Beneficial Effect of Combined Replay for Continual Learning
ICAART: PROCEEDINGS OF THE 13TH INTERNATIONAL CONFERENCE ON AGENTS AND ARTIFICIAL INTELLIGENCE - VOL 2(2021)
Abstract
While deep learning has yielded remarkable results in a wide range of applications, artificial neural networks suffer from catastrophic forgetting of old knowledge as new knowledge is learned. Rehearsal methods overcome catastrophic forgetting by replaying an amount of previously learned data stored in dedicated memory buffers. Alternatively, pseudo-rehearsal methods generate pseudo-samples to emulate the previously learned data, thus alleviating the need for dedicated buffers. Unfortunately, up to now, these methods have shown limited accuracy. In this work, we combine these two approaches and employ the data stored in tiny memory buffers as seeds to enhance the pseudo-sample generation process. We then show that pseudo-rehearsal can improve performance versus rehearsal methods for small buffer sizes. This is due to an improvement in the retrieval process of previously learned information. Our combined replay approach consists of a hybrid architecture that generates pseudo-samples through a reinjection sampling procedure (i.e. iterative sampling). The generated pseudo-samples are then interlaced with the new data to acquire new knowledge without forgetting the previous one. We evaluate our method extensively on the MNIST, CIFAR-10 and CIFAR-100 image classification datasets, and present state-of-the-art performance using tiny memory buffers.
MoreTranslated text
Key words
Incremental Learning,Lifelong Learning,Continual Learning,Sequential Learning,Pseudo-rehearsal,Rehearsal
AI Read Science
Must-Reading Tree
Example
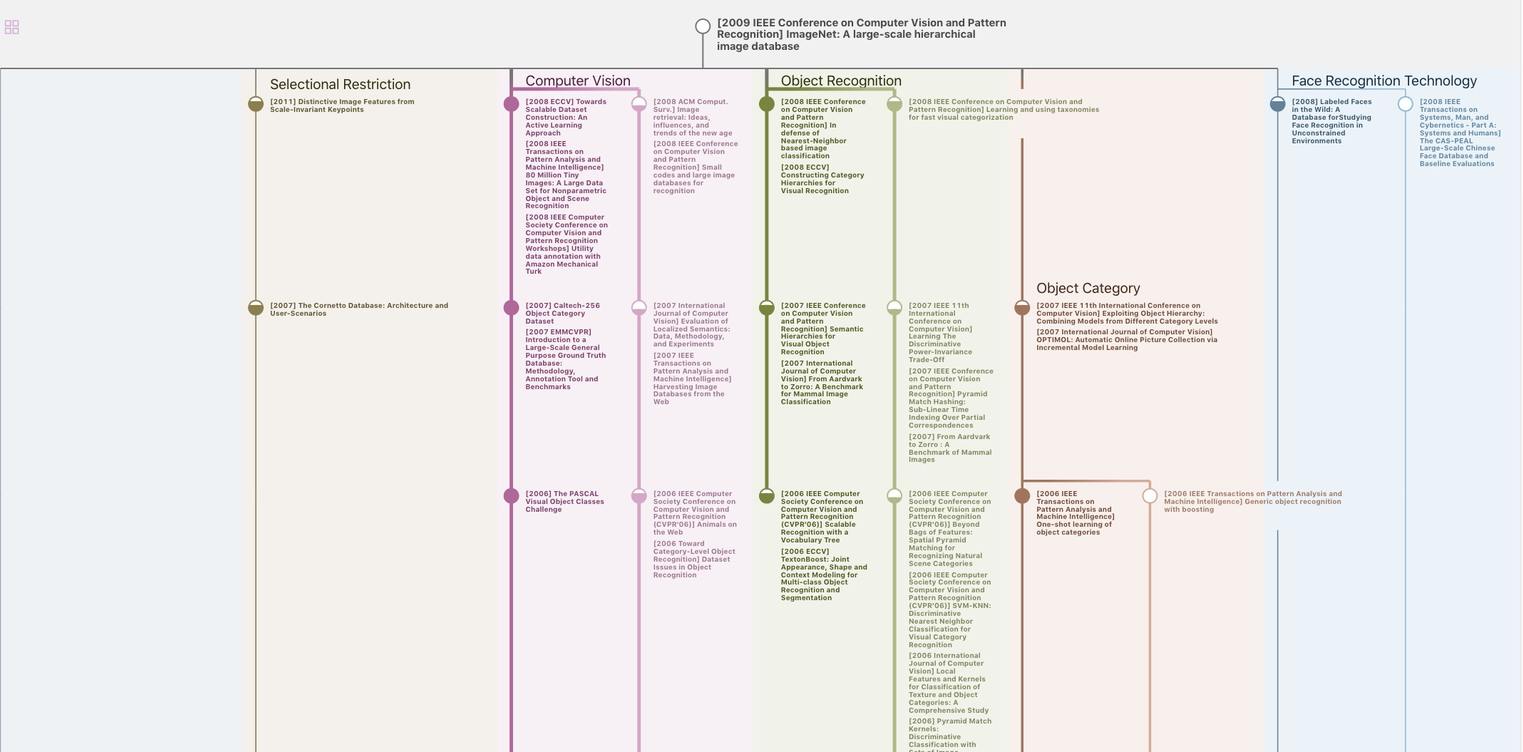
Generate MRT to find the research sequence of this paper
Chat Paper
Summary is being generated by the instructions you defined