Data-Driven Multiobjective Predictive Optimal Control of Refining Process With Non-Gaussian Stochastic Distribution Dynamics
IEEE Transactions on Industrial Informatics(2021)
摘要
The fiber length and the Canadian standard freeness (CSF) are two key indices in measuring pulp quality of the refining process with non-Gaussian stochastic distribution dynamics. Among them, it is defective to use the conventional 1-D average fiber length (AFL) as a pulp quality index because the AFL is insufficient to describe the 2-D probability density function (pdf) shaping of fiber length distribution (FLD) with non-Gaussian types. In this article, a data-driven multiobjective predictive optimal control method is proposed to control the 2-D pdf shaping of FLD and the 1-D CSF, simultaneously. First, a radial basis function neural network (RBF-NN) based stochastic distribution model is developed to approximate the 2-D pdf shaping of FLD, and the parameters of RBF basis functions are updated by an iterative learning rule. Then, taking the developed pulp quality models, including the 2-D pdf model of FLD and the model of 1-D CSF as two predictors, a multiobjective predictive controller is designed by solving the nonlinear programming problems with constraints. Then, the stability of the resulted closed-loop system is also analyzed. Ultimately, the industrial experiments demonstrate the effectiveness of the proposed method.
更多查看译文
关键词
Fiber length distribution (FLD),multiobjective predictive control,probability density function (pdf),refining process,stochastic distribution control (SDC)
AI 理解论文
溯源树
样例
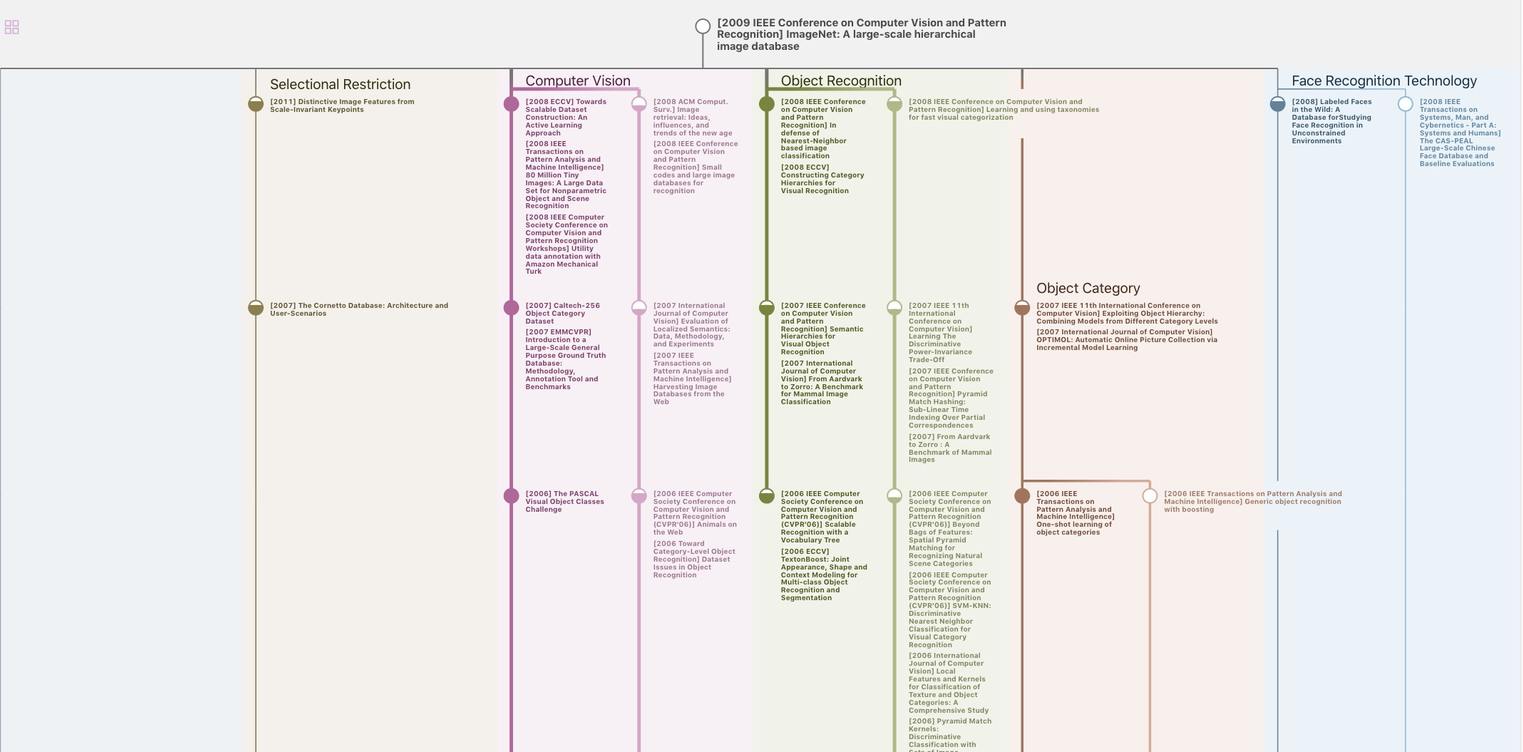
生成溯源树,研究论文发展脉络
Chat Paper
正在生成论文摘要