Demystifying The Use Of Era5-Land And Machine Learning For Wind Power Forecasting
IET RENEWABLE POWER GENERATION(2020)
摘要
Wind is a highly unstable renewable energy source. Accurate forecasting can mitigate the effects of wind inconsistency on the electric grid and help avoid investments in costly energy storage infrastructure. Basing the predictions on open-source forecast models and climate data also makes them entirely free of charge. The present work studies the feasibility of using two machine learning (ML) models and one deep learning (DL) model, random forest (RF) regression, support vector regression (SVR), and long short-term memory (LSTM) for short-term wind power forecasting based on the publicly accessible ERA5-Land dataset. For each forecast model, a selection of hyperparameters is first tuned, followed by determining the best performing input data structure using surrounding data grid points and increasing the time interval of data affecting a single prediction. Both the ML models and the DL model perform better than the baseline (BL) model when forecasting wind speed up to 24 hours ahead. However, a reduced forecast duration is needed to achieve satisfactory wind turbine (WT) power output forecast accuracy. Most notably, the RF is able to produce 3-hour forecasts with the combined WT power output prediction error amounting to less than 10 % of the WT's nominal power.
更多查看译文
关键词
wind power,support vector machines,regression analysis,wind turbines,load forecasting,power engineering computing,wind power plants,power system simulation,random forests,data structures,power grids,deep learning (artificial intelligence),renewable energy source,surplus energy,power production,energy storage,wind inconsistency,electric grid,low investment costs,open-source forecast models,open climate data,deep learning model,support vector regression,short-term memory,short-term wind power forecasting,ERA5-Land data,climate data,data structure,data grid points,reduced forecast duration,nominal power,WT power output prediction error,WT power output forecast,machine learning,random forest regression,long short-term memory,hyperparameter selection,baseline model forecasts,time 1,0 hour to 24,0 hour
AI 理解论文
溯源树
样例
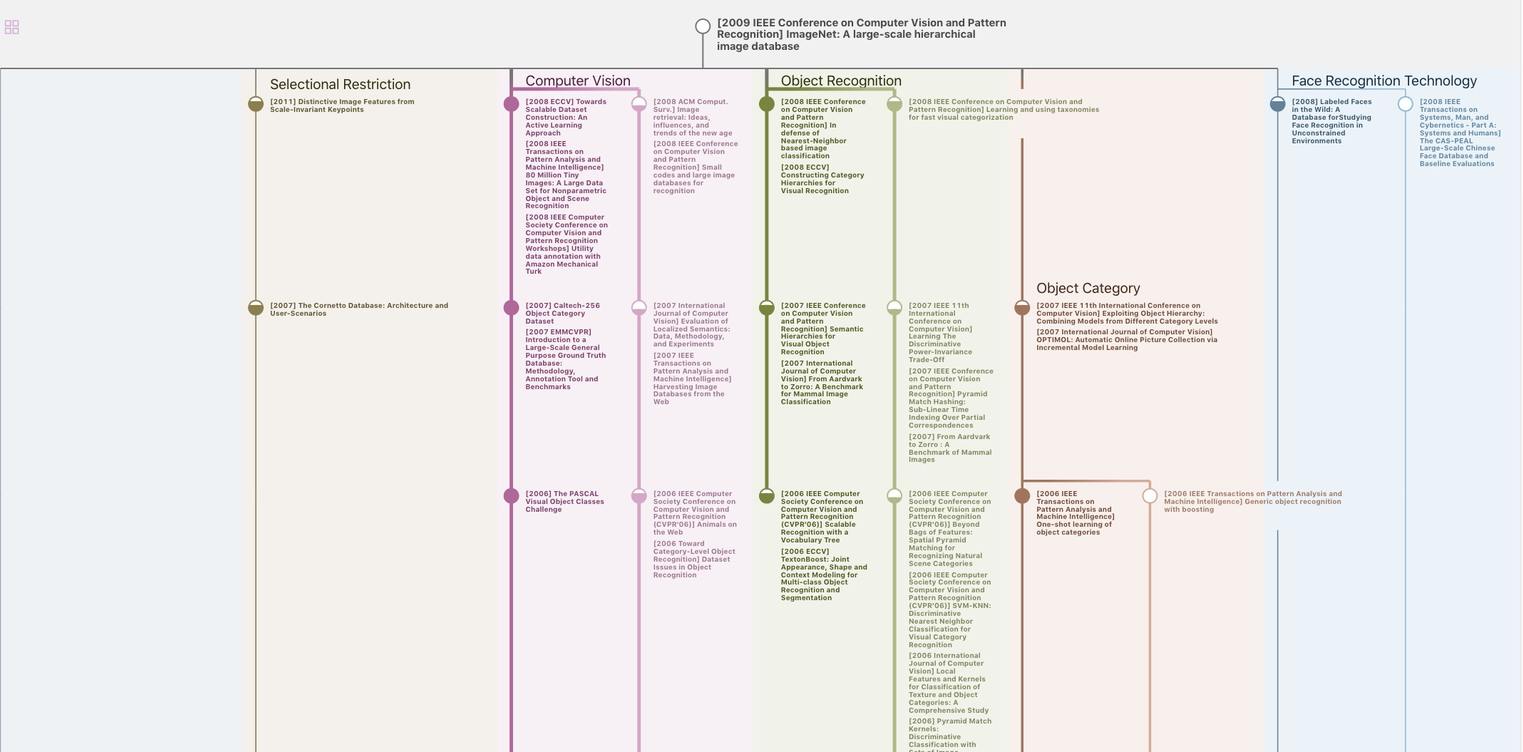
生成溯源树,研究论文发展脉络
Chat Paper
正在生成论文摘要