A deep learning-based and fully automated pipeline for thoracic aorta geometric analysis and TEVAR planning from computed tomography
European Journal of Echocardiography(2021)
摘要
Abstract Funding Acknowledgements Type of funding sources: Public grant(s) – National budget only. Main funding source(s): Ministry of Publich Health - Ricerca Corrente Introduction Thoracic endovascular aortic repair (TEVAR) represents a well-established alternative to open repair in selected patients. Its preoperative feasibility assessment and planning requires a computational tomography (CT)-based analysis of the geometric aortic features to identify an adequate proximal and distal landing zone (LZ) for endograft deployment. Yet, controversies persist on the definition and methods of measurement of specific geometric features of the LZs, including angulation and tortuosity, which are associated with an increased risk of postoperative endograft failure. In this respect, the development of a preoperative image processing method that provides an automatic and highly reproducible 3D identification of critical geometric features and specific anatomical landmarks, thus reducing the time and uncertainties related to manual segmentation, remains a largely unmet clinical need. In this study, we developed and applied a fully automated pipeline embedding a convolutional neural network (CNN), which feeds on 3D CT images to automatically segment the thoracic aorta, recognize the relevant anatomical landmarks and LZs, and quantifies the geometry of the aortic arch in each proximal LZ s (i.e. 0 to 3). Methods Ninety CT scans of healthy aortas were retrieved, being the study conceived as a proof of concept analysis. The thoracic aorta was manually segmented by five independent and expert operators. 72 scans with the corresponding ground truth segmentations were randomly selected and used to train the CNN, which was based on a 3D U-Net architecture. The other 18 scans were used to test the CNN-based segmentations. The fully automated pipeline was obtained by integrating the CNN, 3D geometry skeletonization, and processing of the aortic centerline and wall via computational geometry (Figure). The resulting metrics included aortic arch centerline radius of curvature, proximal landing zones (PLZs) maximum diameters, angulation and tortuosity calculated according to previously published work. These parameters were statistically analyzed to compare standard arches vs. arches with a common origin of the innominate and left carotid artery (CILCA), and the different landing zones in each arch type. Results The CNN segmentation yielded a mean Dice score of 0.94 with respect to manual ground truth segmentations. Standard arches were characterized by significantly larger radius of curvature (p = 0.002) and lower tortuosity in zone 3 (p = 0.004) vs. CILCA arches. For both standard and CILCA arches, comparisons among PLZs revealed statistically significant differences in maximum zone diameters (p < 0.0001), angulation (p < 0.0001) and tortuosity (p < 0.0001). Conclusions We developed a CNN-based automated pipeline for the automated, and reliable geometric quantification of standard and CILCA aortic arches. This tool has the potential to support TEVAR pre-procedural planning in a real clinical setting. Abstract Figure. Automatic pipeline scheme
更多查看译文
关键词
thoracic aorta,computed tomography,tevar planning,learning-based
AI 理解论文
溯源树
样例
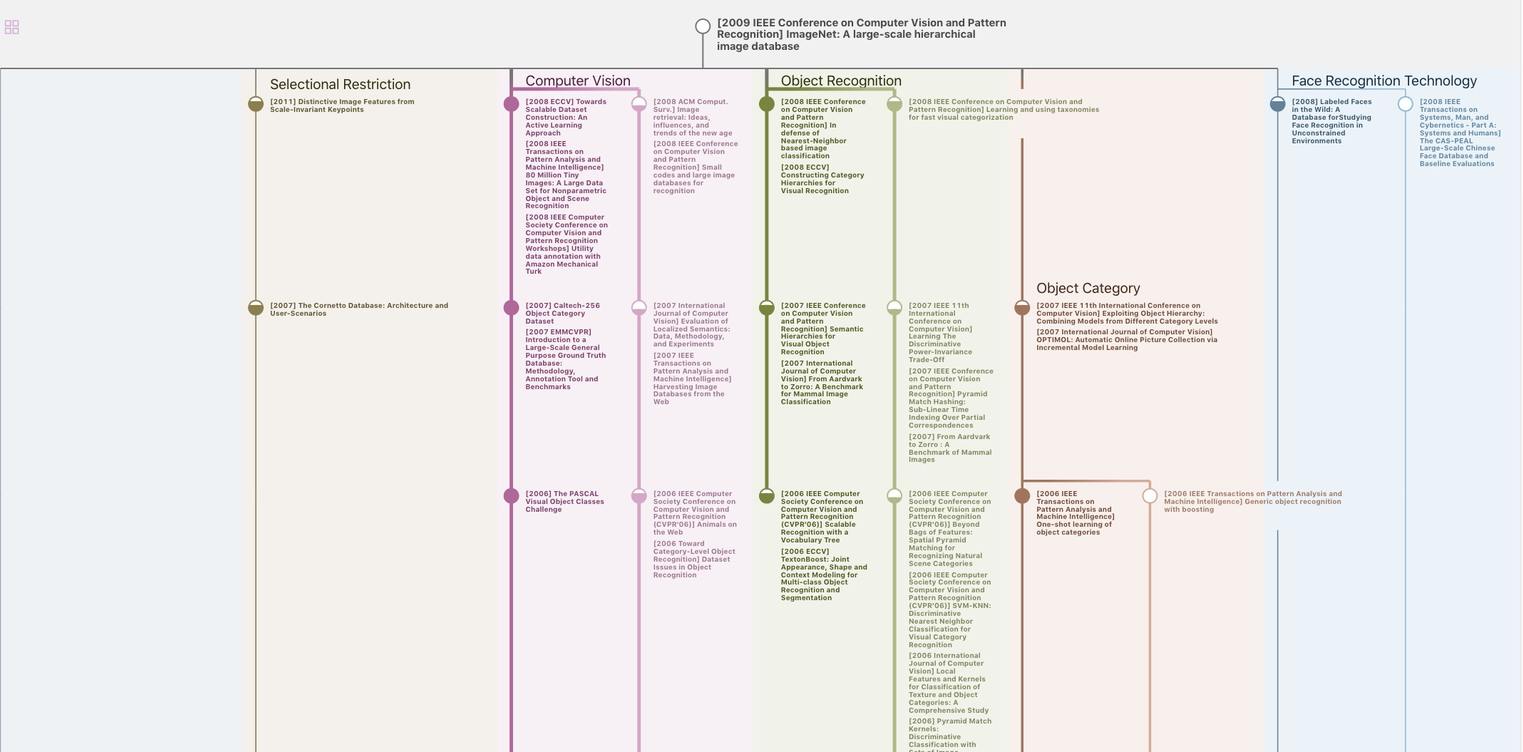
生成溯源树,研究论文发展脉络
Chat Paper
正在生成论文摘要