Relation-aware Siamese region proposal network for visual object tracking
MULTIMEDIA TOOLS AND APPLICATIONS(2021)
摘要
The backbone networks used in Siamese trackers are relatively shallow, such as AlexNet and VGGNet, resulting in insufficient features for tracking task. Therefore, this paper focuses on extracting more discriminative features to improve the performance of Siamese trackers. By comprehensive experimental validations, this goal is achieved through a simple yet effective framework referred as relation-aware Siamese region proposal network (Ra-SiamRPN). Firstly, the deep backbone network ResNet-50 is adopted to extract both low-level detail features and high-level semantic features of an image. Then we propose the feature fusion module (FFM), which can combine low-level detail features with high-level semantic features effectively. Furthermore, we propose the relation reasoning module (RRM) to perform the global relation reasoning in multiple disjoint regions. RRM can generate discriminative information to enhance the features generated by ResNet-50. Extensive experiments are conducted on the dataset OTB2015, VOT2016, VOT2018, UAV123 and LaSOT. The experiment results indicate that Ra-SiamRPN achieves competitive performance with the current advanced algorithms and shows good real-time performance. To be highlighted, in the experiments conducted on the large-scale dataset LaSOT, the success score and the normalized precision score of Ra-SiamRPN are 0.495 and 0.576, respectively. These performance indexes are better than the second best tracker MDNet 24.7% and 25.2%.
更多查看译文
关键词
Object tracking, Siamese network, Graph convolutional network, Deep learning
AI 理解论文
溯源树
样例
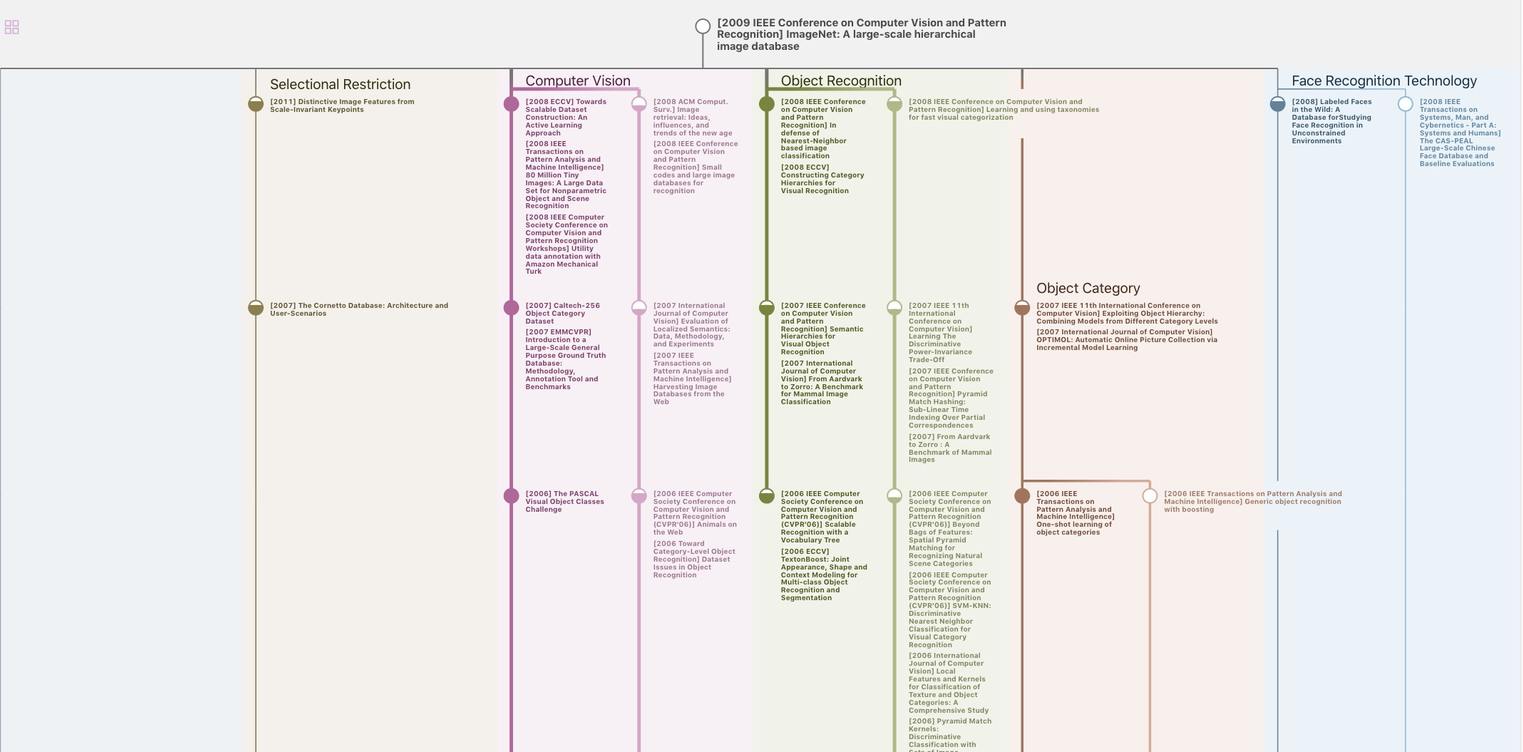
生成溯源树,研究论文发展脉络
Chat Paper
正在生成论文摘要