Skeleton-Based Square Grid For Human Action Recognition With 3d Convolutional Neural Network
IEEE ACCESS(2021)
摘要
Convolutional neural networks (CNNs) can effectively handle grid-structured data but not dynamic skeletons, which are usually expressed as graph structures. In this study, we first propose a skeleton-based square grid (SSG) for transforming dynamic skeletons into three-dimensional (3D) grid-structured data so that CNNs can be applied to such data. Each SSG contains a joint-based square grid (JSG) and a rigid-based square grid (RSG) based on intrinsic and extrinsic dependencies of various body parts, respectively. Next, to enhance the ability of deep features to capture the correlations among 3D grid-structured data, a two-stream 3D CNN is constructed to learn spatiotemporal features using the JSG and RSG sequences. Finally, we introduce a soft attention model that selectively focuses on the informative body parts in the skeleton sequences. We validate our model in terms of action recognition using three datasets: NTU RGB+D, Kinetics Motion, and SBU Kinect Interaction datasets. Our experimental results demonstrate the effectiveness of the proposed approach as well as its superior performance when compared with those of state-of-the-art methods.
更多查看译文
关键词
Skeleton, Three-dimensional displays, Spatiotemporal phenomena, Feature extraction, Convolution, Kernel, Convolutional neural networks, 3D convolutional neural networks, skeleton action recognition, neural network, attention mechanism
AI 理解论文
溯源树
样例
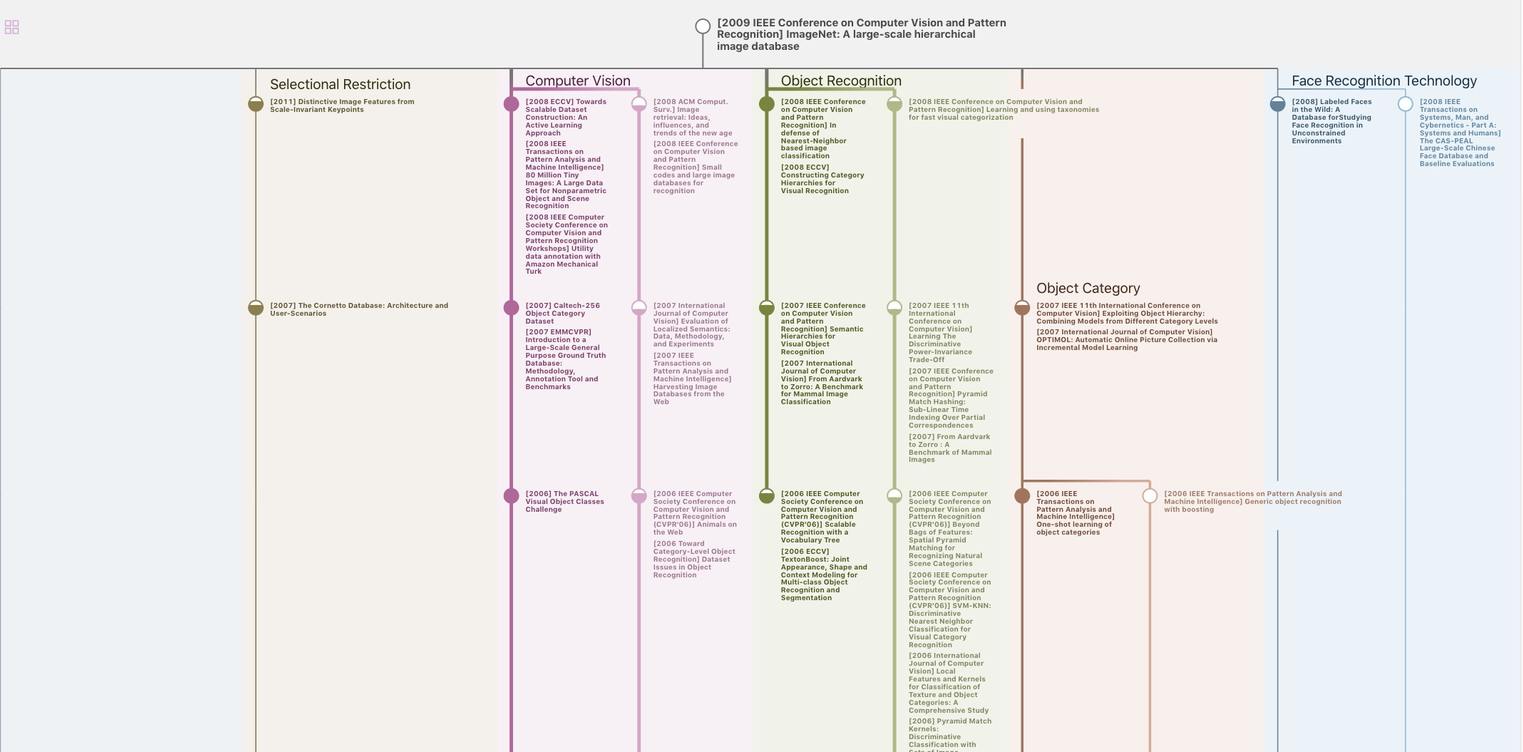
生成溯源树,研究论文发展脉络
Chat Paper
正在生成论文摘要