Investigating 3d Convolutional Layers As Feature Extractors For Anomaly Detection Systems Applied To Surveillance Videos
VISAPP: PROCEEDINGS OF THE 16TH INTERNATIONAL JOINT CONFERENCE ON COMPUTER VISION, IMAGING AND COMPUTER GRAPHICS THEORY AND APPLICATIONS - VOL. 5: VISAPP(2021)
摘要
Over the last few years, several strategies have been leveraged to detect unusual behavior in surveillance videos. Nonetheless, there are still few studies that compare strategies based on 3D Convolutional Neural Networks to tackle such problem. This research gap has motivated the this work in which we aim at investigating the features from a pre-trained C3D model and the training of fully 3D-convolutional auto-encoders for automated video anomaly detection systems, comparing them with respect to the anomaly detection performance and the processing power demands. Additionally, we present an auto-encoder model to detect anomalous behavior based on the pixel reconstruction error. While C3D features coming from the first layers were shown to be both better descriptors and faster to be computed, the auto-encoder achieved results comparable to the C3D, while requiring less computational effort. When compared to other studies using two benchmark datasets, the proposed methods are comparable to the state-of-the-art for the Ped2 dataset, while inferior when detecting anomalies on the Ped1 dataset. Additionally, our experimental results support the development of future 3D-CNN-based anomaly detection methods.
更多查看译文
关键词
Video Surveillance, Unusual Event Detection, Deep Learning
AI 理解论文
溯源树
样例
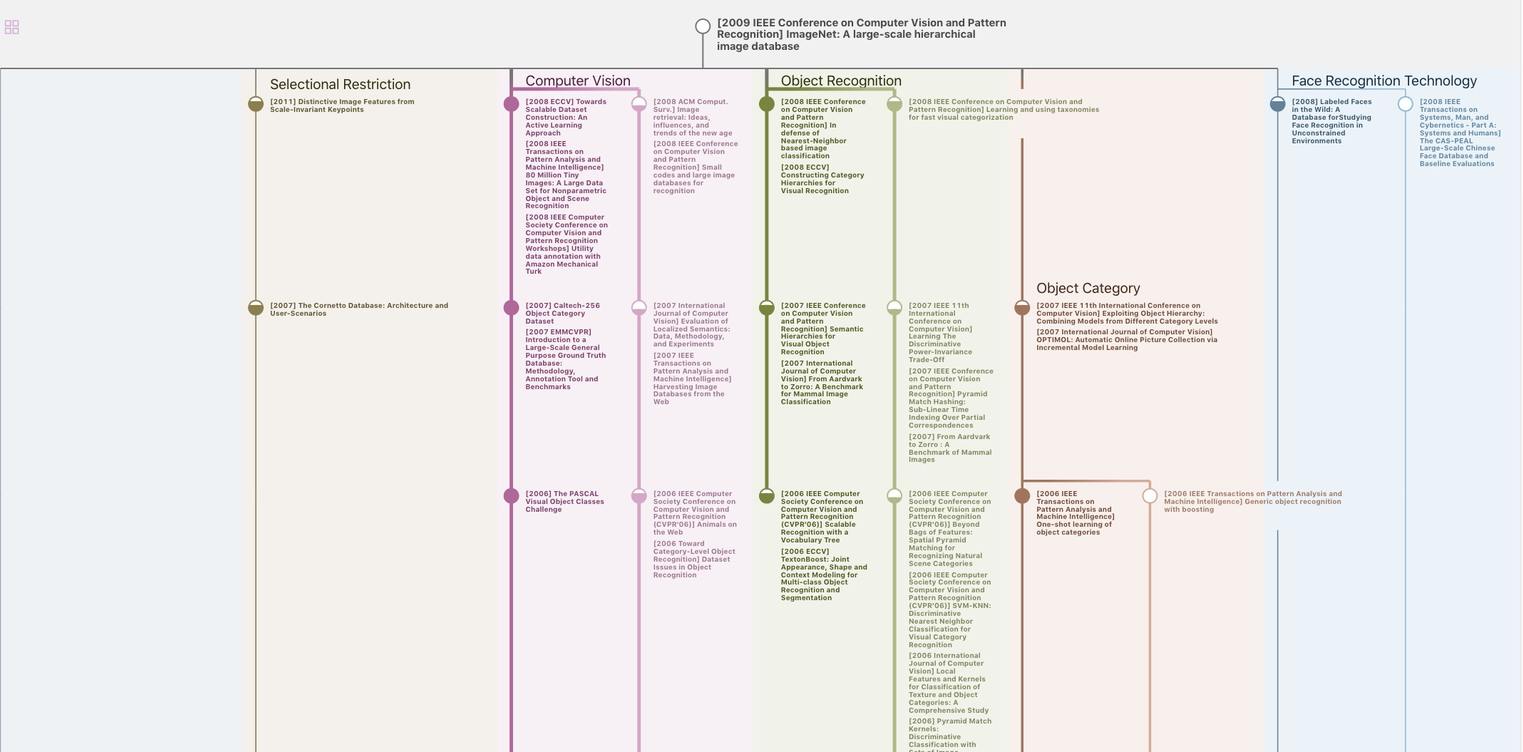
生成溯源树,研究论文发展脉络
Chat Paper
正在生成论文摘要