Reinforcement Learning-Based Routing Protocols For Vehicular Ad Hoc Networks: A Comparative Survey
IEEE ACCESS(2021)
摘要
Vehicular-ad hoc networks (VANETs) hold great importance because of their potentials in road safety improvement, traffic monitoring, and in-vehicle infotainment services. Due to high mobility, sparse connectivity, road-side obstacles, and shortage of roadside units, the links between the vehicles are subject to frequent disconnections; consequently, routing is crucial. Recently, to achieve more efficient routing, reinforcement learning (RL)-based routing algorithms have been investigated. RL represents a class of artificial intelligence that implements a learning procedure based on previous experiences and provides a better solution for future operations. RL algorithms are more favorable than other optimization techniques owing to their modest usage of memory and computational resources. Because a VANET deals with passenger safety, any kind of flaw is intolerable in VANET routing. Fortunately, RL-based algorithms have the potentials to optimize the different quality-of-service parameters of VANET routing such as bandwidth, end-to-end delay, throughput, control overhead, and packet delivery ratio. However, to the best of the authors' knowledge, surveys on RL-based routing protocols for VANETs have not been conducted. To fulfill this gap in the literature and to provide future research directions, it is necessary to aggregate the scattered works on this topic. This study presents a comparative investigation of RL-based routing protocols, by considering their working procedure, advantages, disadvantages, and applications. They are qualitatively compared in terms of key features, characteristics, optimization criteria, performance evaluation techniques, and implemented RL techniques. Lastly, open issues and research challenges are discussed to make RL-based VANET routing protocols more efficient in the future.
更多查看译文
关键词
Vehicular ad hoc networks, Routing, Routing protocols, Quality of service, Reinforcement learning, Optimization, Safety, Vehicular ad hoc network, routing protocol, reinforcement learning, Q-learning, intelligent algorithm, quality-of-service routing, intelligent transportation system
AI 理解论文
溯源树
样例
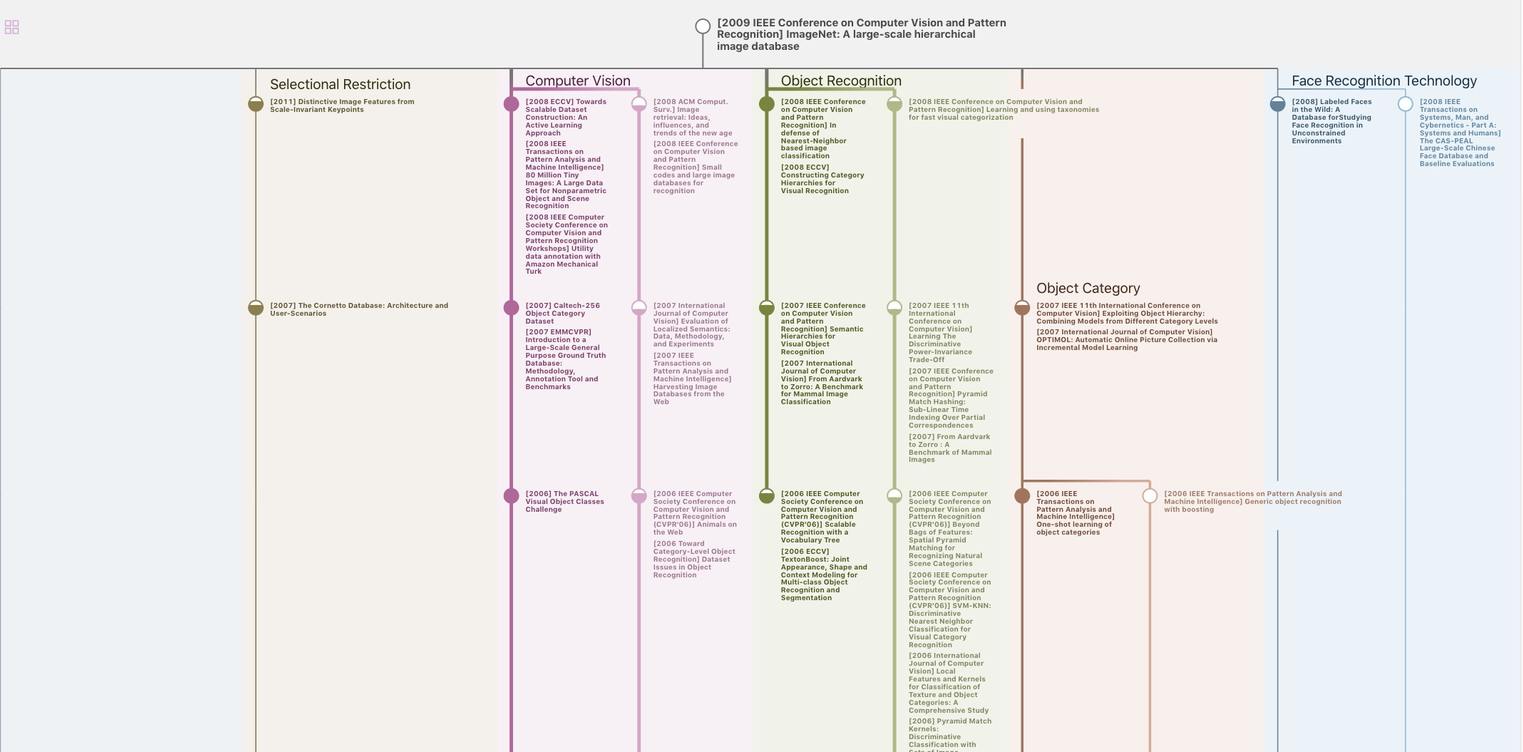
生成溯源树,研究论文发展脉络
Chat Paper
正在生成论文摘要