On The Improvement Of Representative Demand Curves Via A Hierarchical Agglomerative Clustering For Power Transmission Network Investment
ENERGY(2021)
摘要
This paper introduces an optimal clustering-based strategy to gain representative demand curves from hourly demand data that allow determining the power transmission network investment by solving the transmission expansion planning (TEP) problem. The proposed approach also provides a high dimensionality data optimal reduction for the representative demand curves that feed the TEP problem. The key idea behind this strategy is to extract demand patterns from the electric power system demand data through the implementation of a hierarchical agglomerative clustering algorithm (HACA) based on the Elbow's rule and a linkage criterion, such as Ward's variance. Then, a 24-h demand pattern is provided by following three different grouping strategies: seasonal, monthly, and weekly. As a second stage, this strategy includes the TEP formulation together with the transmission losses' linearised model aiming to test the representative demand curves achieved by HACA. To illustrate the efficiency, application, and superior functionality of the proposal, this is implemented over the IEEE 118-node network under several case studies. To determine the most appropriate approach, the results are compared with the well-known K-means method.
更多查看译文
关键词
Transmission expansion planning, Hierarchical agglomerative clustering, Elbow rule, Linkage criterion, High-dimensionality data, K-means
AI 理解论文
溯源树
样例
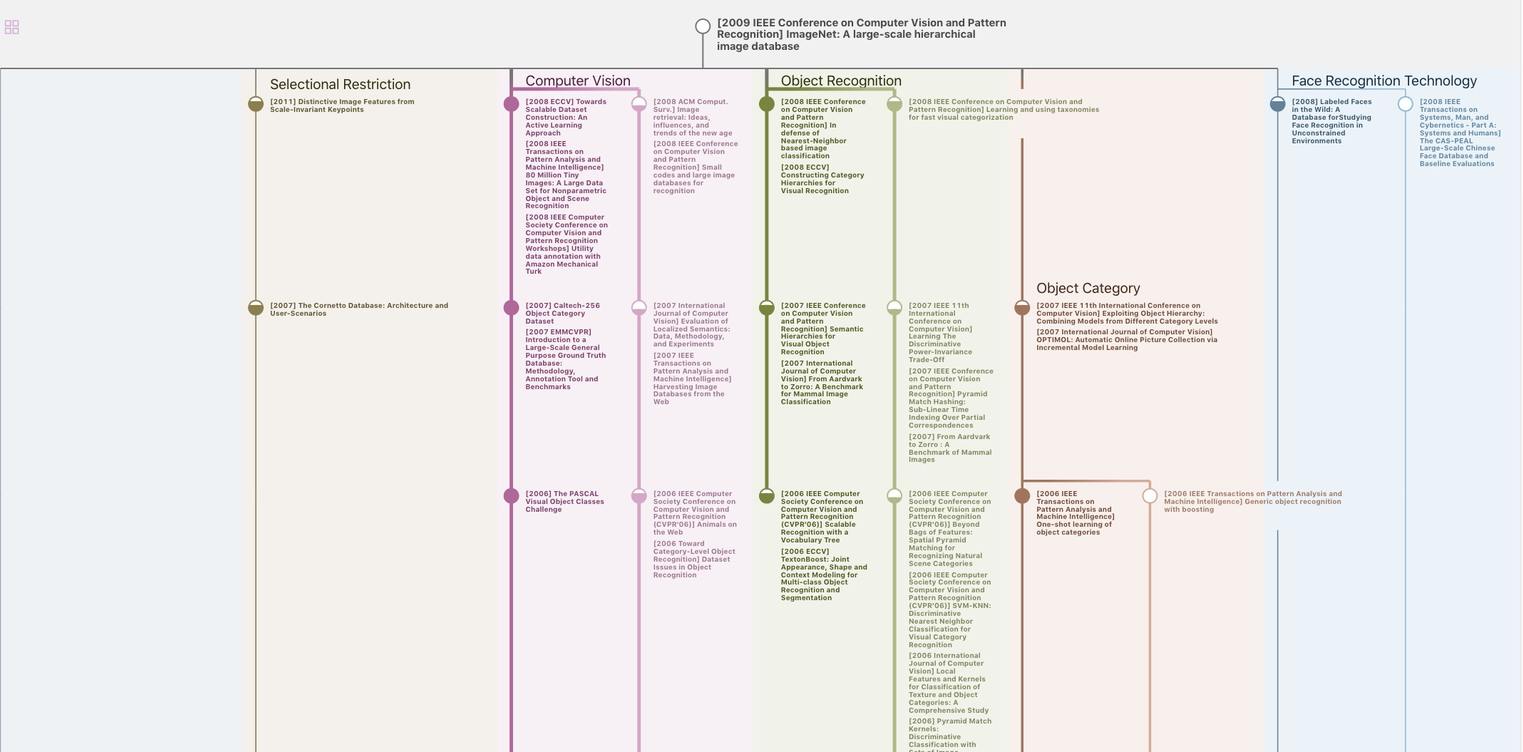
生成溯源树,研究论文发展脉络
Chat Paper
正在生成论文摘要