Rcag-Net: Residual Channelwise Attention Gate Network For Hot Spot Defect Detection Of Photovoltaic Farms
IEEE TRANSACTIONS ON INSTRUMENTATION AND MEASUREMENT(2021)
摘要
The small hot spot defect detection for photovoltaic (PV) farms is a challenging problem due to the feature vanishing as the network deepens. To solve this challenging problem, a novel residual channelwise attention gate network (RCAG-Net) is proposed by employing a novel RCAG module to achieve multiscale feature fusion, complex background suppression, and defect feature highlighting. In RCAG-Net, the novel RCAG module first realizes feature fusion by adding the features of different scale layers. Next, global average pooling (GAP) and multilayer perceptron (MLP) are used to dimension reduction and refinement of the fused features, then yielding an attention map for channelwise feature reweighting by gate mechanism, which employs selective transmission of the convolution neural network (CNN)-extracted features to achieve informative feature filtering. Moreover, residual connection from the fused features to the final output facilitates the insertion of the new RCAG into some classical pretrained models, without breaking its initial behavior. Finally, the proposed approach is validated through a real defect detection system, and the experimental result clearly verifies its effectiveness for small hot spot detection of PV farms.
更多查看译文
关键词
Attention network, defect detection, photovoltaic (PV) farms, unmanned aerial vehicle
AI 理解论文
溯源树
样例
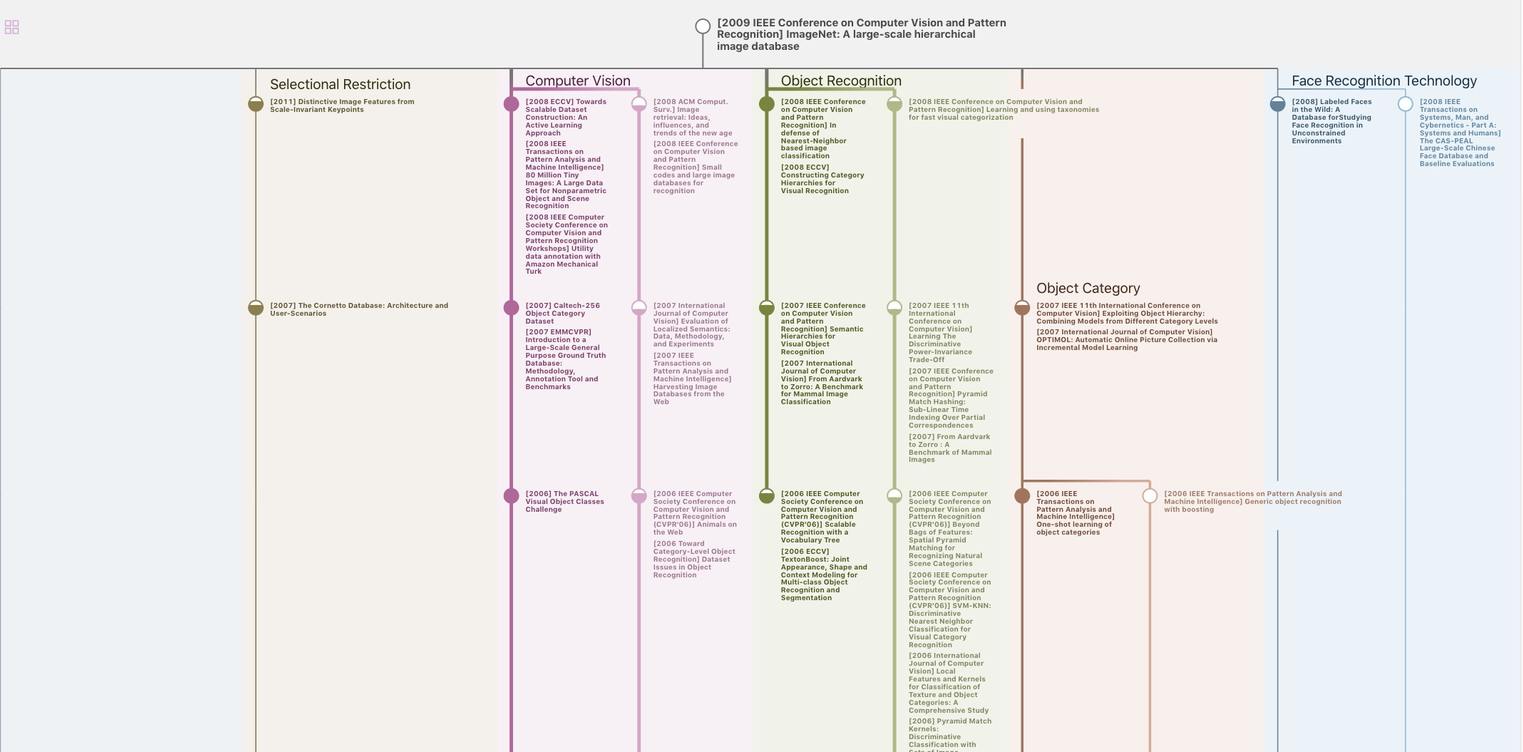
生成溯源树,研究论文发展脉络
Chat Paper
正在生成论文摘要