Efficient Training Of Energy-Based Models Via Spin-Glass Control
MACHINE LEARNING-SCIENCE AND TECHNOLOGY(2021)
摘要
We introduce a new family of energy-based probabilistic graphical models for efficient unsupervised learning. Its definition is motivated by the control of the spin-glass properties of the Ising model described by the weights of Boltzmann machines. We use it to learn the Bars and Stripes dataset of various sizes and the MNIST dataset, and show how they quickly achieve the performance offered by standard methods for unsupervised learning. Our results indicate that the standard initialization of Boltzmann machines with random weights equivalent to spin-glass models is an unnecessary bottleneck in the process of training. Furthermore, this new family allows for very easy access to low-energy configurations, which points to new, efficient training algorithms. The simplest variant of such algorithms approximates the negative phase of the log-likelihood gradient with no Markov chain Monte Carlo sampling costs at all, and with an accuracy sufficient to achieve good learning and generalization.
更多查看译文
关键词
unsupervised learning, Boltzmann machine, spin glass, statistical physics, physics-inspired machine learning
AI 理解论文
溯源树
样例
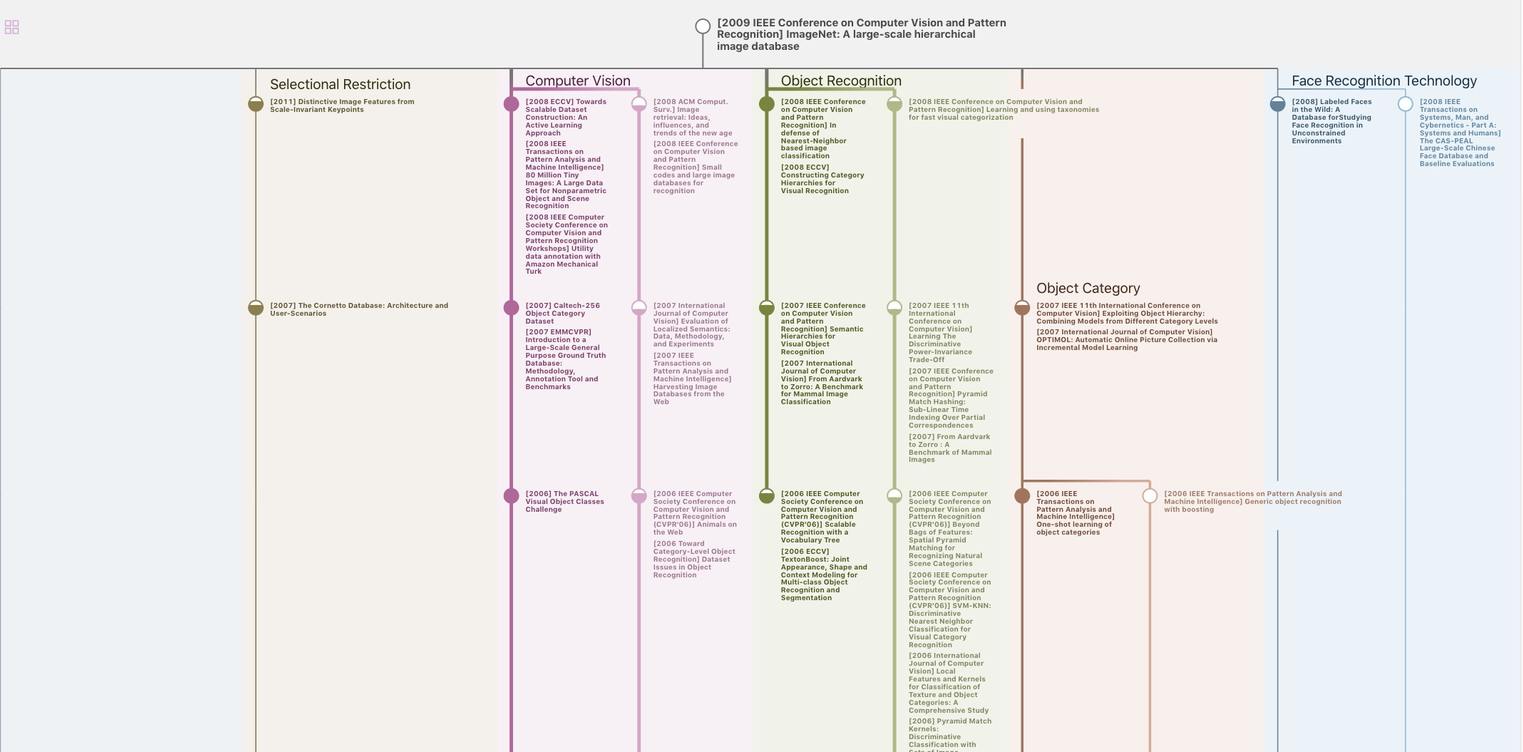
生成溯源树,研究论文发展脉络
Chat Paper
正在生成论文摘要