Deep Learning Based Data-Adaptive Descriptor For Non-Rigid Multi-Modal Medical Image Registration
SIGNAL PROCESSING(2021)
摘要
Non-rigid multi-modal medical image registration is a challenging task in the field of medical image processing and analysis because of the unpredictable complicated deformations and the nonfunctional intensity relation between images. The structural representation based registration (SRR) method can address these factors to some extent by transforming this challenging problem into the mono-modal image registration. However, the existing SSR algorithms generally rely on handcrafted features for structural representation, which tend to lead to inaccurate registered results. To address this problem, this paper has proposed a novel Laplacian Eigenmaps based deep learning network for 2D medical image registration. The proposed network is firstly used to extract the intrinsic features of different modal medical images. Then the self-similarity of extracted features is explored to construct the learning based dataadaptive descriptor (LDAD) for structural representations. The sum of squared differences (SSD) between the structural representations of the reference and floating images is used as the similarity metric to construct the objective function for image registration. The experimental results show that the LDAD based registration method can produce visually better registered results and provide higher registration accuracy for different test images than several state-of-the-art registration methods in terms of target registration error. (C) 2021 Elsevier B.V. All rights reserved.
更多查看译文
关键词
Non-rigid multi-modal registration, Deep learning, Structural representation, Similarity metric, Target registration error
AI 理解论文
溯源树
样例
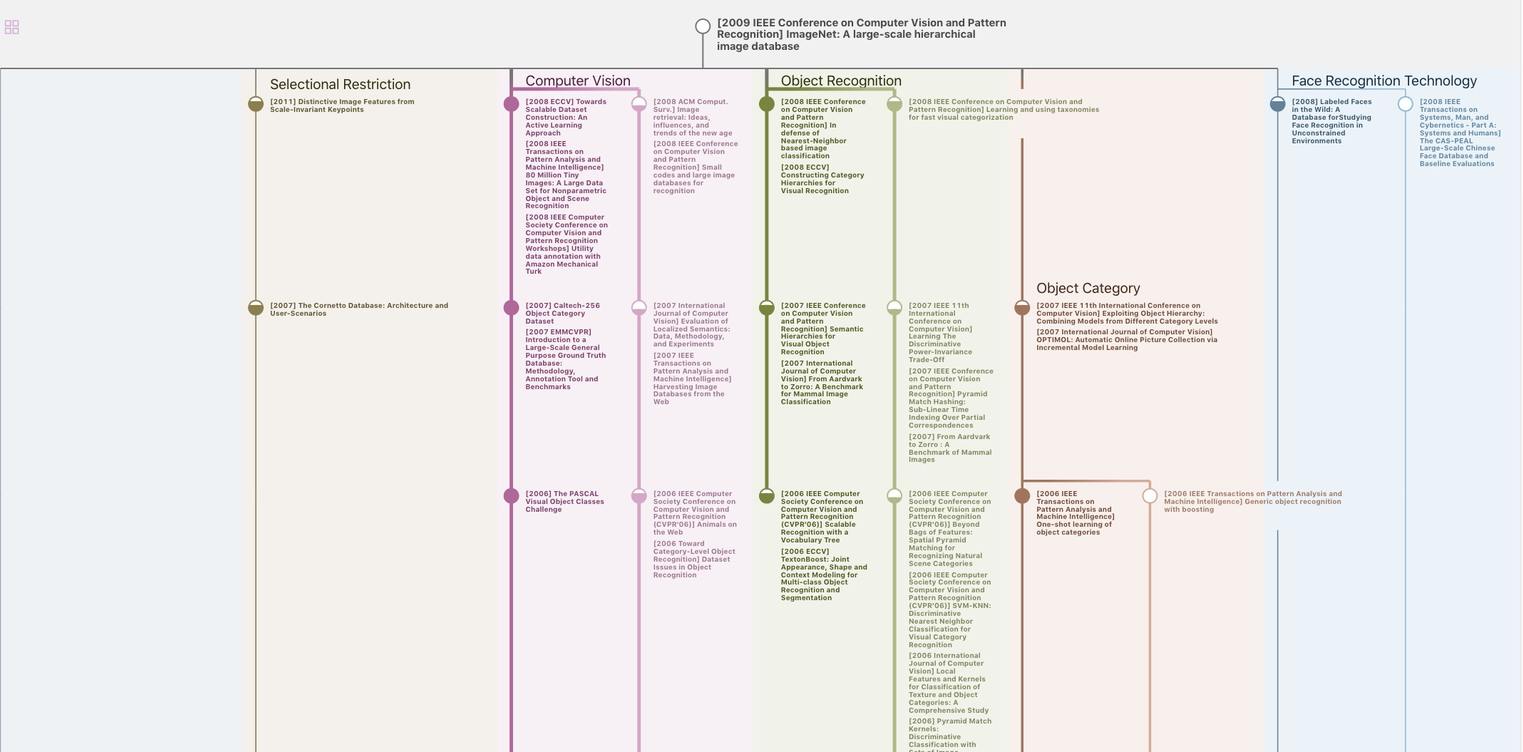
生成溯源树,研究论文发展脉络
Chat Paper
正在生成论文摘要