handle different fault types. A signal processing method that converts one-dimensional time-series signals into grayscale images is firstly applied, and it can eliminate the effect of handcrafted features. Secondly, an optimal DCNN is designed and trained with the ImageNet datasets, which can extract the high-level features of massive images. Finally, TL is further developed to apply the knowledge learned in the source data distribution to the target data distribution, which greatly reduces the dependence on training data and improves the generalization performance of DCNN. Three well-known datasets, including the bearing vibration dataset from the Case Western Reserve University (CWRU), self-priming centrifugal pump dataset (SPCP), and bearing force dataset from the University of Paderborn, are utilized to the performance of the proposed method. Some popular classification methods are also added to the comparison. Results show that the proposed method can precisely identify different fault types and have the highest classification accuracy among other methods.
更多查看译文
关键词
Feature extraction, Fault diagnosis, Convolution, Vibrations, Data mining, Kernel, Support vector machines, Fault diagnosis, deep convolutional neural network, transfer learning, image classification, signal processing
AI 理解论文
溯源树
样例
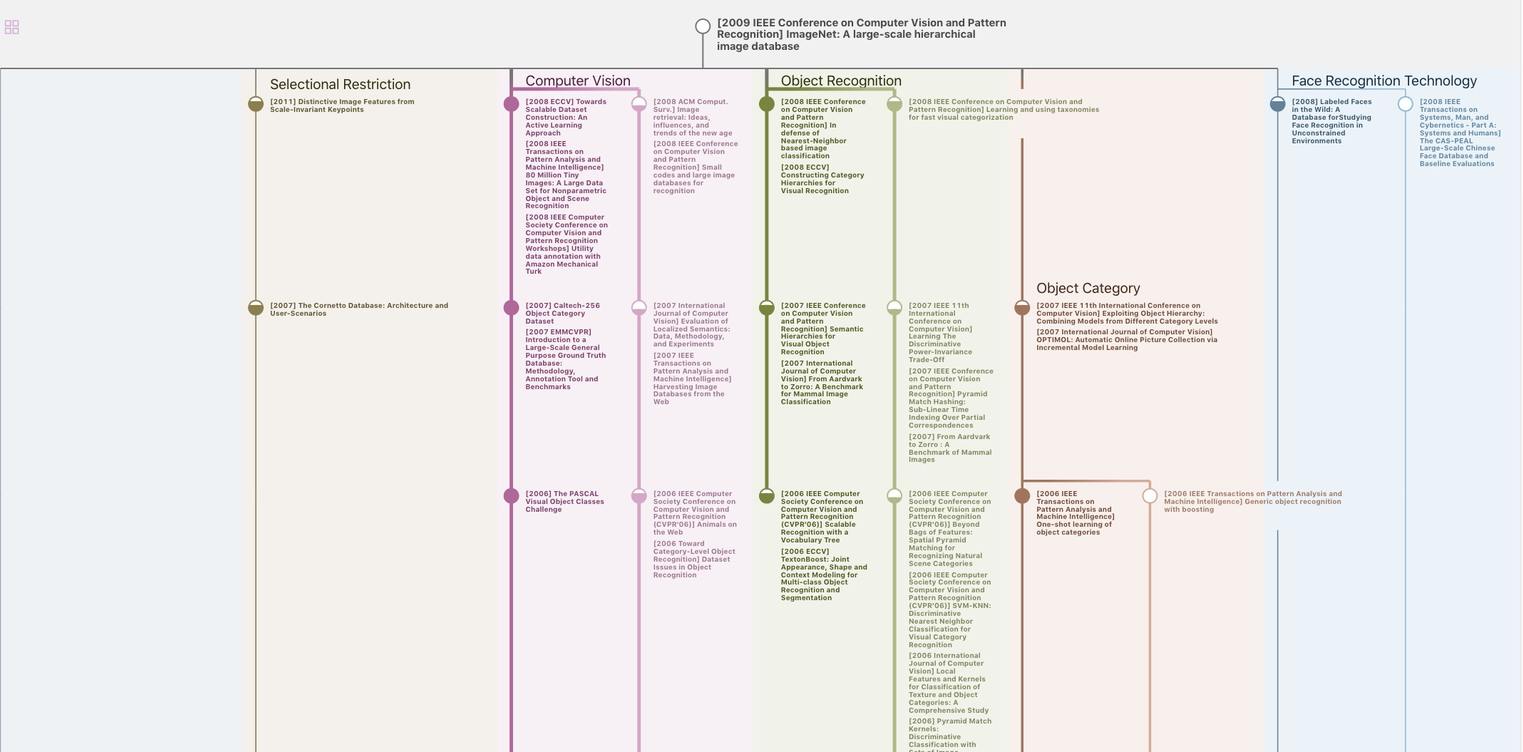
生成溯源树,研究论文发展脉络
Chat Paper
正在生成论文摘要