Classification Of Rock Fragments Produced By Tunnel Boring Machine Using Convolutional Neural Networks
AUTOMATION IN CONSTRUCTION(2021)
摘要
Rock fragmentation in tunnel boring machine (TBM) construction is an important indicator of tunnelling state. Large fragments often correspond to an unhealthy tunnelling state and may cause physical damage to belt conveyors. In this work, a convolutional neural network (CNN), AlexNet, was modified to perform image classification based on the volume of the largest fragment. First, all images were captured at the tunnel construction site. Second, a classification scheme was proposed according to the geological state of the site. After preprocessing raw images, different categories of images were labelled manually to train and evaluate CNN models. Finally, an optimised parameter setting for rock fragment recognition was carried out. Experimental results showed that the extracted features are reliable and can distinguish different types of images well. This competitive performance demonstrates that the proposed methodology can accurately detect large rock fragments by images at the site.
更多查看译文
关键词
Tunnel construction, Rock fragments, Deep learning, Convolutional neural network, AlexNet
AI 理解论文
溯源树
样例
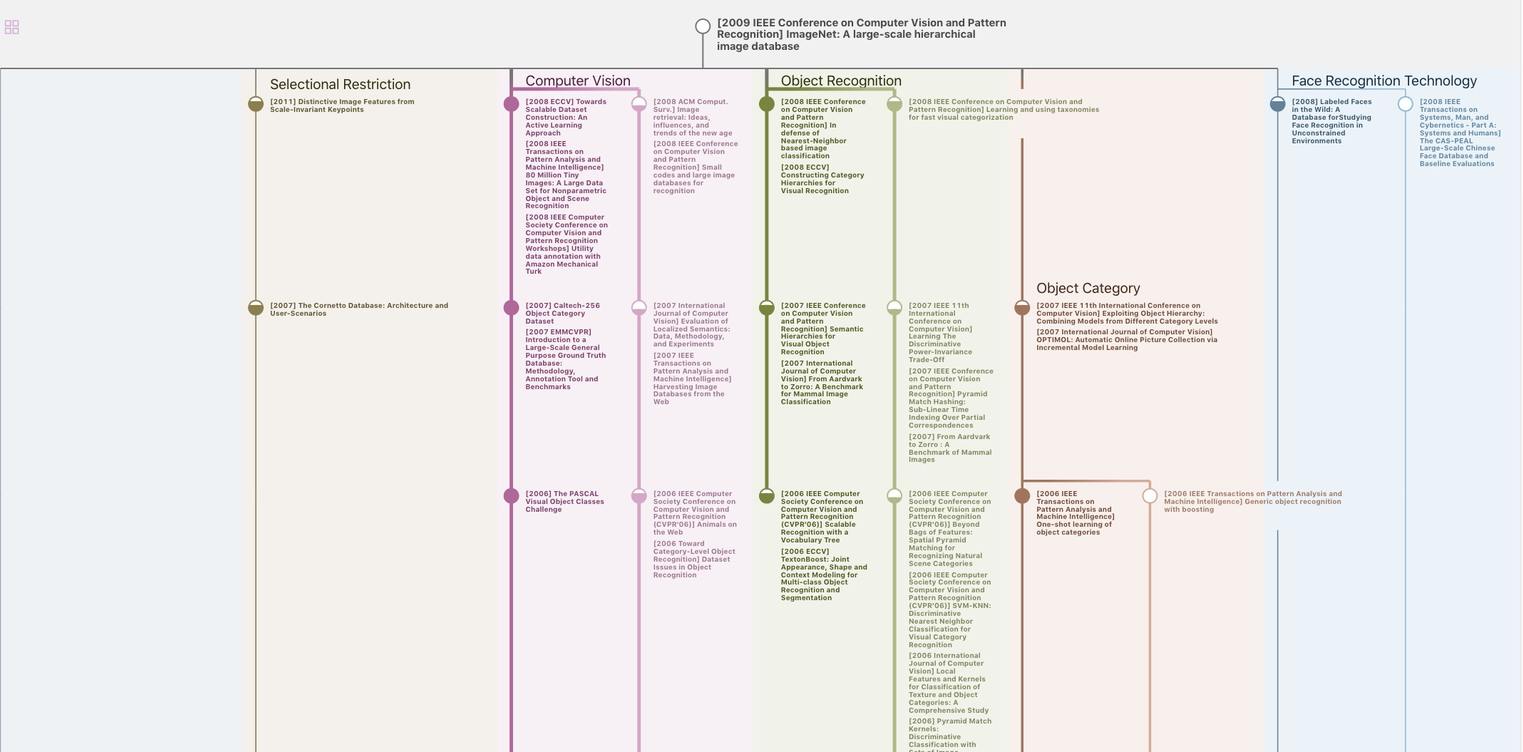
生成溯源树,研究论文发展脉络
Chat Paper
正在生成论文摘要