Attention Aware and Multiple Granularity 3D Convolutional Neural Networks for Lung Nodule Classification on CT Image
2020 IEEE 5th International Conference on Signal and Image Processing (ICSIP)(2020)
摘要
The 3D convolutional neural network (CNN) is able to make full use of the spatial 3D context information of lung nodules. In this paper, a novel 3D CNN is proposed to classify lung nodule. To make the feature map towards the most informative components of an input, spatial attention and channel attention are integrated with the 3D CNN backbone. To combine global and local information, a multiple granularity architecture is designed. We conduct a binary classification (benign and malignant) on two computed tomography (CT) datasets (public Lung Image Database Consortium and Image Database Resource Initiative database (LIDC-IDRI) and private LIDC-TX dataset). It achieves a sensitivity of 0.962, specificity of 0.942 and error rate of 0.048 on LIDC-IDRI database. For the private LIDC-TX dataset, sensitivity, specificity and error rate are 0.718, 0.627 and 0.327, respectively. Experimental results demonstrate that the proposed method get a promising result compared with existing lung nodule classification methods.
更多查看译文
关键词
3D CNN,attention module,multiple granularity architecture,lung nodule classification
AI 理解论文
溯源树
样例
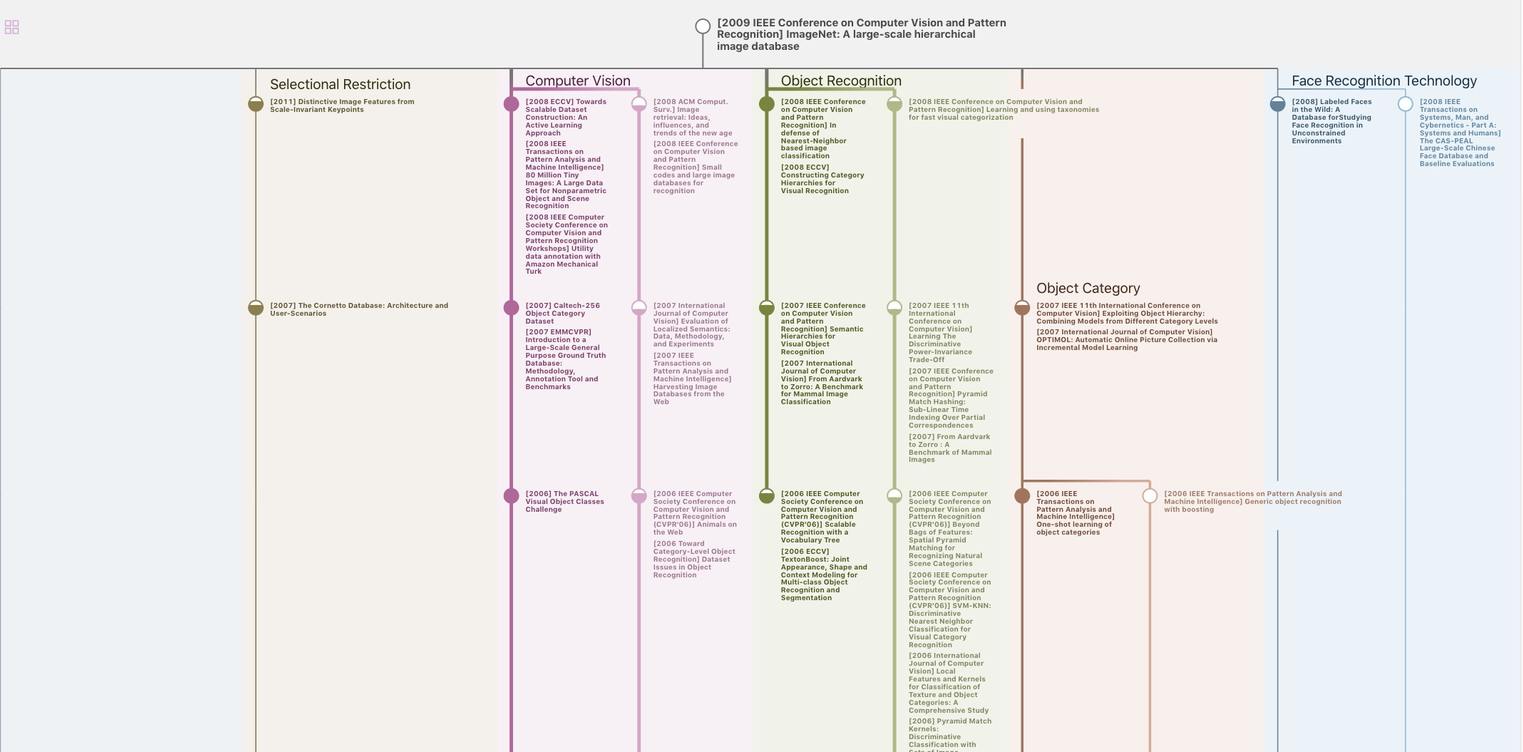
生成溯源树,研究论文发展脉络
Chat Paper
正在生成论文摘要