Exploration of optimal microstructure and mechanical properties in continuous microstructure space using a variational autoencoder
Materials & Design(2021)
摘要
Data-driven approaches enable a deep understanding of microstructure and mechanical properties of materials and greatly promote one's capability in designing new advanced materials. Deep learning-based image processing outperforms conventional image processing techniques with unsupervised learning. This study employs a variational autoencoder (VAE) to generate a continuous microstructure space based on synthetic microstructural images. The structure-property relationships are explored using a computational approach with microstructure quantification, dimensionality reduction, and finite element method (FEM) simulations. The FEM of representative volume element (RVE) with a microstructure-based constitutive model model is proposed for predicting the overall stress-strain behavior of the investigated dual-phase steels. Then, Gaussian process regression (GPR) is used to make connections between the latent space point and the ferrite grain size as inputs and mechanical properties as outputs. The GPR with VAE successfully predicts the newly generated microstructures with target mechanical properties with high accuracy. This work demonstrates that a variety of microstructures can be candidates for designing the optimal material with target properties in a continuous manner.
更多查看译文
关键词
Microstructure-based modeling,Deep learning,Variational autoencoder,Gaussian process regression,Dual-phase steel
AI 理解论文
溯源树
样例
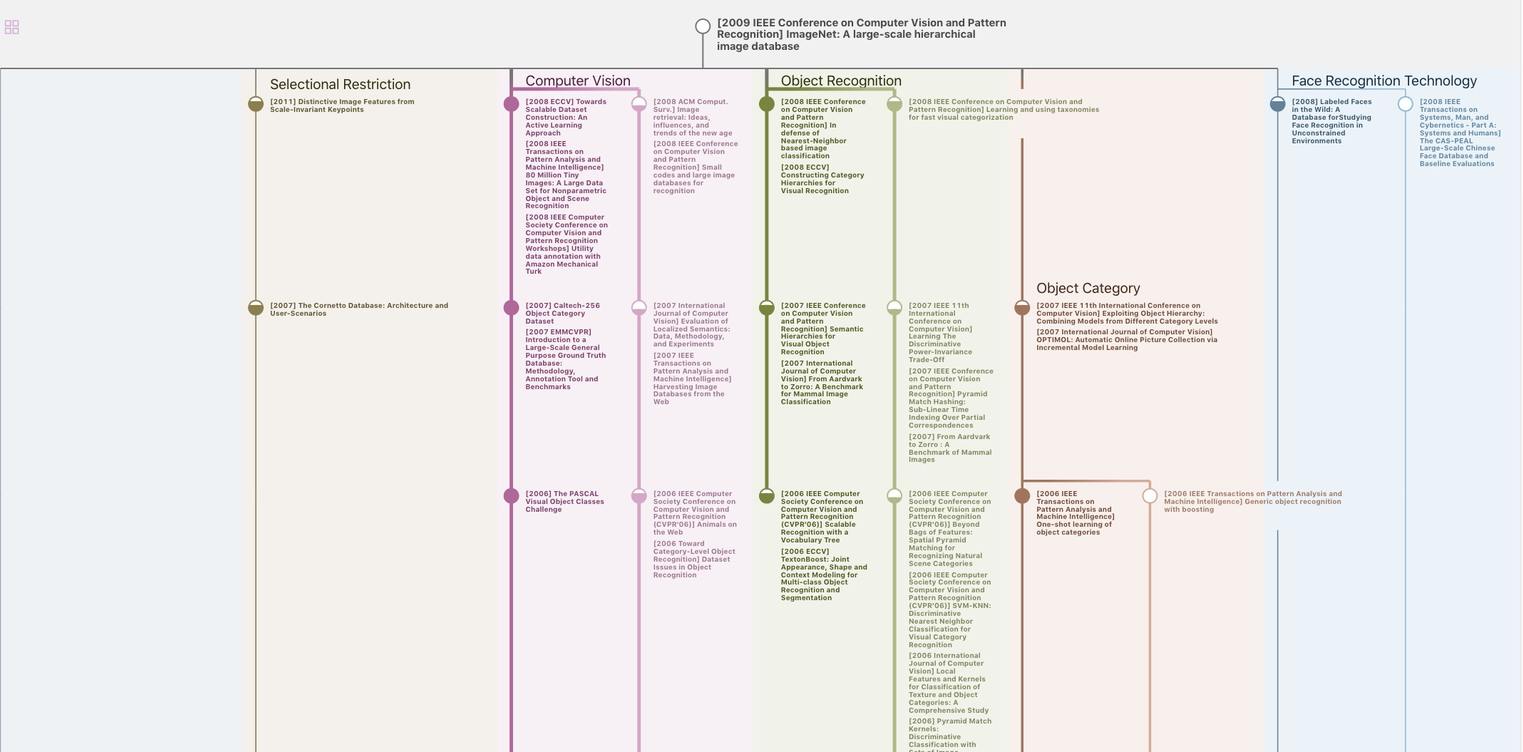
生成溯源树,研究论文发展脉络
Chat Paper
正在生成论文摘要