A Fault Diagnosis Method For Rolling Bearings Based On Parameter Transfer Learning Under Imbalance Data Sets
ENERGIES(2021)
摘要
Fault diagnosis under the condition of data sets or samples with only a few fault labels has become a hot spot in the field of machinery fault diagnosis. To solve this problem, a fault diagnosis method based on deep transfer learning is proposed. Firstly, the discriminator of the generative adversarial network (GAN) is improved by enhancing its sparsity, and then adopts the adversarial mechanism to continuously optimize the recognition ability of the discriminator; finally, the parameter transfer learning (PTL) method is applied to transfer the trained discriminator to target domain to solve the fault diagnosis problem with only a small number of label samples. Experimental results show that this method has good fault diagnosis performance.
更多查看译文
关键词
fault diagnosis, rolling bearings, unbalance samples, deep transfer learning
AI 理解论文
溯源树
样例
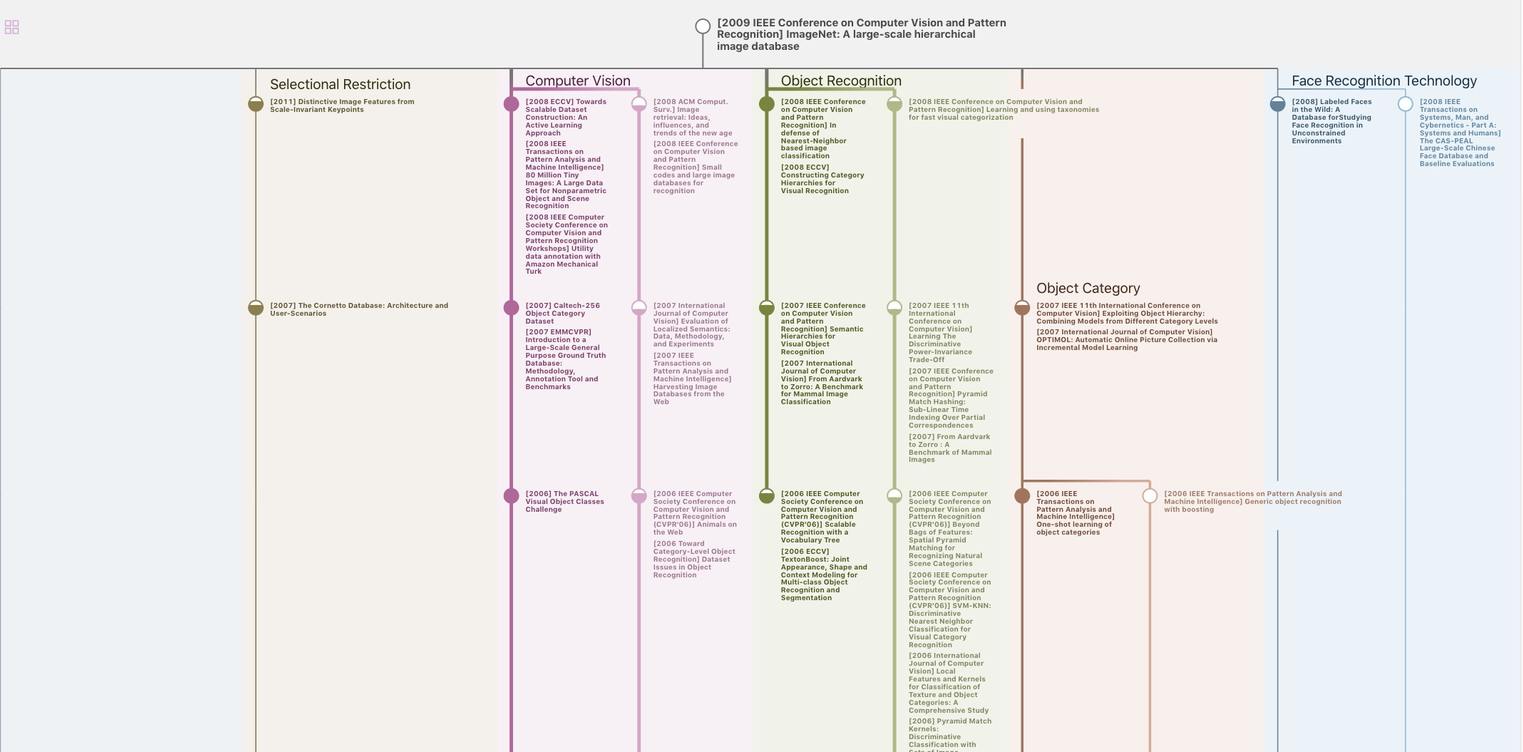
生成溯源树,研究论文发展脉络
Chat Paper
正在生成论文摘要