A novel transfer learning approach for the classification of histological images of colorectal cancer
The Journal of Supercomputing(2021)
Abstract
Colorectal cancer (CRC) is the second most diagnosed cancer in the United States. It is identified by histopathological evaluations of microscopic images of the cancerous region, relying on a subjective interpretation. The Colorectal Histology dataset used in this study contains 5000 images, made available by the University Medical Center Mannheim. This approach proposes the automatic identification of eight types of tissues found in CRC histopathological evaluation. We apply Transfer Learning from architectures of Convolutional Neural Networks (CNNs). We modify the structures of CNNs to extract features from the images and input them to well-known machine learning methods: Naive Bayes, Multilayer Perceptron, k-Nearest Neighbors, Random Forest, and Support Vector Machine (SVM). We evaluated 108 extractor–classifier combinations. The one that achieved the best results is DenseNet169 with SVM (RBF), reaching an Accuracy of 92.083% and F1-Score of 92.117%. Therefore, our approach is capable of distinguishing tissues found in CRC histopathological evaluation.
MoreTranslated text
Key words
Transfer learning,Colorectal cancer,Histological images,Convolutional neural networks
AI Read Science
Must-Reading Tree
Example
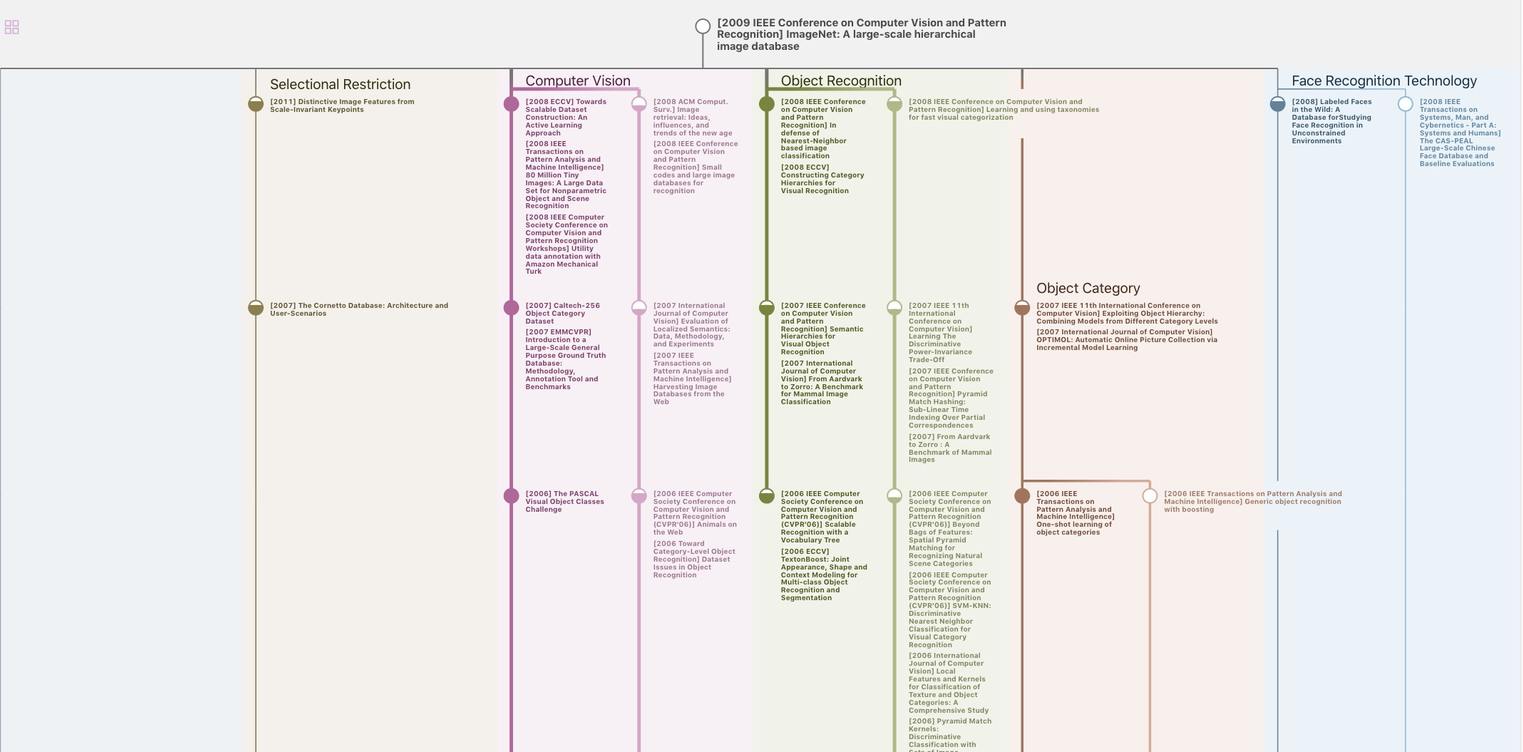
Generate MRT to find the research sequence of this paper
Chat Paper
Summary is being generated by the instructions you defined