Neural Network-Based Photovoltaic Generation Capacity Prediction System With Benefit-Oriented Modification
ENERGY(2021)
摘要
Photovoltaic (PV) generation prediction is a critical technology for integrating solar energy in power systems and markets. Accuracy is the target for most PV prediction models, which represents the minimisation of the average error. However, minimisation of prediction error is to obtain a minimum cost from impact of prediction inaccuracy. The lowest average error may not always relate to the minimum cost. Thus, this paper proposes an integrated PV prediction structure that targets minimum industrial cost from prediction error other than using pure accuracy. The object of machine learning model is modified into the further industrial cost of prediction error, which is the cost of backup generation participation in power dispatch for power grid energy balancing. A feed-forward neural network is selected as typical machine learning model for integration. Additionally, to solve the nesting optimisation problem in network training, an equivalent model is constructed to remove the sub-optimisation and make gradient-based training optimisation feasible. A numerical study shows that the integrated structure leads to prediction results with a lower cost than those of an accuracy-based structure.(c) 2020 Published by Elsevier Ltd.
更多查看译文
关键词
Photovoltaic, Power market, Prediction, Machine learning, Nesting optimisation
AI 理解论文
溯源树
样例
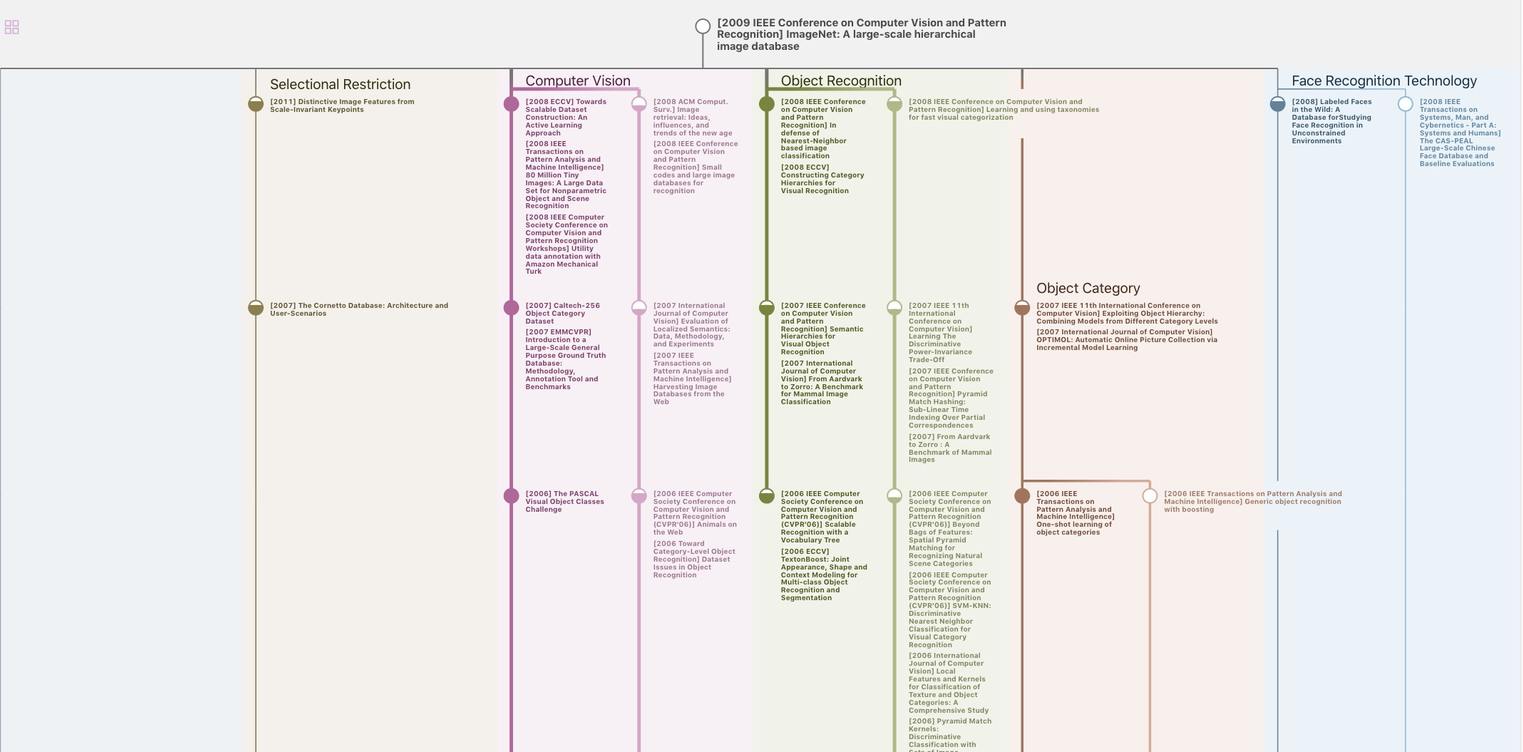
生成溯源树,研究论文发展脉络
Chat Paper
正在生成论文摘要