Site-specific automated contouring model generalisibiliy enhancement
European Journal of Echocardiography(2021)
摘要
Abstract Funding Acknowledgements Type of funding sources: None. Background Segmentation of cardiac structures in echocardiography is a pre-requisite for accurately assessing cardiac morphology and function. Manual or semi-automated segmentation are both routinely used in clinical practice, although these can be time-consuming, and can introduce high inter- and intra- operator variability resulting in decreased reproducibility. Effective contouring with no manual input has proven to be challenging due to variations in image quality, image noise, motion during the acquisition and the lack of a well-defined geometry. Methods This work proposes a coordinate regression method for automated left ventricle (LV) segmentation, presented in Figure 1 (a). The proposed method is based on a modified U-net architecture that outputs the likelihood of coordinates of landmark points. The obtained likelihood heatmaps are converted to 2D coordinates using a differentiable spatial to numerical transform. The model was trained and validated on UK multisite data (1383 subjects) comprising apical 2 and 4 chamber views for both contrast and non-contrast echocardiographic images. The Cardiac Acquisitions for Multi-structure Ultrasound Segmentation (CAMUS) echocardiographic image segmentation database was used to assess the performance of the proposed method acting as data from a new clinical site. The CAMUS dataset comprises apical 2 and 4 chamber views acquired from 500 patients with manually annotated cardiac structures for end-diastole and end-systole frames. The original CAMUS dataset was split into training (450 patients) and testing (50 patients), with manual contours being available only for the training dataset. Therefore, we used the CAMUS training dataset to both test and improve our model, by using a random sample of 100 studies as an independent testing dataset and the remaining 350 studies were used for retraining the initial model to improve performance for this dataset. Results The results obtained on the testing images are presented in Figure 1 (b). When the model was trained using no CAMUS data for the LV segmentation, a mean Dice coefficient of 0.890 and a median of 0.911 was obtained. Including 350 studies with the original 1383 UK dataset and retraining the same model improved the average Dice coefficient to 0.930 and the median to 0.939. The CAMUS dataset authors reported the best average Dice coefficient of 0.924 on the 50 CAMUS testing images, therefore the proposed points regression method introduces a promising alternative to mask-based segmentation models. Conclusions In conclusion, the auto-contouring framework has proven to be effective in terms of its performance and ability to generalise to new data. Furthermore, this work highlights the importance of both evaluating model performance on data from new clinical sites and also enhancing model performance. Abstract Figure.
更多查看译文
AI 理解论文
溯源树
样例
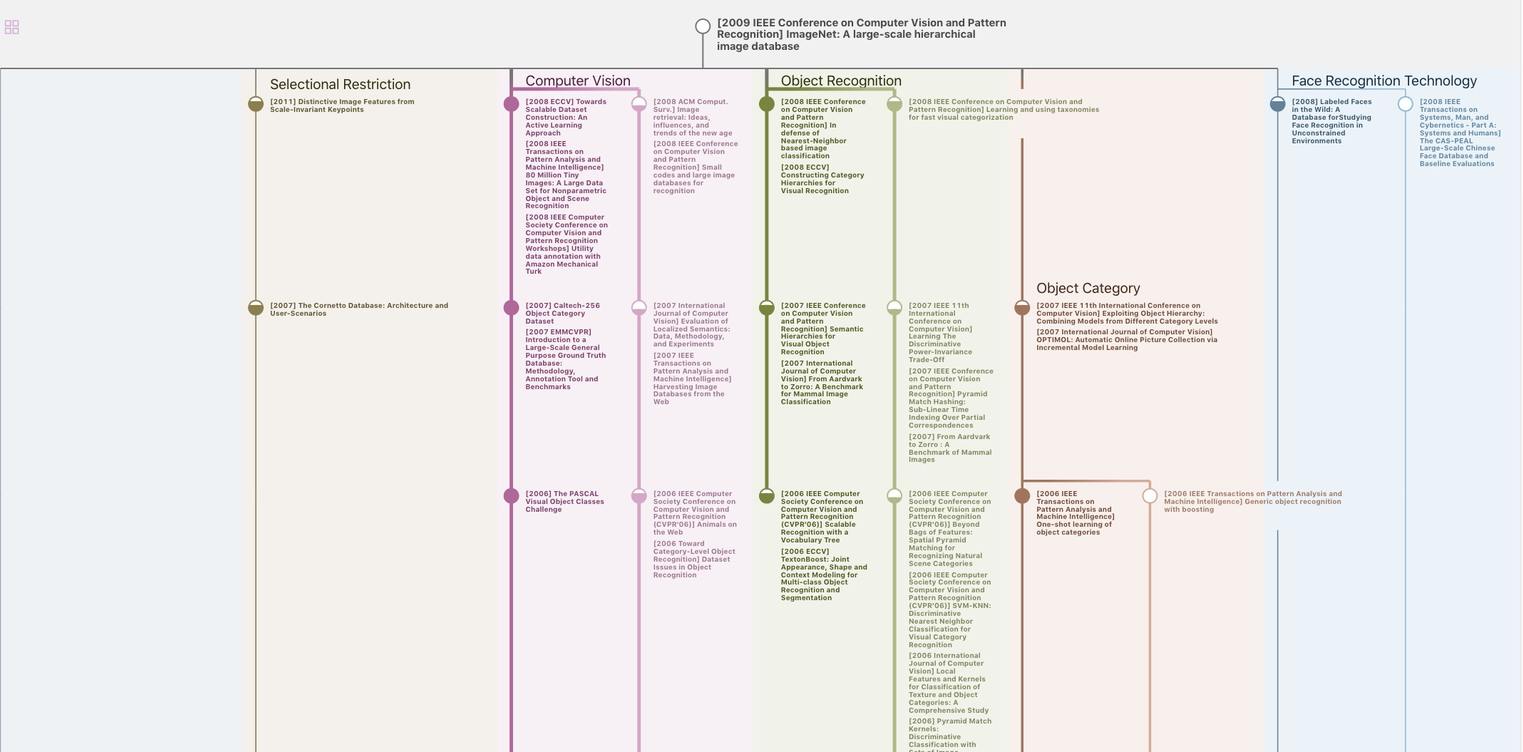
生成溯源树,研究论文发展脉络
Chat Paper
正在生成论文摘要