Anomaly Detection of Trackside Equipment based on Semi-Supervised and Multi-Domain Learning
2020 15th IEEE International Conference on Signal Processing (ICSP)(2020)
摘要
With the rapid development of rail transit, the health status of trackside equipment is more and more crucial to the operation of rail transit. The use of high-precision anomaly detection methods can quickly detect the abnormal conditions of trackside equipment, ensuring the safety of rail transit. In this paper, a new framework of trackside equipment anomaly detection based on semi-supervised and multi-domain deep learning is proposed, which effectively improves the efficiency and accuracy of anomaly detection. Considering the inconspicuous changes in trackside equipment, including screw missing and cable corrosion, it is not feasible to directly use machine learning method for anomaly detection. Therefore, we first match the collected data to get the difference image. Secondly, considering the sparsity of the difference image, the incremental principal component analysis method is used to reduce the dimension of the difference image. Finally, in view of the limited number of training samples and the high cost of manual labeling, we combine semi-supervised deep learning and multi-domain deep learning to effectively train the anomaly detection model. Experimental results show that the proposed method has higher detection accuracy than the traditional artificial anomaly detection and anomaly detection based on shallow image alignment methods.
更多查看译文
关键词
trackside equipment,image processing,semi- supervised deep learning,multi-domain deep learning,incremental principal components analysis
AI 理解论文
溯源树
样例
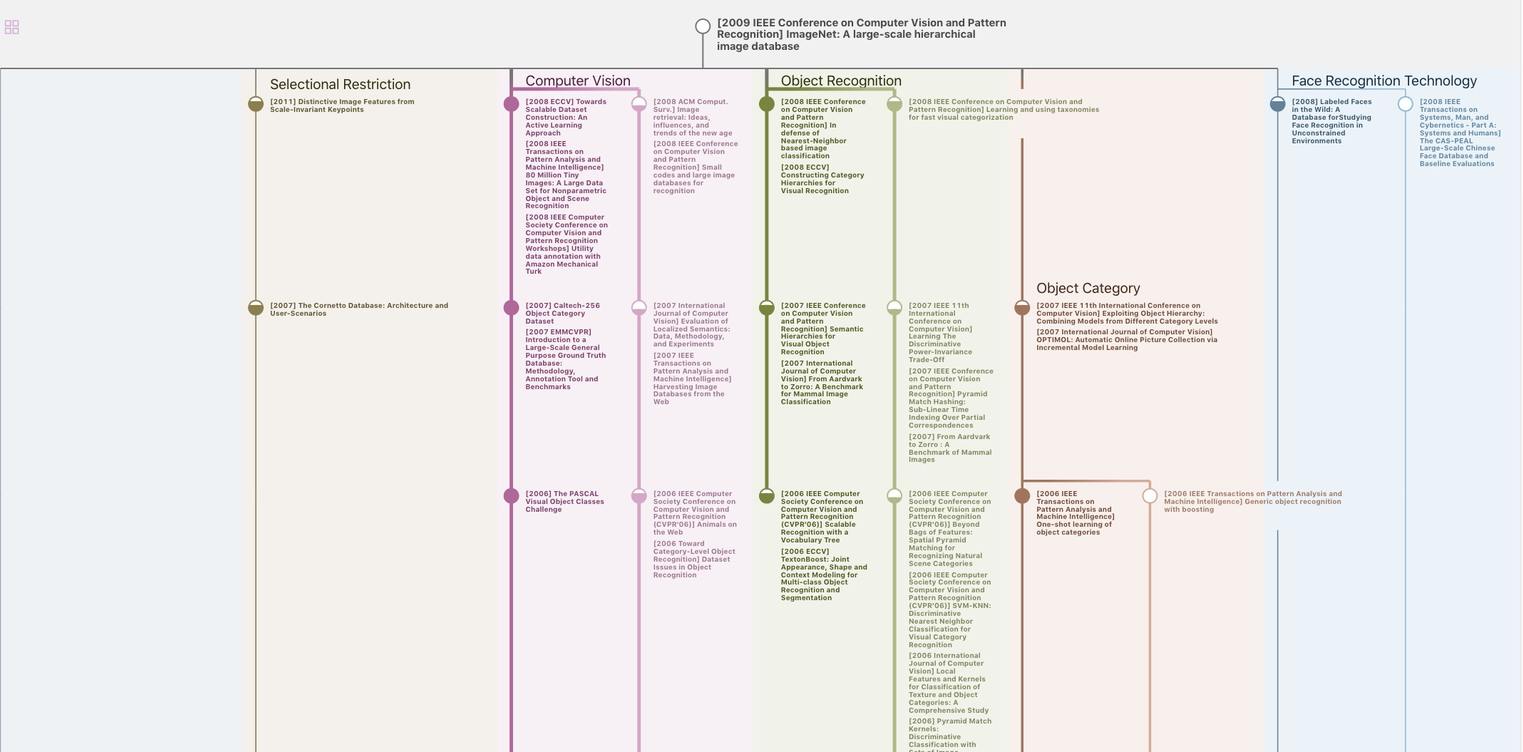
生成溯源树,研究论文发展脉络
Chat Paper
正在生成论文摘要