Research of Fault Feature Extraction and Analysis Method Based on Aeroengine Fault Data
chinese automation congress(2020)
Abstract
Research of engine fault diagnosis is an effective way to ensure the safety of aviation aircraft. The original gas path fault information of turboshaft engines and turboprop engines often introduces a lot of noise during the collection process, which interferes with the extraction of fault features. Therefore, it is necessary to develop fault feature extraction algorithms adapted to the needs of big data processing to solve such problems. In this paper, the C-MAPSS turbofan engine degradation simulation data set is used as the research object. After using Principal components analysis (PCA) for data dimensionality reduction, two algorithms of k-means clustering and DBSCAN clustering are used to extract fault features of the data. The Silhouette Coefficients and DBI internal evaluation indicators of the two methods are analyzed. In order to improve the effect of clustering, a fault feature extraction algorithm with better performance is proposed: DBSCAN k-means Mixing (DKMM) algorithm improves the accuracy of the clustering algorithm. Finally, the fault feature extraction software for turboshaft engines and turboprop engines was developed. The research results of this paper can provide theoretical support for the development of the two-machine special health management system.
MoreTranslated text
Key words
Feature extraction, clustering algorithm, fault feature, turboshaft engine, turboprop engine
AI Read Science
Must-Reading Tree
Example
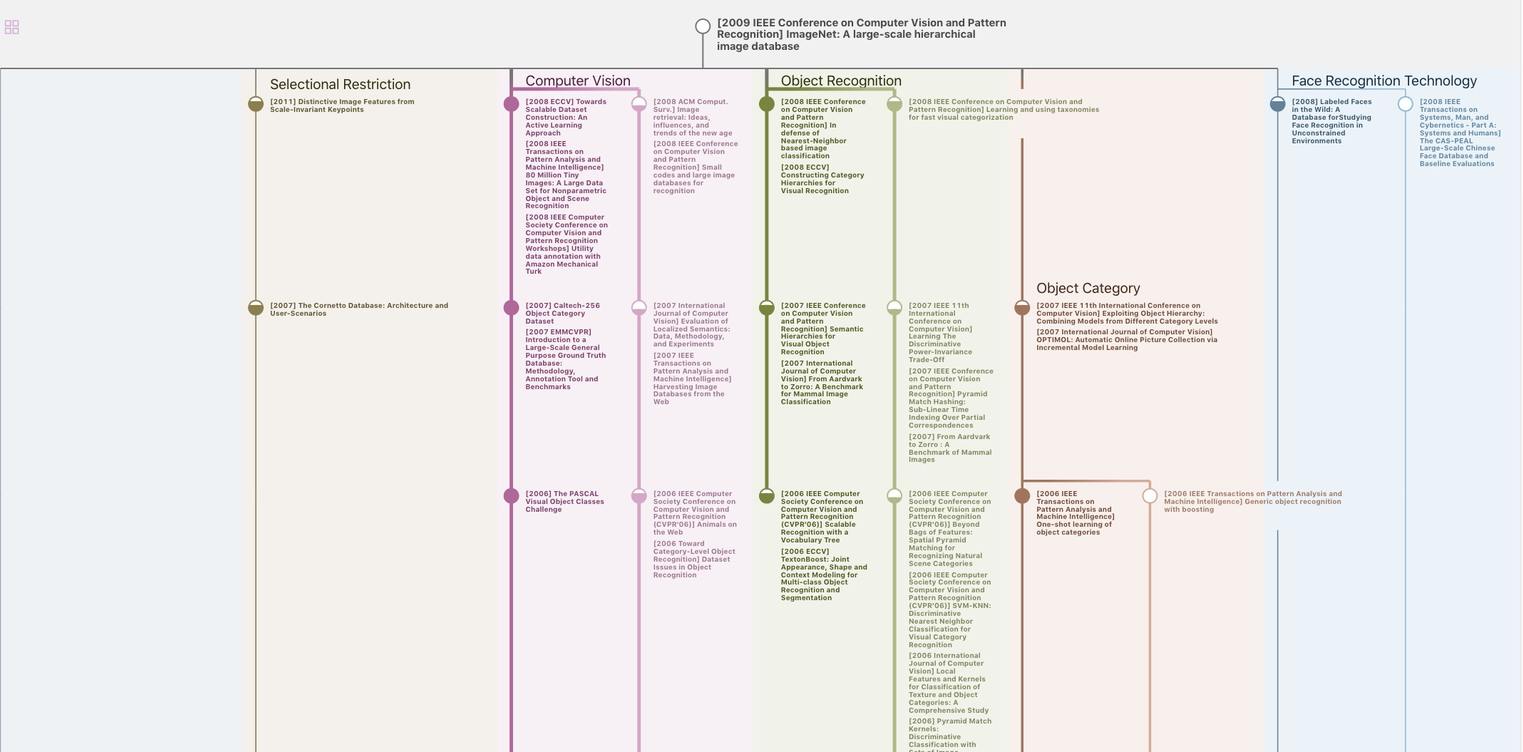
Generate MRT to find the research sequence of this paper
Chat Paper
Summary is being generated by the instructions you defined