Style Transfer For Keypoint Matching Under Adverse Conditions
2020 INTERNATIONAL CONFERENCE ON 3D VISION (3DV 2020)(2020)
摘要
In this work, we address the difficulty of matching local features between images captured at distant points in time resulting in a global appearance change. Inspired by recent neural style transfer techniques, we propose to use an image transformation network to translate night images into day-like appearance, with the objective of better matching performance. We extend traditional style transfer, that optimize for content and style, with a keypoint matching loss function. The joint optimization of these losses allows our model to generate images that can significantly improve the performance of local feature matching, in a self-supervised way. As a result, our approach is flexible and does not require paired training data, which is difficult to obtain in practice. We show how our method can be used as an extension to a state-of-the-art differentiable feature extractor to improve its performance in challenging scenarios. This is demonstrated in our evaluation on day-night image matching and visual localization tasks with night-rain image queries.
更多查看译文
AI 理解论文
溯源树
样例
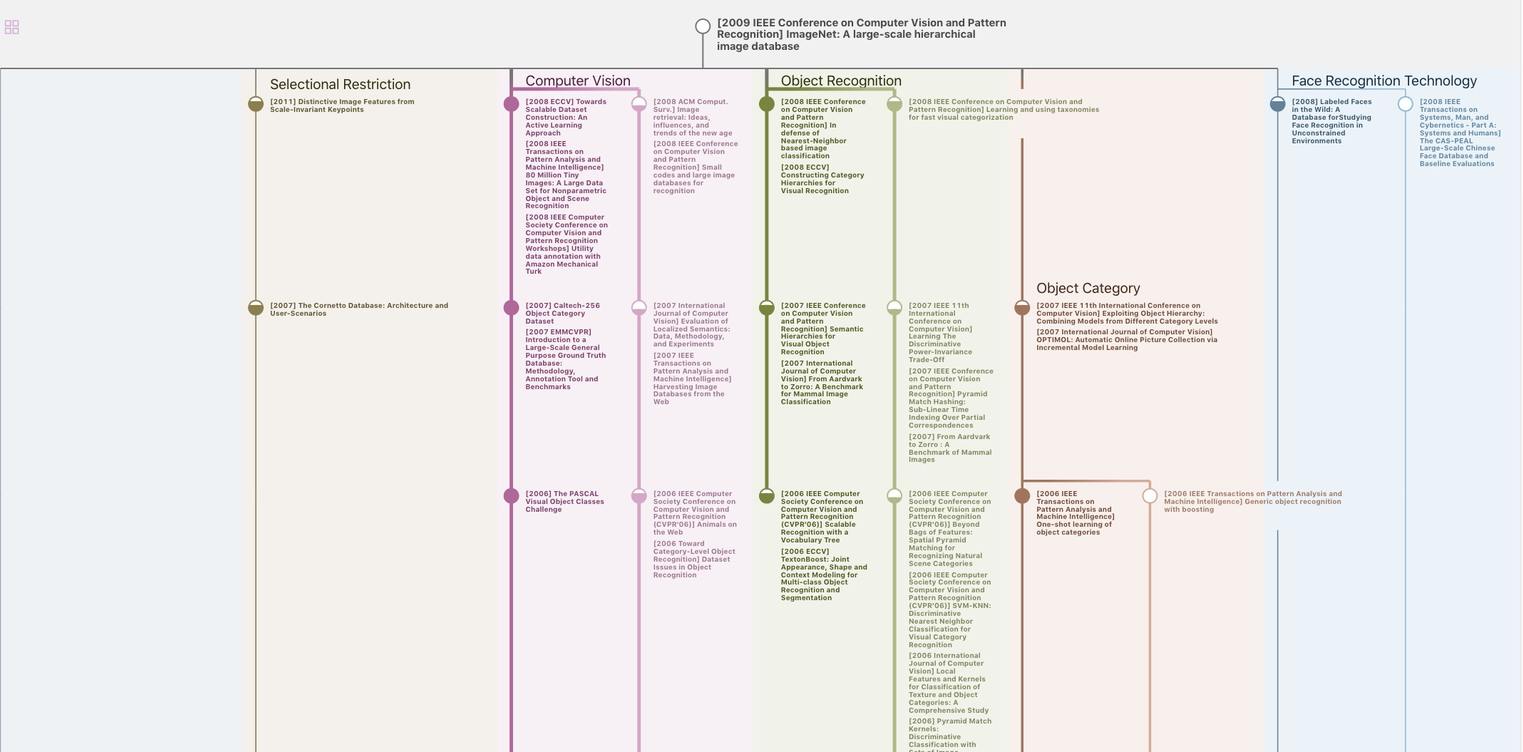
生成溯源树,研究论文发展脉络
Chat Paper
正在生成论文摘要